Asymmetrical Distribution Definition And Examples In Statistics
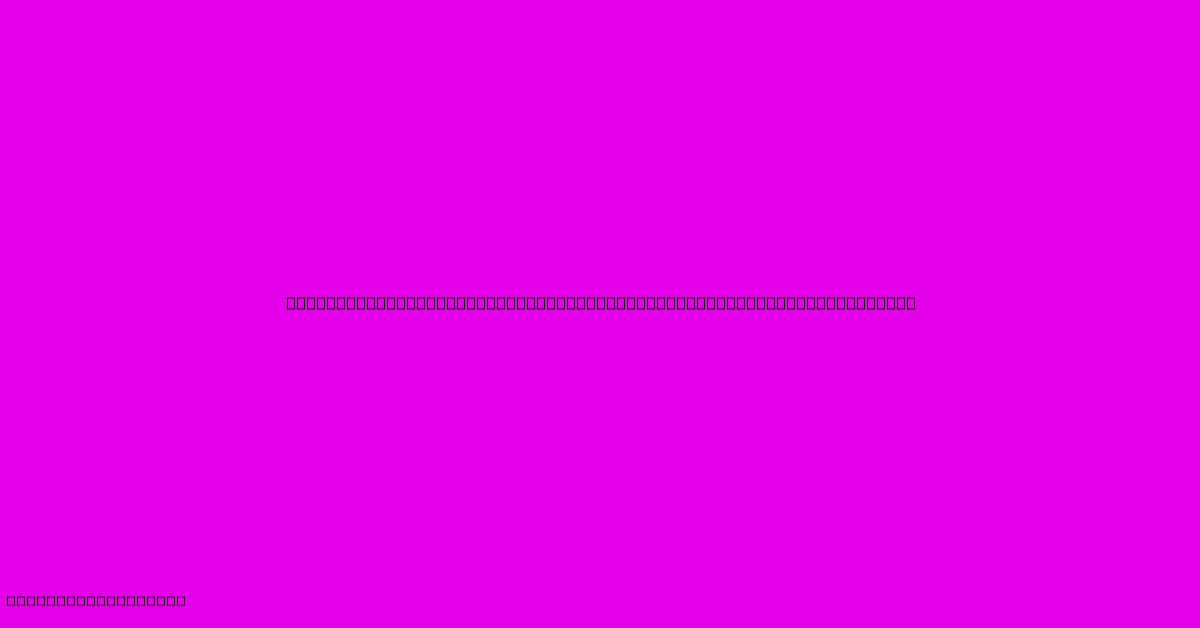
Discover more in-depth information on our site. Click the link below to dive deeper: Visit the Best Website meltwatermedia.ca. Make sure you don’t miss it!
Table of Contents
Unveiling the Secrets of Asymmetrical Distributions: A Deep Dive into Statistical Skew
What is asymmetrical distribution and why should you care? It's a fundamental concept in statistics that reveals hidden patterns in your data. Understanding it unlocks powerful insights across various fields.
Editor's Note: This comprehensive guide to asymmetrical distributions in statistics was published today. It provides a thorough exploration of the topic, complete with examples and practical applications.
Importance & Summary: Asymmetrical distributions, or skewed distributions, are crucial in data analysis because they reveal the presence of outliers, indicate potential biases in data collection, and inform the choice of appropriate statistical methods. This guide will explore the definition of asymmetrical distributions, delve into various types of skewness (positive and negative), and provide real-world examples to illustrate the concept's significance in different fields, including finance, healthcare, and social sciences. The guide also covers methods for identifying and measuring skewness.
Analysis: The information compiled in this guide is based on established statistical principles and extensive research across multiple disciplines. Numerous academic papers, textbooks, and online resources were consulted to ensure accuracy and completeness. Real-world examples were selected to represent diverse applications and illustrate the practical implications of understanding asymmetrical distributions.
Key Takeaways:
- Asymmetrical distributions deviate from a symmetrical pattern.
- Skewness measures the asymmetry of a distribution.
- Positive skew indicates a long tail to the right.
- Negative skew indicates a long tail to the left.
- Understanding skewness is crucial for choosing appropriate statistical tests.
Asymmetrical Distribution: Definition and Types
Asymmetrical distribution, also known as skewed distribution, refers to a probability distribution where the data points are not evenly distributed around the mean. Unlike a symmetrical distribution (like the normal distribution), where the mean, median, and mode are equal and the data is balanced on both sides of the central tendency, an asymmetrical distribution shows a pronounced tail extending in one direction. This tail indicates a concentration of data points on one side of the mean, while the other side has a more dispersed spread.
