Decision Analysis Da Definition Uses And Examples
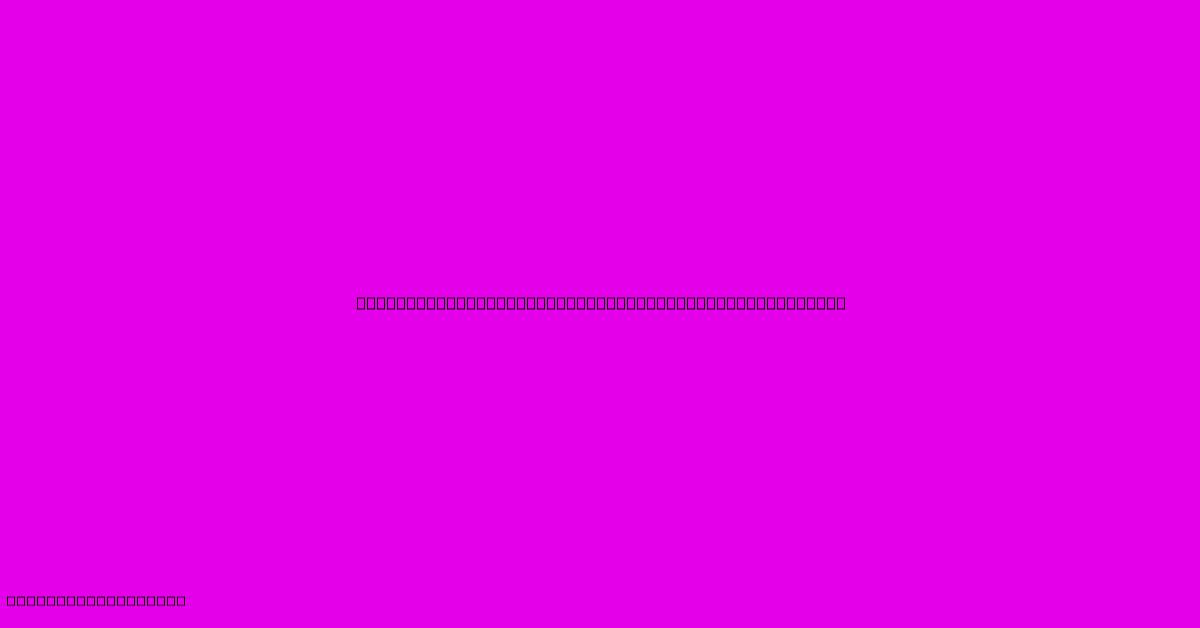
Discover more in-depth information on our site. Click the link below to dive deeper: Visit the Best Website meltwatermedia.ca. Make sure you don’t miss it!
Table of Contents
Unlocking Strategic Decisions: A Deep Dive into Decision Analysis (DA)
Editor's Note: This comprehensive guide to Decision Analysis (DA) has been published today, offering valuable insights and practical applications for strategic decision-making.
Importance & Summary: Decision Analysis (DA) is a crucial methodology for navigating complex choices in various fields, from business and engineering to healthcare and public policy. This guide will explore DA's definition, uses, and illustrative examples, demonstrating its power in optimizing decision-making processes and outcomes. We'll examine key aspects like decision trees, influence diagrams, and utility theory, providing a robust understanding of DA's practical applications.
Analysis: The information compiled for this guide is drawn from a synthesis of leading academic texts on decision analysis, real-world case studies from diverse industries, and practical applications observed across various professional settings. The goal is to present a clear, concise, and actionable framework for understanding and utilizing DA in diverse contexts.
Key Takeaways:
- DA provides a structured approach to complex decisions.
- It incorporates quantitative and qualitative factors.
- DA utilizes various tools, including decision trees and influence diagrams.
- It facilitates risk assessment and mitigation.
- DA improves decision-making transparency and accountability.
Decision Analysis: A Structured Approach to Complex Choices
Decision Analysis (DA) is a systematic and analytical approach to making decisions, especially those involving uncertainty, risk, and multiple stakeholders. It's a multi-disciplinary field drawing on principles from mathematics, statistics, economics, and psychology to provide a framework for evaluating options and making informed choices. DA differs from intuitive or ad-hoc decision-making by explicitly acknowledging uncertainty and incorporating quantitative analysis to optimize decision outcomes.
