Benchmark Error Definition
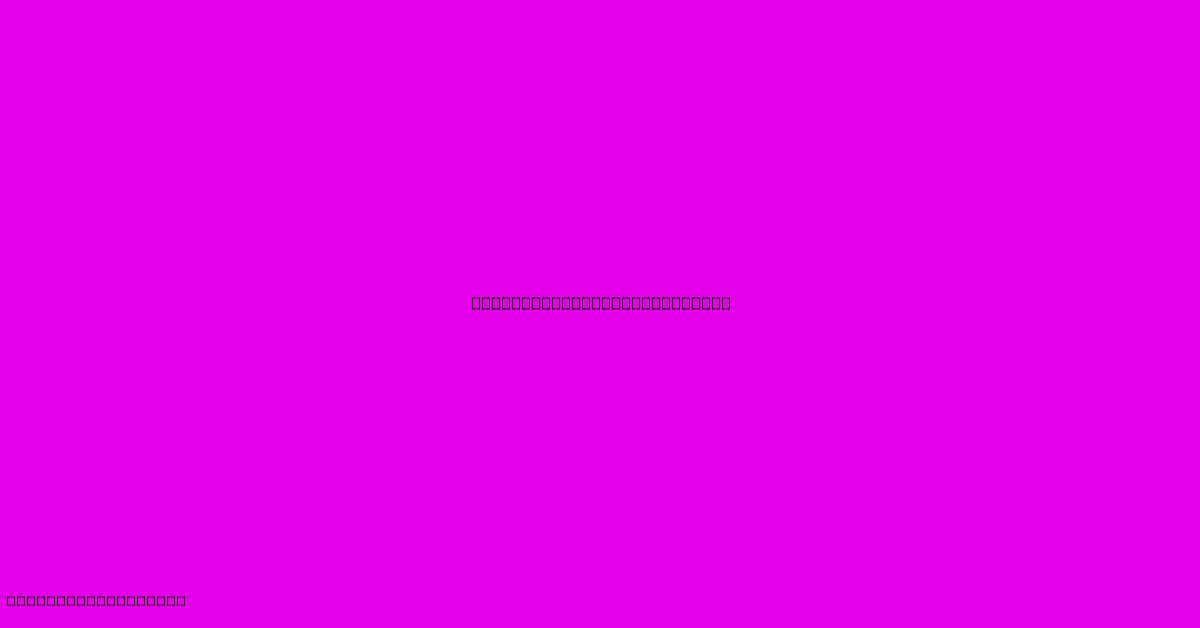
Discover more in-depth information on our site. Click the link below to dive deeper: Visit the Best Website meltwatermedia.ca. Make sure you don’t miss it!
Table of Contents
Unveiling Benchmark Error: A Comprehensive Guide to Accuracy and Precision
Hook: Does your benchmark data truly reflect reality, or are hidden errors skewing your results? The accuracy of your insights depends entirely on understanding and mitigating benchmark error.
Editor's Note: This comprehensive guide to benchmark error has been published today, offering valuable insights into its definition, sources, and mitigation strategies.
Importance & Summary: Benchmarking is crucial for evaluating performance, identifying areas for improvement, and driving strategic decision-making across various sectors. However, the validity of benchmark comparisons hinges on the accuracy of the benchmark data itself. This guide explores benchmark error—the difference between the measured benchmark value and the true value—detailing its various sources and offering strategies for minimizing its impact. We'll examine systematic and random errors, their influence on data analysis, and best practices for reliable benchmarking.
Analysis: The information compiled in this guide draws upon established statistical methodologies, peer-reviewed research in benchmarking practices, and case studies illustrating the consequences of unchecked benchmark error. We analyzed various error sources, their propagation mechanisms, and the impact on resulting interpretations.
Key Takeaways:
- Understanding benchmark error is critical for accurate performance assessments.
- Different types of errors exist, each requiring a specific mitigation approach.
- Robust data collection and validation processes are essential.
- Transparency and clear communication of limitations are vital.
Benchmark Error: A Deep Dive
Subheading: Benchmark Error Defined
Introduction: Benchmark error represents the discrepancy between the observed benchmark value and the true, underlying value. This discrepancy can stem from various sources, leading to inaccurate conclusions and potentially flawed decision-making. Understanding the sources and types of benchmark error is crucial for ensuring the reliability and validity of any benchmarking exercise.
Key Aspects:
- Systematic Error: Consistent and predictable deviations from the true value.
- Random Error: Inconsistent and unpredictable fluctuations, typically due to chance.
- Measurement Error: Inaccuracies in the data collection process itself.
- Sampling Error: Errors arising from the selection of the benchmark sample.
- Data Reporting Error: Inaccuracies or inconsistencies in how data is recorded and reported.
Discussion:
-
Systematic Error: This type of error introduces a consistent bias into the benchmark data. For example, if a specific measurement instrument is consistently miscalibrated, all measurements will be systematically off by a certain amount. This could lead to underestimating or overestimating the true performance. Mitigation strategies include calibrating instruments regularly, using multiple independent measurement methods, and employing rigorous quality control checks during data collection. The impact of systematic error can be significant, potentially leading to incorrect conclusions about relative performance.
