Empirical Rule Definition Formula Example How Its Used
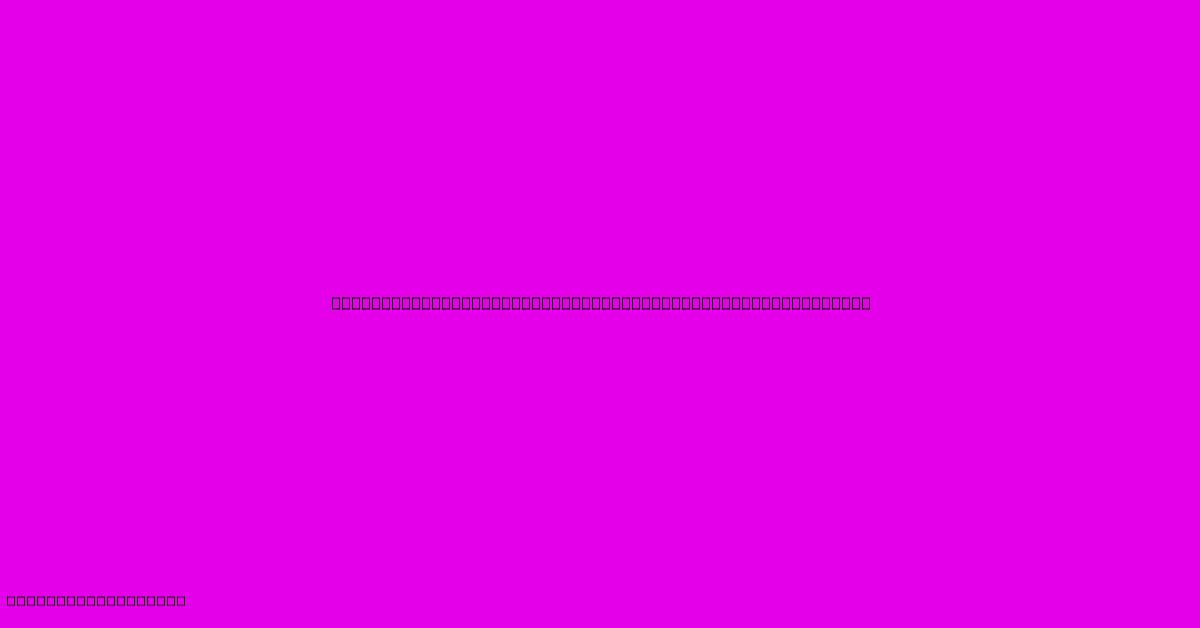
Discover more in-depth information on our site. Click the link below to dive deeper: Visit the Best Website meltwatermedia.ca. Make sure you don’t miss it!
Table of Contents
Unlocking the Secrets of the Empirical Rule: Definition, Formula, and Real-World Applications
Hook: Ever wondered how much data falls within a specific range around the average? The empirical rule, also known as the 68-95-99.7 rule, provides a powerful and surprisingly simple answer, revealing the hidden patterns within normally distributed data.
Editor's Note: This comprehensive guide to the empirical rule has been published today, offering a deep dive into its definition, formula, applications, and practical examples.
Importance & Summary: The empirical rule is a cornerstone of descriptive statistics, providing a quick way to understand the distribution of data around the mean. It's widely applicable across various fields, from finance and healthcare to engineering and quality control, enabling efficient data analysis and informed decision-making. This guide will delve into the rule's mathematical foundation, explore its practical applications, and illustrate its use with clear examples. It will cover the key concepts of normal distribution, standard deviation, and z-scores, clarifying their roles in applying the empirical rule effectively.
Analysis: This analysis synthesizes information from numerous statistical textbooks and research papers to provide a clear and concise explanation of the empirical rule. The explanation avoids complex mathematical derivations, focusing instead on its practical application and interpretation. Real-world examples from diverse fields are used to illustrate the rule's versatility and importance.
Key Takeaways:
- The empirical rule applies only to normally distributed data.
- It estimates the percentage of data points falling within one, two, and three standard deviations of the mean.
- Understanding standard deviation is crucial for applying the rule.
- The rule simplifies data interpretation and provides insights without complex calculations.
- The rule has wide applications across various disciplines.
The Empirical Rule: A Deep Dive
Introduction
The empirical rule, also known as the 68-95-99.7 rule, is a statistical guideline that describes the percentage of data points falling within a specific number of standard deviations from the mean in a normal distribution. Understanding this rule is fundamental for interpreting data and making informed conclusions in numerous fields. Its simplicity belies its profound impact on data analysis and interpretation.
