Benchmark For Correlation Values Definition
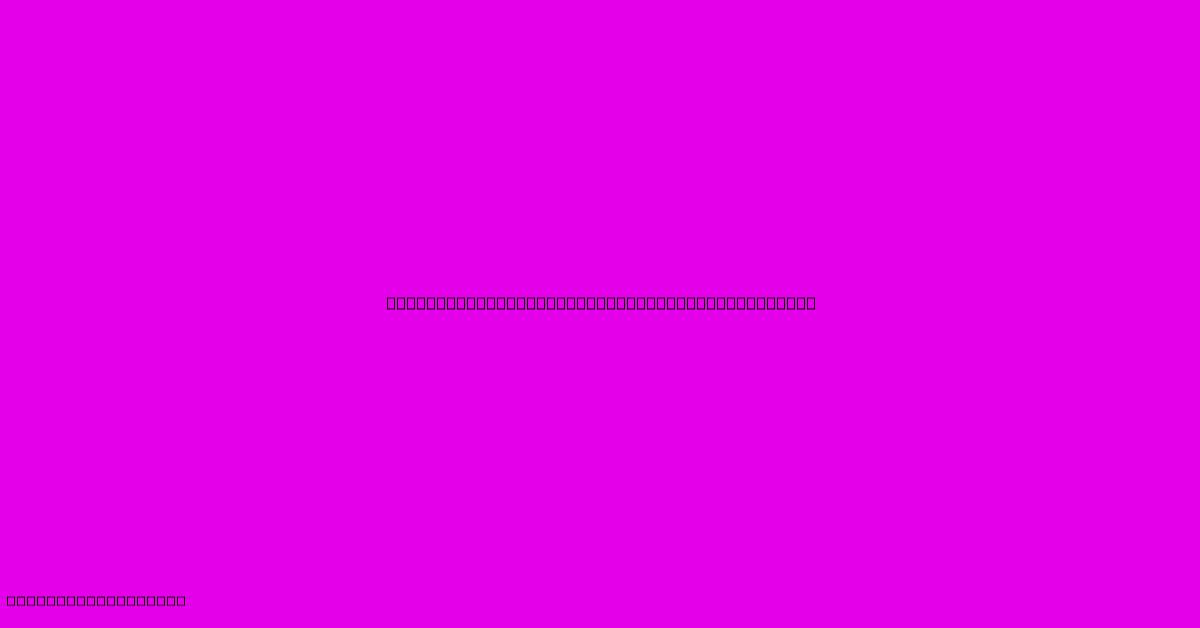
Discover more in-depth information on our site. Click the link below to dive deeper: Visit the Best Website meltwatermedia.ca. Make sure you don’t miss it!
Table of Contents
Benchmark for Correlation Values: Definition, Interpretation, and Application
What determines a "strong" correlation? Is there a universally accepted benchmark? The answer is more nuanced than a simple yes or no. This article explores the definition and interpretation of correlation values, examining the benchmarks used across various fields and highlighting the crucial context needed for proper analysis.
Editor's Note: This comprehensive guide to correlation value benchmarks has been published today to provide clarity and insight into interpreting correlation coefficients.
Importance & Summary: Understanding correlation benchmarks is critical for correctly interpreting statistical relationships between variables. This guide summarizes the common benchmarks (e.g., Pearson's r, Spearman's rho), discusses factors influencing their interpretation, and explores their application across different disciplines. It clarifies the limitations of relying solely on numerical benchmarks and emphasizes the importance of considering contextual factors. Topics include the difference between correlation strength and significance, and the impact of sample size and data distribution.
Analysis: This analysis compiles information from statistical literature, research articles across diverse fields, and established statistical guidelines to provide a comprehensive overview of correlation value benchmarks. The aim is to offer a practical guide for researchers, analysts, and anyone interpreting correlation data.
Key Takeaways:
- Correlation values range from -1 to +1.
- Benchmarks are guidelines, not strict rules.
- Context (field, research question) is paramount.
- Significance testing is crucial alongside magnitude.
- Non-linear relationships may not show up in simple correlation.
Correlation Values: Unveiling the Relationship Between Variables
Correlation analysis assesses the strength and direction of a linear relationship between two or more variables. The most common measure is Pearson's correlation coefficient (r), suitable for continuous variables with a linear relationship and approximately normal distribution. Spearman's rank correlation coefficient (ρ), on the other hand, is used for ordinal or non-normal data, assessing monotonic relationships (i.e., consistently increasing or decreasing).
Key Aspects of Correlation Benchmarks:
- Magnitude: This refers to the absolute value of the correlation coefficient (|r| or |ρ|). A higher absolute value indicates a stronger relationship.
- Direction: The sign (+ or -) indicates the direction of the relationship. A positive correlation means as one variable increases, the other tends to increase. A negative correlation means as one variable increases, the other tends to decrease.