There are two main types of asymmetrical distributions:
-
Positive Skew (Right Skew): In a positively skewed distribution, the tail extends to the right, indicating a greater number of data points clustered towards the lower values with fewer, more extreme values at the higher end. The mean is typically greater than the median, which in turn is greater than the mode. This type of distribution frequently represents scenarios where there’s an upper bound or limit influencing the data.
-
Negative Skew (Left Skew): Conversely, a negatively skewed distribution features a tail extending to the left. This suggests a higher concentration of data points at the higher values, with fewer data points at the lower end. In this case, the mean is usually smaller than the median, which is smaller than the mode. This type often appears where there's a lower bound or limitation.
Key Aspects of Asymmetrical Distributions
Measures of Skewness
Several statistical measures quantify the degree of skewness:
- Pearson's Mode Skewness: A simple method that calculates the difference between the mean and mode, divided by the standard deviation.
- Pearson's Median Skewness: Similar to the mode skewness, but uses the median instead of the mode.
- Bowley's Skewness: Based on quartiles, providing a robust measure less sensitive to outliers.
- Moment Coefficient of Skewness: Uses the third moment about the mean to measure skewness. This is a more complex but widely used method.
These measures provide numerical values to indicate the direction and magnitude of skewness. A value of zero suggests symmetry, while positive values indicate positive skew, and negative values indicate negative skew. The magnitude of the value reflects the severity of the skewness.
Visual Identification of Skewness
Besides quantitative measures, visual inspection of histograms, box plots, and probability plots can effectively identify skewness.
- Histograms: A skewed histogram will clearly show a longer tail on one side of the central peak.
- Box Plots: A skewed box plot will have the median positioned off-center within the box, and one whisker will be significantly longer than the other.
- Probability Plots (Q-Q plots): These plots compare the quantiles of the data to the quantiles of a normal distribution. Deviations from the diagonal line indicate skewness.
Examples of Asymmetrical Distributions in Real-World Applications
Numerous real-world phenomena exhibit asymmetrical distributions:
1. Income Distribution: Income data often displays a positive skew, with a concentration of individuals earning lower incomes and a smaller number earning substantially higher incomes. The long right tail reflects the presence of high-income earners.
2. Stock Market Returns: Stock market returns often show a positive skew. While most returns might be relatively small or negative, large positive returns occur less frequently, pulling the mean higher than the median.
3. Healthcare Data: Survival times after a diagnosis of a serious illness often present a positive skew. The majority of patients may experience shorter survival times, while a few experience much longer survival, creating a right-leaning tail.
4. Test Scores: Test scores can exhibit both positive and negative skew depending on the difficulty of the test and the distribution of student abilities. A very easy test might show negative skew (most scores clustered at the high end), while a very difficult test could show positive skew.
5. Real Estate Prices: Real estate prices often demonstrate a positive skew due to the presence of a few exceptionally high-priced properties in comparison to a large number of more moderately priced homes.
Implications of Skewness in Statistical Analysis
The presence of skewness significantly impacts the choice of appropriate statistical methods. Many standard statistical procedures assume normality (symmetry) in the data. When data exhibits significant skewness, these procedures may yield inaccurate or misleading results. Transformations such as logarithmic or square root transformations can often address skewness by making the distribution more symmetrical. Non-parametric tests, which do not assume normality, are also viable alternatives when dealing with heavily skewed data.
FAQ
FAQ: Understanding Asymmetrical Distributions
Q1: What is the difference between a symmetrical and asymmetrical distribution? A1: A symmetrical distribution is balanced around its mean, with data points evenly distributed on both sides. An asymmetrical distribution is unbalanced, with a longer tail extending in one direction.
Q2: How is skewness measured? A2: Skewness is typically measured using various statistical measures, including Pearson's mode and median skewness, Bowley's skewness, and the moment coefficient of skewness.
Q3: What does positive skewness imply? A3: Positive skewness (right skew) implies a longer tail extending to the right, suggesting a concentration of data points toward lower values and a few outliers at higher values.
Q4: What does negative skewness imply? A4: Negative skewness (left skew) indicates a longer tail extending to the left, signifying a concentration of data points toward higher values and a few outliers at lower values.
Q5: How can I visualize skewness in my data? A5: Histograms, box plots, and probability plots are visual tools to identify the presence and direction of skewness in data.
Q6: Why is understanding skewness important? A6: Understanding skewness is crucial for selecting appropriate statistical methods and interpreting results correctly. Many standard statistical tests assume normality (no skewness) and may produce inaccurate outcomes if this assumption is violated.