-
Random Error: Unlike systematic error, random error is unpredictable and varies randomly around the true value. Factors such as human error in data entry or minor fluctuations in environmental conditions can contribute to random error. The impact of random error can be lessened through techniques like increasing the sample size, employing statistical methods to average out fluctuations, and using robust data analysis techniques. Larger sample sizes generally lead to more reliable estimates and reduced random error.
-
Measurement Error: This refers to inaccuracies stemming directly from the process of measuring and recording data. This could involve issues with the measurement tools themselves, human error during data collection, or limitations in the data capture methodology. For example, using an outdated or poorly maintained device to measure processing speed will yield inaccurate results. Addressing measurement error requires careful selection and calibration of instruments, detailed training of personnel involved in data collection, and rigorous quality assurance protocols. The impact on benchmarking is a direct distortion of the true performance.
-
Sampling Error: This type of error arises when the selected sample does not accurately represent the population being studied. For instance, if a benchmark focuses on a small, non-representative sample of companies, the results may not be generalizable to the broader industry. Stratified sampling techniques, ensuring appropriate representation across various subgroups, can help mitigate sampling error. Overreliance on convenience sampling can lead to biased results that do not reflect the true population characteristics.
-
Data Reporting Error: Inaccuracies or inconsistencies in how data is recorded and reported can significantly affect the reliability of benchmarks. This may involve human errors in data entry, inconsistencies in data formatting, or the use of inappropriate units of measurement. Standardized data entry protocols, rigorous data validation processes, and clear reporting guidelines are essential for minimizing data reporting error. Even minor inconsistencies can accumulate and significantly distort benchmark results.
Subheading: Sources of Benchmark Error
Introduction: Identifying the sources of benchmark error is crucial for effective mitigation strategies. A thorough understanding of potential pitfalls aids in the design of a robust and reliable benchmarking process.
Facets:
-
Title: Instrument Calibration
- Explanation: Inaccurate calibration of measurement instruments leads to consistent bias.
- Example: A faulty speedometer consistently under-reports speed, leading to an underestimation of travel time in transportation benchmarking.
- Risk & Mitigation: Regular calibration and verification of instrument accuracy.
- Impact & Implications: Biased benchmark results, incorrect conclusions.
-
Title: Data Collection Procedures
- Explanation: Poorly defined or inconsistently applied data collection procedures introduce variability.
- Example: Unclear instructions on how to measure customer satisfaction can lead to varying interpretation and inconsistent data.
- Risk & Mitigation: Standardized procedures, detailed instructions, and training for data collectors.
- Impact & Implications: Inconsistent and unreliable data, hindering accurate comparisons.
-
Title: Data Entry Errors
- Explanation: Human errors during data entry introduce inaccuracies into the dataset.
- Example: Mistyping numerical values or misinterpreting data codes.
- Risk & Mitigation: Data validation checks, double entry procedures, and automated data entry systems.
- Impact & Implications: Inaccurate data, potentially leading to misleading benchmark results.
-
Title: Sample Bias
- Explanation: Selection of a sample that does not represent the overall population.
- Example: Benchmarking based solely on large corporations may not reflect the performance of smaller businesses.
- Risk & Mitigation: Stratified sampling, random sampling, ensuring representative samples.
- Impact & Implications: Results that cannot be generalized to the broader population.
Summary: Understanding the multiple sources of benchmark error, from instrument limitations to sampling biases, is crucial for establishing the credibility of benchmarking exercises. Addressing each source, through preventative measures and mitigation strategies, significantly improves data accuracy and enhances the value of benchmark comparisons.
Subheading: Mitigating Benchmark Error
Introduction: Effective mitigation of benchmark error requires a multi-faceted approach, addressing all potential sources of error throughout the benchmarking process.
Further Analysis: Implementing rigorous quality control measures at each stage—from data collection to analysis—significantly reduces the chances of inaccurate results. Using statistical methods to identify outliers and adjust for bias is crucial. Clear communication of the limitations of the benchmark data is essential to ensure responsible interpretation of the results.
Closing: Addressing benchmark error is not just a matter of technical precision but a fundamental requirement for reliable decision-making. By incorporating robust methodologies and transparent communication, organizations can leverage benchmarking to its full potential, driving informed strategies and effective performance improvement.
Subheading: FAQ
Introduction: This section addresses frequently asked questions related to benchmark error.
Questions:
-
Q: What is the most significant type of benchmark error? A: The most significant type depends on the context, but systematic errors often have the most substantial impact because they consistently bias results.
-
Q: How can I reduce random error in my benchmarking? A: Increase sample size, use robust statistical methods, and improve data collection consistency.
-
Q: How do I identify systematic error? A: Compare your results with other independent data sources or re-measure using different methods. Consistent deviations suggest systematic error.
-
Q: What are the consequences of ignoring benchmark error? A: Inaccurate performance assessments, flawed decision-making, and missed opportunities for improvement.
-
Q: How can I improve the accuracy of my benchmark data? A: Employ standardized procedures, use calibrated instruments, and implement rigorous quality control measures throughout the process.