Key Aspects of Decision Analysis:
- Problem Structuring: Clearly defining the decision problem, identifying objectives, and establishing the scope of analysis.
- Uncertainty Modeling: Representing and quantifying uncertainties associated with future events or outcomes.
- Preference Modeling: Determining decision-makers' preferences and values through utility assessment.
- Decision Model Building: Developing a formal model representing the decision problem, incorporating uncertainties, and preferences.
- Analysis and Evaluation: Analyzing the model to determine the optimal decision strategy, considering risk and sensitivity.
- Implementation and Monitoring: Implementing the chosen strategy and monitoring its performance to inform future decisions.
Discussion of Key Aspects:
1. Problem Structuring: This crucial initial step involves clearly articulating the decision problem, identifying the decision-maker(s), and defining the objectives. For example, a pharmaceutical company might frame its problem as "Should we launch a new drug given uncertain market demand and regulatory approval?" Subsequent steps depend on the clarity and specificity of this initial framing. The scope of the analysis must also be carefully defined to manage complexity and ensure the analysis remains relevant and manageable.
2. Uncertainty Modeling: DA explicitly addresses the uncertainties inherent in many decisions. This often involves identifying key uncertain factors (e.g., market size, competitor actions, technological advancements) and quantifying their potential impacts using probability distributions. For instance, instead of assuming a single market size for the new drug, the pharmaceutical company might use a probability distribution reflecting the range of possible market sizes, based on market research and expert judgment.
3. Preference Modeling: DA utilizes utility theory to represent decision-makers' preferences for different outcomes. Utility functions assign numerical values to outcomes reflecting their desirability. In our example, the pharmaceutical company might assign higher utility to outcomes with greater profitability and lower risk of regulatory failure. This step ensures the analysis aligns with the company's strategic goals and risk tolerance.
4. Decision Model Building: Decision models, often represented as decision trees or influence diagrams, provide a visual and quantitative representation of the decision problem. Decision trees depict possible sequences of events and decisions, while influence diagrams represent the relationships between variables and decision nodes. These models integrate the elements of problem structuring, uncertainty modeling, and preference modeling to create a comprehensive representation of the decision landscape.
5. Analysis and Evaluation: This stage involves analyzing the decision model to determine the optimal strategy. Techniques such as expected value calculations, sensitivity analysis, and decision tree analysis are employed to identify the option that maximizes the expected utility, considering the probabilities and preferences. Sensitivity analysis examines how the optimal decision changes with variations in uncertain inputs, helping to understand the robustness of the chosen strategy.
6. Implementation and Monitoring: Once a decision is made, its implementation is crucial. DA also emphasizes monitoring the outcomes and comparing them to the model's predictions. This feedback loop provides insights for refining future models and improving decision-making processes. Regular monitoring and adaptation are vital to ensure the chosen strategy remains effective in the face of changing circumstances.
Decision Tree Analysis: A Visual Representation of Decisions
Decision tree analysis is a popular technique within DA. It graphically depicts the decision problem as a tree-like structure, showing sequential decisions and their potential outcomes. Each branch represents a possible decision or event, and each node represents a decision point or an outcome. Probabilities are assigned to uncertain events, and utilities are assigned to the final outcomes. The analysis then determines the optimal decision strategy by maximizing expected utility.
Facets of Decision Tree Analysis:
- Roles: Decision trees help visualize complex scenarios, clarify uncertainties, and explicitly represent preferences.
- Examples: Evaluating investment opportunities, choosing marketing strategies, or assessing medical treatment options.
- Risks and Mitigations: Oversimplification of the problem or inaccurate probability and utility estimates can lead to suboptimal decisions. Rigorous data collection and expert judgment can mitigate these risks.
- Impacts and Implications: Decision trees improve transparency, facilitate communication, and support informed, data-driven decision-making.