- Significance: Statistical significance tests determine the likelihood that an observed correlation is due to chance. A significant correlation (p-value typically below 0.05) suggests the relationship is unlikely to be random.
Interpreting Correlation Coefficients: Benchmarks and Nuances
While various guidelines exist, there's no universal benchmark for "strong" or "weak" correlation. The interpretation depends heavily on the specific field and research question. Below are commonly cited interpretations, acknowledging their contextual limitations:
| Magnitude (|r| or |ρ|) | Interpretation (General Guideline) | |---|---| | 0.00 - 0.19 | Very weak or negligible correlation | | 0.20 - 0.39 | Weak correlation | | 0.40 - 0.59 | Moderate correlation | | 0.60 - 0.79 | Strong correlation | | 0.80 - 1.00 | Very strong or perfect correlation |
It's crucial to understand that these are guidelines, not rigid rules. In some fields, a correlation of 0.4 might be considered strong, while in others, it could be considered weak. For instance, in social sciences, correlations around 0.3 might be considered meaningful, reflecting the complexity of human behavior. In contrast, physics or engineering often demand much stronger correlations for establishing robust relationships.
Subheading: Sample Size and Statistical Significance
The sample size significantly influences the interpretation of correlation coefficients. With larger samples, even small correlations can achieve statistical significance. Conversely, a large correlation in a small sample may not be statistically significant. Therefore, considering both the magnitude and statistical significance (p-value) is essential.
Beyond the Numbers: Contextual Factors
Several contextual factors should be considered alongside numerical benchmarks:
- Field of study: The accepted benchmarks differ across disciplines. What's considered a strong correlation in one field may be weak in another.
- Research question: The strength of correlation needed to support a specific hypothesis varies. A small but statistically significant correlation might be meaningful if it confirms a predicted relationship.
- Data distribution: Departures from normality can affect the interpretation of Pearson's r. Spearman's ρ is more robust to non-normality.
- Causation vs. Correlation: Correlation doesn't imply causation. Even a strong correlation doesn't prove that one variable causes changes in another. Confounding variables or spurious relationships must be considered.
Subheading: Application of Correlation Benchmarks Across Disciplines
Correlation analysis finds applications in diverse fields:
- Medicine: Examining the relationship between risk factors and diseases. A strong correlation between smoking and lung cancer, for instance, is a crucial piece of evidence.
- Finance: Assessing the relationship between asset prices or market trends. Correlation analysis helps in portfolio diversification and risk management.
- Economics: Studying the relationship between economic indicators (e.g., inflation and unemployment).
- Psychology: Investigating correlations between personality traits or psychological measures.
- Environmental Science: Analyzing the correlation between environmental factors and ecological changes.
Subheading: Limitations and Considerations
While correlation analysis is a valuable tool, it has limitations:
- Non-linear relationships: Correlation coefficients primarily capture linear relationships. Non-linear relationships might be missed if only simple correlation is used. Scatter plots should always be examined.
- Outliers: Extreme values can disproportionately influence correlation coefficients. Careful consideration of outliers is crucial.
- Confounding variables: Correlation does not imply causation. Other unmeasured variables might be driving the observed correlation.
FAQ
Subheading: FAQ
Introduction: This section addresses frequently asked questions concerning correlation value benchmarks.
Questions:
-
Q: What's the difference between Pearson's r and Spearman's rho? A: Pearson's r measures linear correlations between continuous variables with normal distributions, while Spearman's rho measures monotonic correlations between ordinal or non-normal data.
-
Q: Is a p-value of 0.01 always more significant than a p-value of 0.05? A: Yes, a lower p-value indicates stronger evidence against the null hypothesis (no correlation).
-
Q: Can a correlation coefficient be negative? A: Yes, a negative coefficient indicates an inverse relationship: as one variable increases, the other tends to decrease.
-
Q: What if my correlation is statistically significant but the magnitude is weak? A: This could be due to a large sample size. The practical importance of a weak correlation, even if statistically significant, might be limited.
-
Q: How do I visualize correlation data? A: Scatter plots provide a visual representation of the relationship between two variables, making it easier to detect non-linear relationships or outliers.
-
Q: What if my data doesn't meet the assumptions of Pearson's correlation? A: Consider using alternative methods like Spearman's rank correlation or other non-parametric techniques.
Summary: Understanding the nuances of correlation analysis requires careful consideration of both magnitude, significance, and contextual factors.
Transition: Let's now explore practical tips for effective correlation analysis.
Tips for Effective Correlation Analysis
Subheading: Tips for Effective Correlation Analysis
Introduction: This section provides practical advice for conducting and interpreting correlation analysis effectively.
Tips:
- Visualize your data: Always start with scatter plots to visually inspect the relationship between variables before calculating correlation coefficients.