-
Q: What is the role of transparency in benchmarking? A: Openly communicating limitations, uncertainties, and potential sources of error builds trust and prevents misinterpretations of the benchmark results.
Summary: Addressing these common concerns highlights the importance of comprehensive data management and analytical rigor in benchmarking processes.
Transition: Understanding these frequently asked questions lays the groundwork for implementing practical strategies to minimize benchmark error.
Subheading: Tips for Minimizing Benchmark Error
Introduction: This section offers practical advice for reducing the impact of benchmark error in your benchmarking projects.
Tips:
- Develop Standardized Procedures: Create detailed and consistent data collection and analysis protocols.
- Calibrate Instruments Regularly: Ensure that measurement devices are accurate and properly calibrated.
- Employ Robust Statistical Methods: Use statistical techniques to identify and account for outliers and biases.
- Increase Sample Size: Larger samples generally lead to more reliable and representative results.
- Implement Data Validation Checks: Regularly verify the accuracy and consistency of the data.
- Utilize Multiple Data Sources: Compare results from different sources to identify potential discrepancies.
- Document Limitations and Assumptions: Clearly articulate the limitations and potential sources of error in the benchmark data.
- Seek External Validation: Have an independent expert review your data and methodology.
Summary: By following these tips, organizations can significantly improve the accuracy and reliability of their benchmarking efforts.
Transition: Implementing these strategies results in more credible benchmark data and informed decision-making.
Subheading: Conclusion
Summary: This guide explored the multifaceted nature of benchmark error, highlighting its sources, types, and mitigation strategies. Accurate benchmarking hinges on the precision of data collection, rigorous analysis, and transparent communication of limitations.
Closing Message: Understanding and minimizing benchmark error is not merely a technical detail; it's a cornerstone of effective benchmarking and data-driven decision-making. By consistently applying sound methodological practices and prioritizing data quality, organizations can unlock the full potential of benchmarking for strategic advantage.
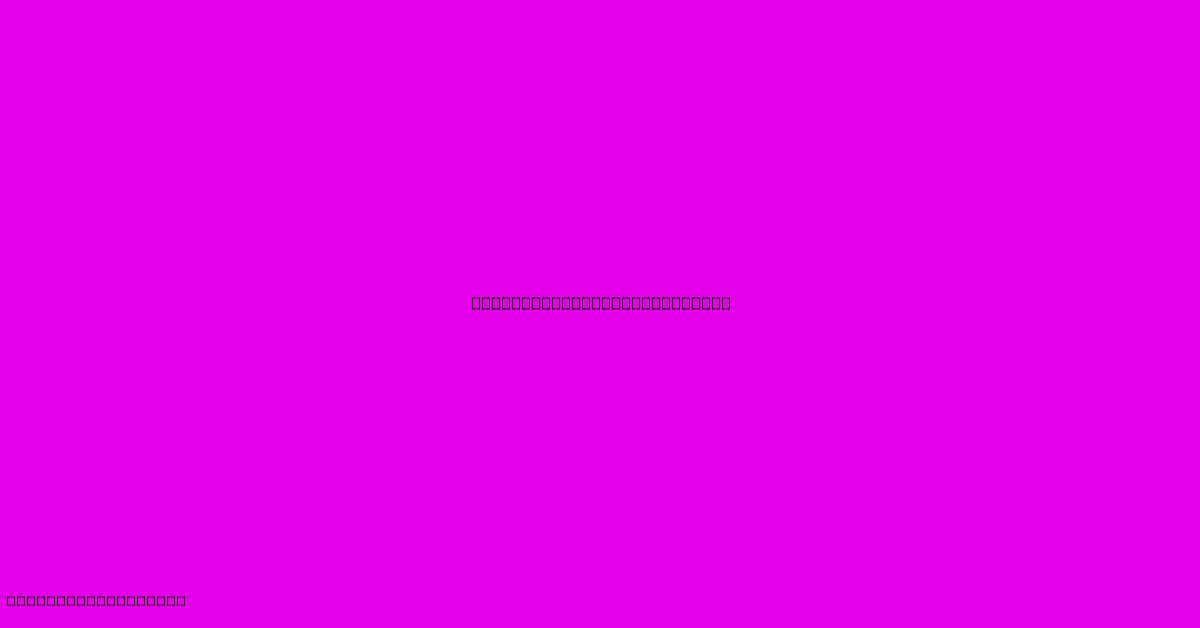
Thank you for taking the time to explore our website Benchmark Error Definition. We hope you find the information useful. Feel free to contact us for any questions, and don’t forget to bookmark us for future visits!
We truly appreciate your visit to explore more about Benchmark Error Definition. Let us know if you need further assistance. Be sure to bookmark this site and visit us again soon!
Featured Posts
-
What Is The Difference Between I Bonds And Tips
Jan 07, 2025
-
When Is Dba Insurance Required
Jan 07, 2025
-
European Best Bid And Offer Ebbo Definition
Jan 07, 2025
-
What To Do If Your Car Is Stolen Without Insurance
Jan 07, 2025
-
What Are The Minimum Requirements For Auto Insurance In Texas
Jan 07, 2025