Key Aspects
- Normal Distribution: The empirical rule exclusively applies to datasets following a normal distribution, also known as a Gaussian distribution – a bell-shaped curve symmetrical around the mean.
- Mean: The average of the dataset, representing the central tendency.
- Standard Deviation: A measure of the spread or dispersion of data around the mean. A higher standard deviation indicates greater variability.
- Z-scores: These represent the number of standard deviations a data point lies from the mean. A z-score of 1 indicates the data point is one standard deviation above the mean; a z-score of -2 indicates it's two standard deviations below the mean.
Discussion
The empirical rule states that for a normal distribution:
- Approximately 68% of the data falls within one standard deviation of the mean (µ ± σ).
- Approximately 95% of the data falls within two standard deviations of the mean (µ ± 2σ).
- Approximately 99.7% of the data falls within three standard deviations of the mean (µ ± 3σ).
This rule provides a quick assessment of data spread without complex calculations. For instance, if the average height of adult women is 162 cm with a standard deviation of 6 cm, the empirical rule suggests that roughly 68% of women have heights between 156 cm (162 - 6) and 168 cm (162 + 6). Similarly, about 95% would have heights between 150 cm and 174 cm (162 ± 2*6).
Standard Deviation: The Measure of Spread
Introduction
Standard deviation is intrinsically linked to the empirical rule. Understanding its calculation and interpretation is crucial for applying the rule effectively. Standard deviation quantifies the dispersion of data points around the mean, with a larger value indicating greater variability.
Facets
- Role: Standard deviation measures the average distance of data points from the mean.
- Example: In a dataset of exam scores, a high standard deviation indicates a wide range of scores, while a low standard deviation shows scores clustered closely around the average.
- Risks & Mitigations: Misinterpreting standard deviation can lead to inaccurate conclusions. Ensuring data normality before applying the rule mitigates this risk.
- Impacts & Implications: Standard deviation is crucial for numerous statistical analyses, including hypothesis testing and confidence intervals.
Summary
Understanding standard deviation is paramount for applying the empirical rule correctly. It provides context for interpreting the percentages of data within specific ranges around the mean. Its significance extends far beyond the empirical rule, playing a vital role in diverse statistical procedures.
Applying the Empirical Rule: Real-World Examples
Example 1: Manufacturing Quality Control
A factory produces light bulbs with a mean lifespan of 1000 hours and a standard deviation of 50 hours. Using the empirical rule:
- Approximately 68% of bulbs have lifespans between 950 and 1050 hours (1000 ± 50).
- Approximately 95% have lifespans between 900 and 1100 hours (1000 ± 2*50).
- Approximately 99.7% have lifespans between 850 and 1150 hours (1000 ± 3*50).
This information helps the manufacturer set quality control standards and predict potential product failures.
Example 2: Student Test Scores
A class's average score on a test is 75 with a standard deviation of 10. Applying the empirical rule:
- About 68% of students scored between 65 and 85 (75 ± 10).
- About 95% scored between 55 and 95 (75 ± 2*10).
- About 99.7% scored between 45 and 105 (75 ± 3*10).
This allows educators to gauge the overall class performance and identify students who may require additional support.
FAQ
Introduction
This section addresses frequently asked questions about the empirical rule.
Questions
- Q: Can the empirical rule be applied to all datasets? A: No, it only applies to data following a normal distribution.
- Q: What if my data is not normally distributed? A: Alternative methods, such as Chebyshev's inequality, can be used for non-normal distributions.
- Q: How accurate are the percentages given by the empirical rule? A: The percentages are approximations; the actual percentages may slightly vary.
- Q: Is the empirical rule useful for small datasets? A: It's more accurate with larger datasets, as the normal distribution approximation improves with sample size.
- Q: How does the empirical rule relate to z-scores? A: Z-scores represent the number of standard deviations a data point is from the mean; the empirical rule uses z-scores implicitly to define the ranges.
- Q: Where can I find more information on normal distribution? A: Statistical textbooks and online resources offer in-depth explanations of normal distributions.
Summary
The FAQs highlight the limitations and applications of the empirical rule, emphasizing the importance of data normality and understanding z-scores.
Tips for Using the Empirical Rule
Introduction
These tips will help you effectively apply the empirical rule in your data analysis.