- Check assumptions: Ensure your data meets the assumptions of the chosen correlation method (e.g., normality for Pearson's r).
- Consider outliers: Identify and address outliers that may unduly influence the results.
- Test for significance: Always perform a statistical significance test to determine the likelihood that the observed correlation is due to chance.
- Report both magnitude and significance: Provide both the correlation coefficient and its associated p-value.
- Consider alternative methods: If your data doesn't meet the assumptions of Pearson's r, use Spearman's rho or other appropriate methods.
- Interpret in context: The interpretation of correlation coefficients depends heavily on the field of study and research question.
- Avoid causal inferences: Remember that correlation does not imply causation.
Summary: Following these tips will improve the accuracy and interpretability of your correlation analyses.
Transition: Let's summarize the key insights from this exploration of correlation value benchmarks.
Summary of Correlation Value Benchmarks
Summary: This article explored the definition and interpretation of correlation values, emphasizing that benchmarks are guidelines, not rigid rules. The interpretation depends on the field of study, research question, sample size, and statistical significance. Contextual factors and limitations of correlation analysis (non-linear relationships, outliers, confounding variables) were discussed. Practical tips for effective correlation analysis were also provided.
Closing Message: Understanding and interpreting correlation coefficients requires careful consideration of both the numerical values and their broader context. This guide serves as a foundation for more nuanced analyses, encouraging researchers to move beyond simplistic interpretations and to integrate diverse analytical approaches for comprehensive insights. Further exploration of more advanced techniques (e.g., partial correlation) is encouraged for situations with confounding variables.
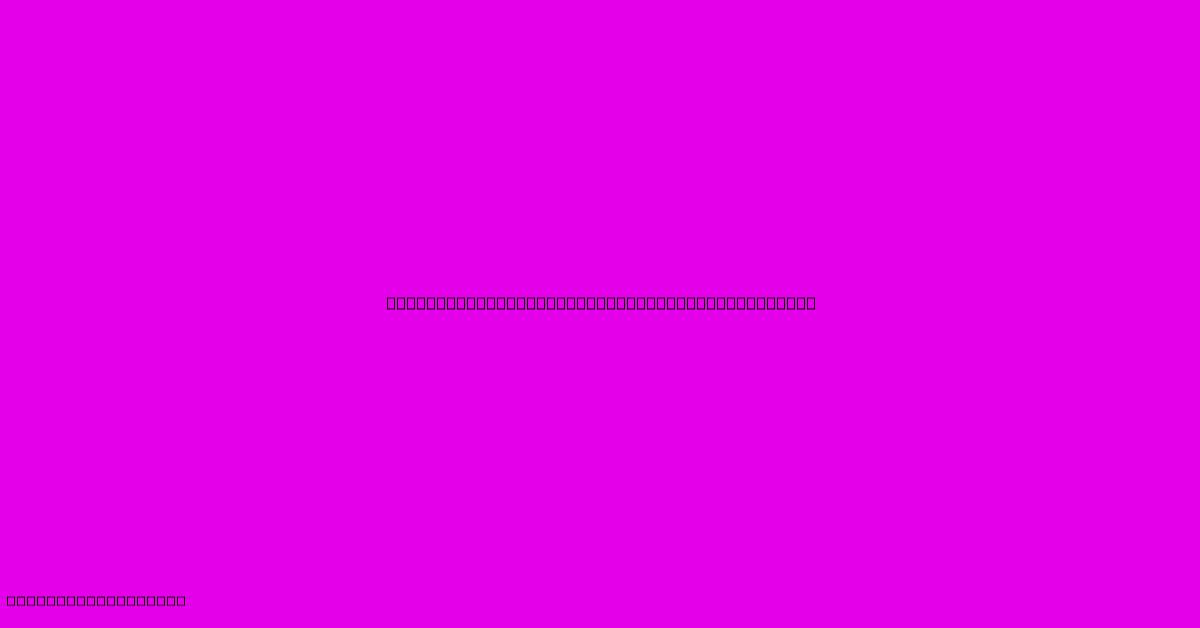
Thank you for taking the time to explore our website Benchmark For Correlation Values Definition. We hope you find the information useful. Feel free to contact us for any questions, and don’t forget to bookmark us for future visits!
We truly appreciate your visit to explore more about Benchmark For Correlation Values Definition. Let us know if you need further assistance. Be sure to bookmark this site and visit us again soon!
Featured Posts
-
Current Account Surplus Definition And Countries That Have It
Jan 07, 2025
-
Base Metals Definition Uses Trading Investing
Jan 07, 2025
-
How To Remove Keratin Bonds At Home
Jan 07, 2025
-
What Happens If You Dont Have Life Insurance
Jan 07, 2025
-
Decamillionaire Definition Word Origin Uses
Jan 07, 2025