Influence Diagrams: A Network Representation of Relationships
Influence diagrams offer a more compact representation of complex decisions involving multiple variables and uncertain relationships. They visually represent the causal relationships between variables and decision nodes using a network structure. Influence diagrams provide a high-level overview of the decision problem, making it easier to identify key variables and their interdependencies. They can be particularly useful for analyzing more intricate problems than easily handled by decision trees.
Facets of Influence Diagrams:
- Roles: Influence diagrams illustrate the dependencies between variables, showing how changes in one variable can impact others.
- Examples: Modeling supply chain dynamics, analyzing the effects of policy changes, or understanding the interplay between economic factors and consumer behavior.
- Risks and Mitigations: Incorrect specification of relationships or incomplete information can affect the analysis's validity. Sensitivity analysis and expert consultation can help mitigate these risks.
- Impacts and Implications: Influence diagrams enhance understanding of complex systems, facilitate collaboration, and support more robust decision-making processes.
Real-World Examples of Decision Analysis Applications:
- Healthcare: Decision analysis is used to assess the cost-effectiveness of new medical treatments, evaluate diagnostic strategies, and optimize resource allocation.
- Energy: Energy companies employ decision analysis to evaluate investment decisions in renewable energy projects, assess risks associated with exploration and production, and optimize power grid operations.
- Finance: Decision analysis is critical in portfolio management, evaluating investment strategies, and assessing the risks of financial instruments.
- Marketing: Marketing professionals utilize DA to design effective marketing campaigns, segment target markets, and optimize pricing strategies.
- Engineering: Engineers apply DA to optimize system design, assess risks in engineering projects, and make crucial decisions about maintenance and reliability.
FAQ: Addressing Common Questions about Decision Analysis
Introduction:
This section addresses frequently asked questions about Decision Analysis (DA).
Questions:
Q1: What are the limitations of Decision Analysis? A1: DA requires careful structuring and quantification, which can be time-consuming and resource-intensive. Subjective judgments about probabilities and utilities are inherent, and the models' accuracy depends on the quality of the input data.
Q2: Can DA handle highly uncertain situations? A2: Yes, DA excels in handling uncertainty. Probabilistic methods are used to represent uncertain events and assess the potential impacts of different outcomes. Sensitivity analysis helps identify critical uncertainties that warrant closer scrutiny.
Q3: How can I learn more about Decision Analysis techniques? A3: Numerous resources are available, including academic textbooks, online courses, and professional development programs. Many universities offer courses in decision analysis and related fields.
Q4: What software tools are used for Decision Analysis? A4: Several software packages support DA, offering decision tree and influence diagram modeling capabilities. Some commonly used options include TreeAge Pro, Analytica, and R with specific packages.
Q5: Is DA suitable for all types of decisions? A5: While DA is a powerful tool, it might not be the best approach for every decision. Simple, straightforward decisions may not require the rigor of formal DA. The most appropriate methodology depends on the decision's complexity and the availability of data and resources.
Q6: How can I ensure the accuracy of my DA model? A6: Thorough data collection, expert judgment elicitation, and rigorous testing are essential for ensuring model accuracy. Sensitivity analysis can identify critical assumptions and help assess the model's robustness.
Summary:
This FAQ section provided answers to common questions about decision analysis, addressing its limitations, applicability, and best practices.
Tips for Effective Decision Analysis
Introduction:
This section provides actionable tips for effectively applying decision analysis techniques.
Tips:
- Clearly define the problem: Begin by articulating the decision problem and objectives explicitly.
- Identify key uncertainties: Thoroughly identify all relevant uncertain factors and quantify their potential impacts.
- Elicit expert judgment: Involve experts to provide insights and inform probability and utility assessments.
- Use appropriate modeling techniques: Select decision tree analysis, influence diagrams, or other methods based on the decision's complexity.
- Perform sensitivity analysis: Assess how the optimal decision changes with variations in uncertain input parameters.
- Clearly communicate results: Present the analysis results in a clear, concise manner that is easily understandable by all stakeholders.
- Monitor and adapt: Track the outcomes and compare them with the model’s predictions to inform future decisions.