Tips
- Verify Normality: Before applying the rule, confirm your data follows a normal distribution using histograms, Q-Q plots, or statistical tests.
- Calculate Mean and Standard Deviation: Accurately compute the mean and standard deviation of your data.
- Identify Ranges: Use the formula (µ ± σ), (µ ± 2σ), and (µ ± 3σ) to define the ranges.
- Interpret Percentages: Remember the approximate percentages (68%, 95%, 99.7%) associated with each range.
- Consider Sample Size: The rule is more accurate with larger datasets.
- Context Matters: Interpret results in the context of the specific problem.
- Use Software: Statistical software can simplify calculations and data visualization.
- Understand Limitations: The empirical rule is an approximation, and real-world data may not perfectly adhere to the normal distribution.
Summary
These tips provide a practical guide to using the empirical rule effectively and interpreting results accurately.
Summary
This exploration of the empirical rule highlighted its definition, formula, and numerous applications across various fields. Understanding this rule offers a powerful tool for quickly interpreting data and drawing meaningful conclusions about normally distributed datasets.
Closing Message
The empirical rule, although a simple concept, offers profound insights into data distribution. Its widespread applicability makes it an essential tool for anyone working with data analysis. By mastering its use, analysts can enhance their data interpretation skills and make more informed decisions.
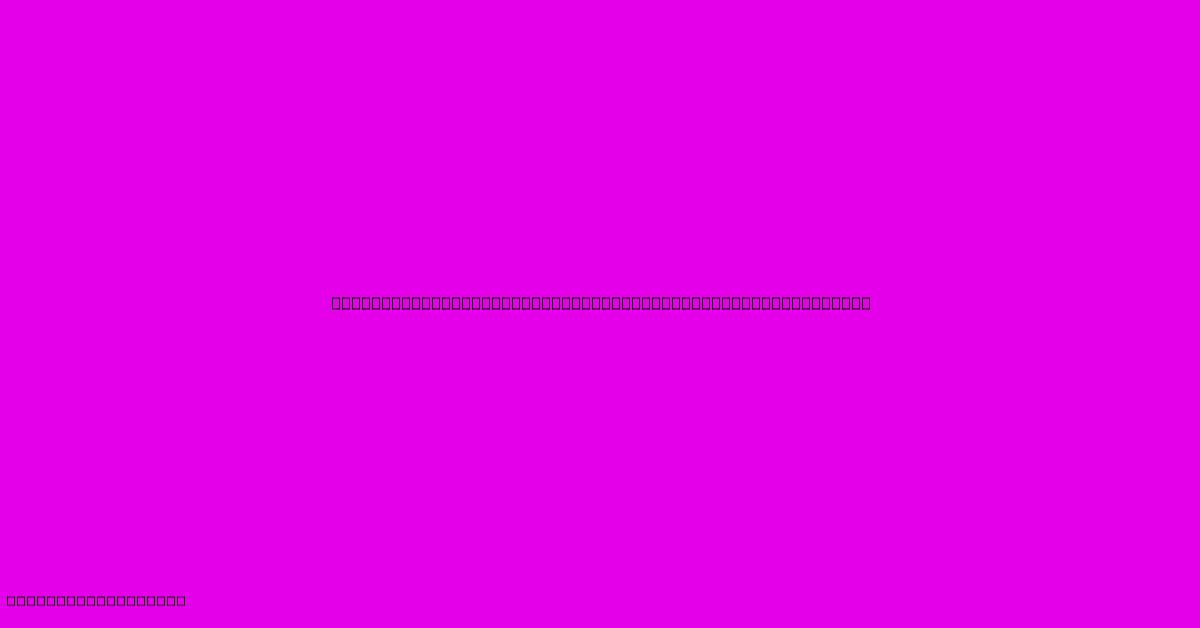
Thank you for taking the time to explore our website Empirical Rule Definition Formula Example How Its Used. We hope you find the information useful. Feel free to contact us for any questions, and don’t forget to bookmark us for future visits!
We truly appreciate your visit to explore more about Empirical Rule Definition Formula Example How Its Used. Let us know if you need further assistance. Be sure to bookmark this site and visit us again soon!
Featured Posts
-
Which Travel Insurance Covers Pregnancy
Jan 07, 2025
-
What Is The Cash Surrender Value Of Life Insurance
Jan 07, 2025
-
Euroyen Definition
Jan 07, 2025
-
Fed Speak Definition
Jan 07, 2025
-
How Much Is Homeowners Insurance Georgia
Jan 07, 2025