Attribute Sampling Definition Purpose And How It Works
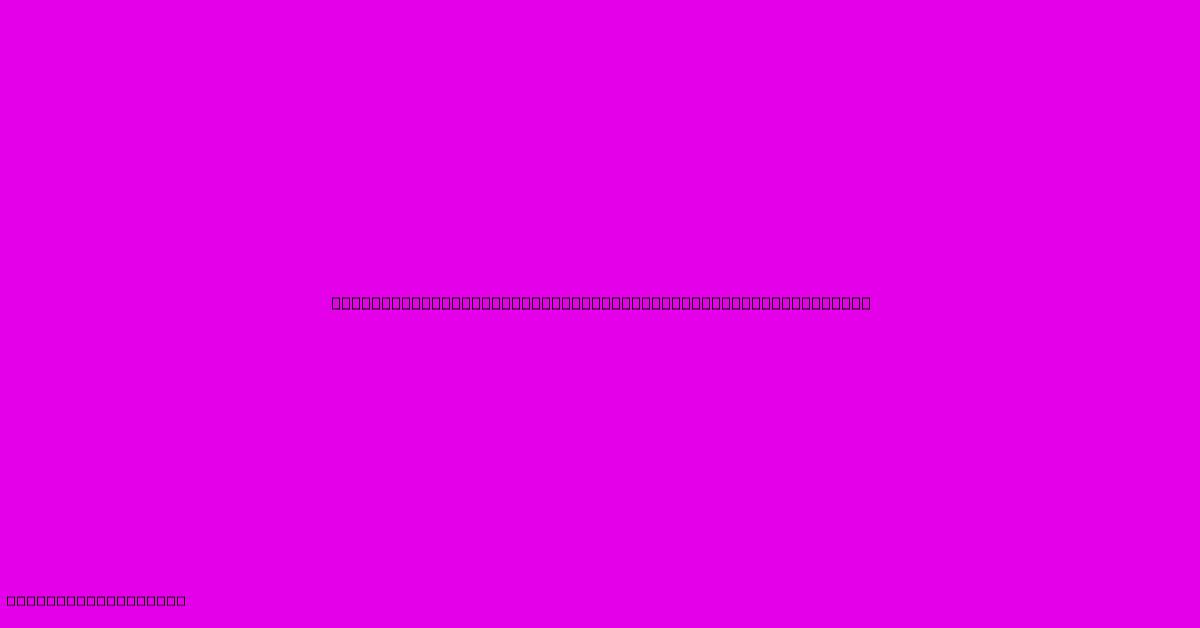
Discover more in-depth information on our site. Click the link below to dive deeper: Visit the Best Website meltwatermedia.ca. Make sure you don’t miss it!
Table of Contents
Unveiling Attribute Sampling: Purpose, Definition, and Mechanics
Do you know how to effectively assess the quality of a large population without examining every single member? Attribute sampling offers a powerful solution. This technique is crucial for various fields, ensuring efficient and accurate assessments.
Editor's Note: This comprehensive guide on attribute sampling has been published today, providing a detailed exploration of its definition, purpose, and mechanics.
Importance & Summary: Attribute sampling is a statistical method used to estimate the proportion of a population possessing a specific characteristic (attribute). It's vital for cost-effective quality control, auditing, and research, offering insights without exhaustive data collection. This guide provides a detailed understanding of attribute sampling, including its procedures, applications, and interpretations. Key concepts discussed include sample size determination, confidence levels, and error margins.
Analysis: The information compiled in this guide is drawn from established statistical literature, focusing on practical applications and clear explanations. Emphasis is placed on providing readers with the tools to understand and apply attribute sampling effectively. This includes step-by-step examples and illustrative scenarios.
Key Takeaways:
- Attribute sampling estimates the proportion of a population with a specific characteristic.
- It's cost-effective and time-saving compared to inspecting the entire population.
- Sample size is crucial for accuracy and depends on confidence level and acceptable error.
- Different sampling plans (e.g., single, double, multiple) exist to optimize efficiency.
- Results are expressed as confidence intervals, reflecting the inherent uncertainty.
Attribute Sampling: A Deep Dive
Introduction
Attribute sampling is a statistical technique employed to determine the proportion of items in a population possessing a specific attribute or characteristic. Unlike variable sampling, which measures numerical values, attribute sampling focuses on qualitative aspects, classifying items as either conforming or non-conforming to a defined standard. This approach finds wide applications across numerous fields, including quality control, auditing, surveys, and market research. Its importance stems from its ability to provide reliable estimates about a large population with significantly reduced costs and time compared to full inspection.
Key Aspects of Attribute Sampling
- Defining the Attribute: Clearly defining the attribute of interest is paramount. Ambiguity can lead to inconsistent results.
- Sample Selection: The method of selecting the sample (random, stratified, systematic) critically affects the validity of the results. Random sampling is preferred for unbiased estimates.
- Sample Size Determination: Determining the appropriate sample size is crucial to ensure the results are statistically significant and representative of the population. This depends on factors like the desired confidence level and the acceptable margin of error.