Tips for Working with Asymmetrically Distributed Data
- Visualize your data: Always begin with visual inspection using histograms, box plots, or other graphical representations to identify skewness.
- Calculate skewness measures: Quantify the degree of skewness using appropriate statistical measures.
- Consider data transformations: If skewness is substantial, explore data transformations (logarithmic, square root, etc.) to make the distribution more symmetrical.
- Use appropriate statistical tests: Choose statistical tests that are robust to violations of normality assumptions or use non-parametric methods designed for non-normal data.
- Interpret results carefully: Consider the impact of skewness on your statistical conclusions and be cautious when drawing inferences.
- Explore the underlying reasons: Investigate the cause of the skewness, as it may provide insights into the underlying process generating the data.
Summary
This guide has provided a detailed exploration of asymmetrical distributions in statistics, covering their definition, types, measures, and implications. Understanding and addressing skewness is essential for accurate data analysis and informed decision-making. By applying the methods and insights discussed in this guide, researchers and analysts can more effectively interpret data and draw reliable conclusions across diverse fields.
Closing Message
Asymmetrical distributions are ubiquitous in real-world data. Mastering the principles outlined herein empowers better data interpretation, leading to more accurate and insightful analyses across numerous disciplines. Further investigation into advanced statistical techniques for handling skewed data will solidify this foundation and unlock even deeper analytical capabilities.
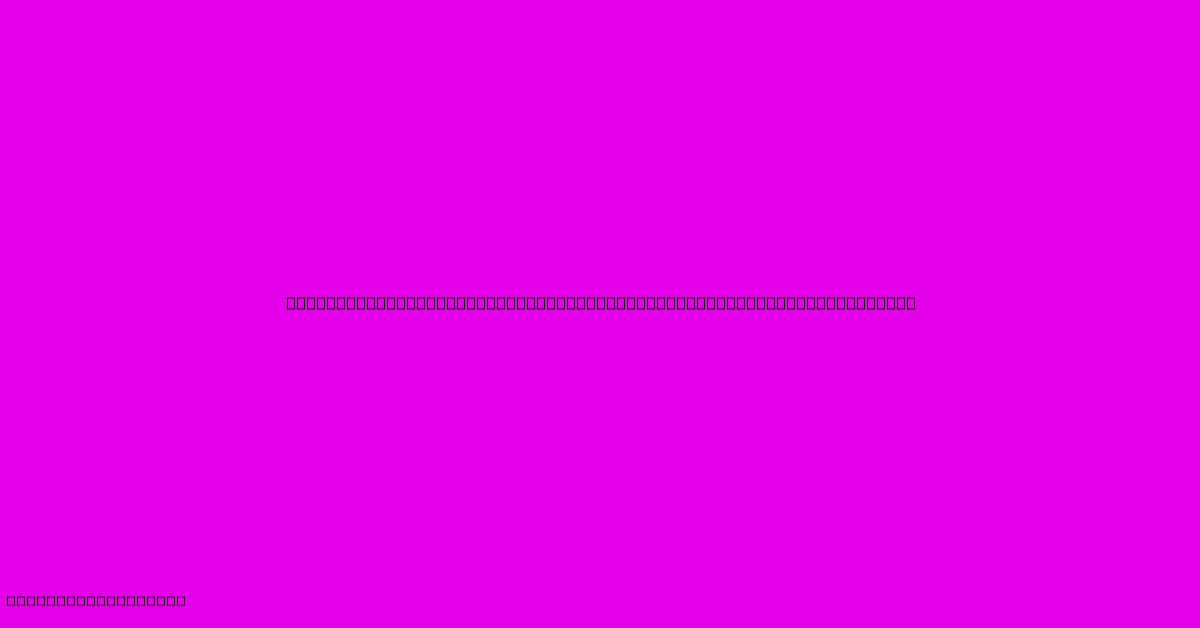
Thank you for taking the time to explore our website Asymmetrical Distribution Definition And Examples In Statistics. We hope you find the information useful. Feel free to contact us for any questions, and don’t forget to bookmark us for future visits!
We truly appreciate your visit to explore more about Asymmetrical Distribution Definition And Examples In Statistics. Let us know if you need further assistance. Be sure to bookmark this site and visit us again soon!
Featured Posts
-
What Happens If You Dont Report Cryptocurrency On Taxes
Jan 10, 2025
-
Regulation T Reg T Definition Of Requirement And Example
Jan 10, 2025
-
What Annual Percentage Do Pension Funds Pay Per Year In Fees
Jan 10, 2025
-
Allied Lines Definition
Jan 10, 2025
-
Return On Average Equity Roae Definition And What It Indicates
Jan 10, 2025