- Iterate and refine: Refine the model and decision-making process based on experience and feedback.
Summary:
These tips provide guidance for applying decision analysis effectively, ensuring informed, data-driven decision-making.
Summary of Decision Analysis
This guide explored the principles and applications of Decision Analysis (DA), a powerful methodology for making strategic choices in complex situations. DA provides a structured framework for navigating uncertainty, assessing risk, and maximizing expected utility. The guide illustrated DA's use through decision tree analysis and influence diagrams, demonstrating their application in various domains.
Closing Message:
By embracing the principles and techniques of Decision Analysis, organizations and individuals can enhance their decision-making capabilities, improving outcomes and achieving strategic objectives. The adoption of data-driven, structured approaches like DA is crucial in today's increasingly complex and uncertain world. A commitment to continuous learning and refinement of DA methodologies will lead to better and more informed decisions across all sectors.
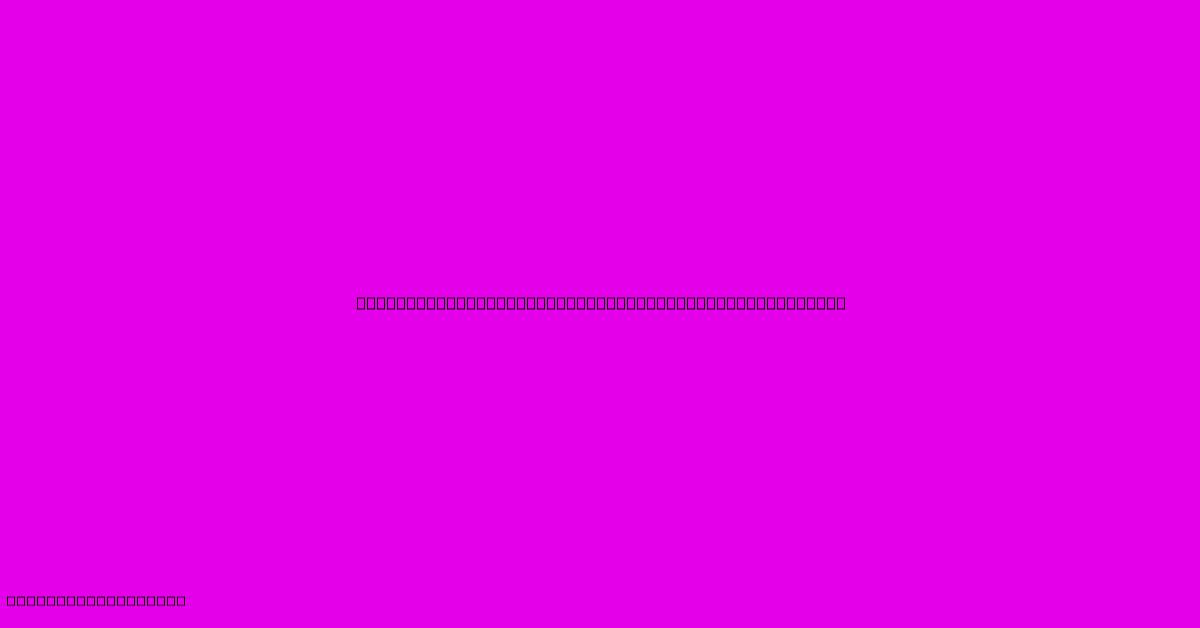
Thank you for taking the time to explore our website Decision Analysis Da Definition Uses And Examples. We hope you find the information useful. Feel free to contact us for any questions, and don’t forget to bookmark us for future visits!
We truly appreciate your visit to explore more about Decision Analysis Da Definition Uses And Examples. Let us know if you need further assistance. Be sure to bookmark this site and visit us again soon!
Featured Posts
-
What Insurance Covers Mounjaro For Weight Loss
Jan 07, 2025
-
Best Ask Definition
Jan 07, 2025
-
Face Value Definition In Finance Comparison With Market Value
Jan 07, 2025
-
What Is Face Value In Bonds
Jan 07, 2025
-
How Much Is Ajovy With Insurance
Jan 07, 2025