- Statistical Inference: The results from the sample are used to make inferences about the entire population. This involves calculating confidence intervals, which quantify the uncertainty associated with the estimate.
Discussion of Key Aspects
Defining the Attribute
The accuracy of attribute sampling hinges on precise definition of the attribute. For example, in a quality control setting, the attribute might be "defective units," requiring a clear description of what constitutes a defect. In a customer satisfaction survey, the attribute could be "customer satisfaction," requiring a well-defined scale or criteria. Vague definitions lead to inconsistent classification and undermine the reliability of the results.
Sample Selection
Various sampling methods exist, each with its own advantages and disadvantages. Random sampling, where each item in the population has an equal chance of being selected, is often preferred to minimize bias. Stratified sampling divides the population into subgroups (strata) and samples from each stratum proportionally. Systematic sampling selects items at regular intervals. The choice of sampling method should align with the characteristics of the population and the research objectives.
Sample Size Determination
Calculating the appropriate sample size is critical. Larger samples generally lead to more precise estimates, but also increase costs and time. The determination involves considering three key factors:
- Confidence Level: The probability that the true population proportion lies within the calculated confidence interval (e.g., 95% confidence level).
- Acceptable Error (Margin of Error): The maximum allowable difference between the sample estimate and the true population proportion.
- Estimated Population Proportion: A preliminary estimate of the proportion of the population possessing the attribute. If no prior information is available, a conservative estimate of 50% is often used.
Specialized formulas or statistical software are used to calculate the necessary sample size based on these parameters.
Statistical Inference
After collecting the sample data, statistical methods are used to estimate the population proportion and construct a confidence interval. The confidence interval provides a range of values within which the true population proportion is likely to fall with the specified confidence level. This reflects the inherent uncertainty associated with using a sample to estimate a population parameter.
Attribute Sampling Plans: Single, Double, and Multiple
Several attribute sampling plans exist, each designed to optimize efficiency and accuracy based on specific needs:
- Single Sampling Plan: A single sample is selected, and a decision is made based on the number of non-conforming units found.
- Double Sampling Plan: If the results of the first sample are inconclusive, a second sample is taken to improve the precision of the estimate.
- Multiple Sampling Plan: Similar to double sampling, but allows for multiple samples if needed, providing even more flexibility.
The choice of plan depends on factors like the cost of sampling, the acceptable risk of making incorrect decisions, and the characteristics of the population.
Connecting Attribute Sampling to Specific Scenarios
Quality Control in Manufacturing
In manufacturing, attribute sampling plays a crucial role in quality assurance. By randomly selecting a sample of products from a production batch, manufacturers can estimate the proportion of defective units. This helps in assessing the overall quality of the batch and making informed decisions about whether to accept or reject the entire batch.
Facets of Attribute Sampling in Manufacturing:
- Role: To estimate the proportion of defective units within a production batch.
- Example: Inspecting a sample of 100 light bulbs to estimate the percentage of faulty bulbs.
- Risks & Mitigations: Type I error (rejecting a good batch) and Type II error (accepting a bad batch) can be minimized by careful sample size determination and selection of an appropriate sampling plan.
- Impacts & Implications: Improper sampling can lead to the release of defective products or unnecessary rejection of good products, affecting cost and reputation.
Auditing Financial Statements
Auditors use attribute sampling to assess the risk of material misstatements in financial statements. For example, they might sample a set of transactions to determine the proportion of those with errors. This helps in evaluating the reliability of the financial records and making informed judgements about the audit opinion.
Facets of Attribute Sampling in Auditing:
- Role: To estimate the proportion of errors or misstatements in a population of transactions.
- Example: Sampling invoices to estimate the percentage of those with incorrect amounts.
- Risks & Mitigations: The risk of sampling error is mitigated by using a sufficiently large and representative sample.
- Impacts & Implications: Inaccurate sampling can lead to incorrect audit opinions, potentially causing financial losses for stakeholders.
Frequently Asked Questions (FAQ)
FAQ
Introduction: This section addresses common questions and concerns about attribute sampling.
Questions:
-
What is the difference between attribute and variable sampling? Attribute sampling deals with qualitative characteristics (conforming/non-conforming), while variable sampling deals with quantitative measurements.
-
How do I choose the right sample size? Sample size depends on the desired confidence level, acceptable error, and estimated population proportion; specialized formulas or software are used for calculation.
-
What are the limitations of attribute sampling? Attribute sampling provides estimates, not exact figures; sampling error is inherent, and the results are subject to uncertainty.
-
Can attribute sampling be used for small populations? While it's generally used for large populations, it can be applied to smaller ones but may provide less precise estimates.
-
How do I interpret the results of attribute sampling? Results are usually expressed as confidence intervals, indicating the range of values within which the true population proportion is likely to fall.
-
What software can I use for attribute sampling? Many statistical software packages, such as Minitab, SPSS, and R, can be used to perform attribute sampling calculations.
Summary: Understanding the nuances of attribute sampling enables informed decision-making and minimizes risks associated with generalizations from samples to populations.
Tips for Effective Attribute Sampling
Tips of Attribute Sampling
Introduction: This section presents practical tips for effectively employing attribute sampling techniques.
Tips:
- Clearly define the attribute of interest: Ambiguity in the definition will lead to inconsistent results.
- Use a random sampling method: Avoid bias by ensuring each item in the population has an equal chance of selection.
- Determine the appropriate sample size: Use relevant statistical formulas or software to determine the necessary sample size based on the desired precision and confidence level.
- Document the sampling process: Maintain a detailed record of the sampling methodology and results for future reference and auditability.
- Use appropriate statistical software: Employ statistical software to facilitate the calculation of confidence intervals and interpretation of results.
- Consider the cost-benefit trade-off: Balance the cost of sampling against the need for precision.
- Interpret results cautiously: Remember that the results are estimates subject to sampling error, and always consider the confidence interval.
Summary: Following these tips can ensure the successful implementation of attribute sampling, producing reliable and meaningful results. It emphasizes the importance of careful planning, appropriate methodology, and cautious interpretation.
Summary of Attribute Sampling
Summary: This guide explored the definition, purpose, and mechanics of attribute sampling, a crucial statistical method for estimating the proportion of items in a population possessing a specific characteristic. The guide delved into key aspects such as defining the attribute, sample selection, sample size determination, and statistical inference, providing practical examples and explanations of different sampling plans.
Closing Message: Mastering attribute sampling empowers organizations with efficient tools for quality control, auditing, and research, offering insights into large populations without the cost and time constraints of complete enumeration. Continued exploration of advanced sampling techniques and the utilization of appropriate statistical software will further enhance the application and reliability of this valuable method.
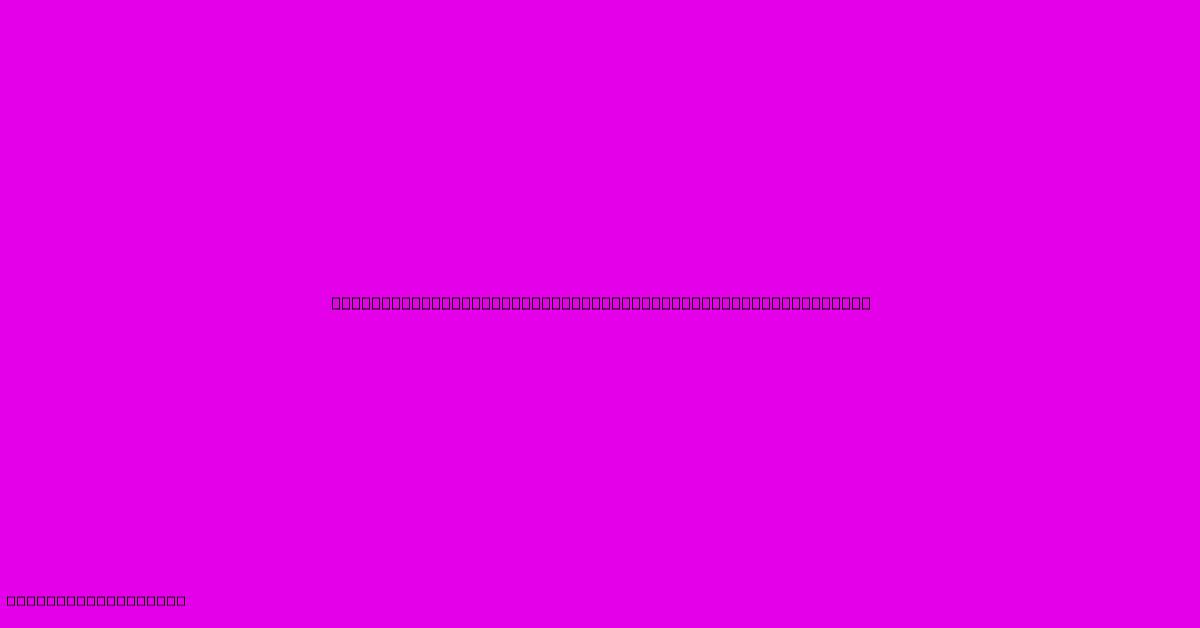
Thank you for taking the time to explore our website Attribute Sampling Definition Purpose And How It Works. We hope you find the information useful. Feel free to contact us for any questions, and don’t forget to bookmark us for future visits!
We truly appreciate your visit to explore more about Attribute Sampling Definition Purpose And How It Works. Let us know if you need further assistance. Be sure to bookmark this site and visit us again soon!
Featured Posts
-
How To Invest In Venture Capital Funds
Jan 10, 2025
-
Alberta Investment Management Corporation Aimco Definition
Jan 10, 2025
-
Accommodation Endorsement Definition
Jan 10, 2025
-
Relativity Trap Definition
Jan 10, 2025
-
What Is Accounting Technician
Jan 10, 2025