Endogenous Variable Definition Meaning And Examples
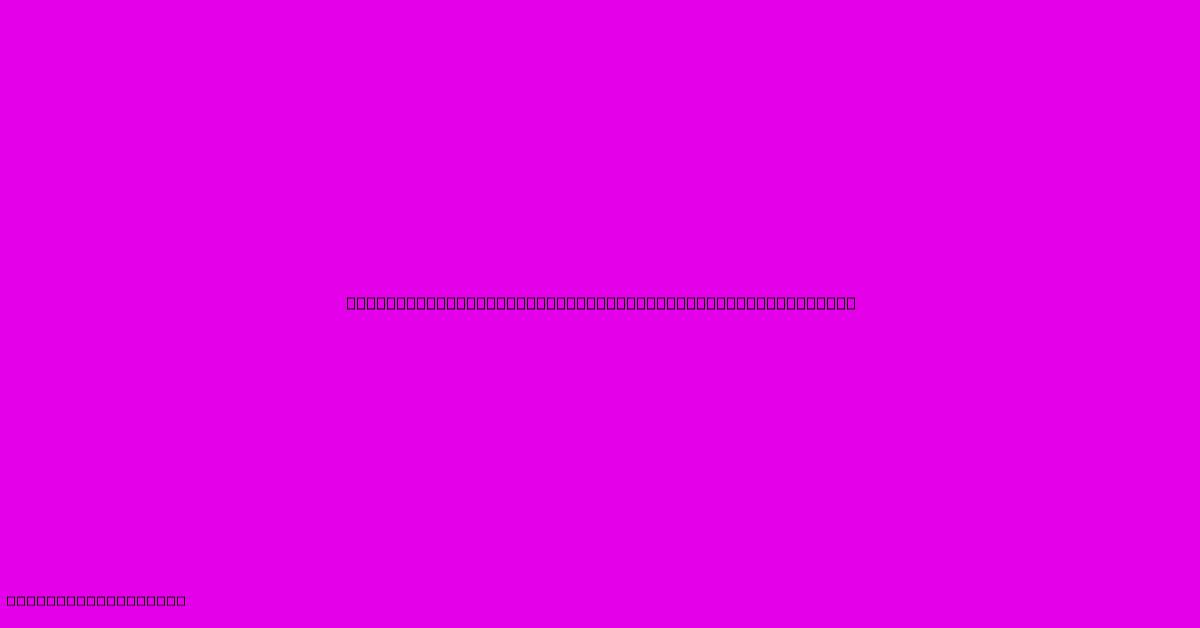
Discover more in-depth information on our site. Click the link below to dive deeper: Visit the Best Website meltwatermedia.ca. Make sure you don’t miss it!
Table of Contents
Unveiling Endogenous Variables: Definition, Meaning & Examples
Does the interconnectedness of variables in your model leave you puzzled? Understanding endogenous variables is crucial for accurate analysis. This comprehensive guide explores endogenous variables, their meaning, and practical examples across various fields.
Editor's Note: This guide to endogenous variables has been published today to provide a clear and concise understanding of this crucial statistical concept.
Importance & Summary: Endogenous variables are a cornerstone of econometrics, statistical modeling, and causal inference. Understanding their nature is essential for building robust models that accurately represent relationships between variables, avoiding spurious correlations and ensuring the reliability of research findings. This guide will define endogenous variables, explore their characteristics, explain how they differ from exogenous variables, and illustrate them with real-world examples across different disciplines. We'll delve into the implications of endogenous variables, outlining how to identify and address them in your analyses.
Analysis: The information presented in this guide is compiled from a range of authoritative sources in econometrics, statistics, and related fields. Peer-reviewed journals, academic textbooks, and reputable online resources have been consulted to ensure accuracy and completeness. The examples provided are chosen to represent diverse contexts and demonstrate the practical applications of the concept.
Key Takeaways:
- Clear definition and explanation of endogenous variables.
- Distinction between endogenous and exogenous variables.
- Real-world examples across various fields.
- Methods for identifying and handling endogenous variables.
- Practical implications for research and analysis.
Endogenous Variables: A Deep Dive
Introduction
Endogenous variables, within the context of a statistical model, are variables whose values are determined within the system being modeled. This means their values are influenced by other variables within the same model, creating a complex web of interconnected relationships. Understanding this interconnectedness is pivotal for accurate model building and interpretation. The impact of neglecting the endogenous nature of variables can lead to biased and unreliable results.
Key Aspects of Endogenous Variables
- Internal Determination: Endogenous variables are determined within the model, implying they are not independent of other variables within the same system.
- Interdependence: These variables are often correlated with other variables in the model, leading to potential problems if not appropriately handled.
- Causality Issues: The presence of endogenous variables can confound causal inference, leading to misinterpretations of cause-and-effect relationships.
Discussion
The core issue with endogenous variables stems from simultaneity or feedback loops. For instance, consider the relationship between price and quantity demanded in a market. The price influences quantity demanded (a negative relationship), but the quantity demanded also influences price (through supply and demand dynamics). This creates a simultaneous equation system, where both price and quantity are endogenous. A simple regression of quantity demanded on price would yield biased and inconsistent estimates due to this simultaneity.
Another example is the relationship between education and income. While higher education may lead to higher income, individuals with higher innate abilities might simultaneously pursue more education and earn higher incomes. The correlation between education and income is partly endogenous as it's influenced by unobserved abilities.
Subheading: Simultaneity Bias
Introduction: Simultaneity bias arises when endogenous variables are correlated with the error term in a regression model. This correlation violates the fundamental assumption of ordinary least squares (OLS) regression that the error term is uncorrelated with the regressors.
Facets:
- Role: Simultaneity bias leads to inconsistent and biased parameter estimates.
- Examples: The price-quantity example above perfectly illustrates simultaneity bias. Ignoring the simultaneity and regressing quantity on price alone would lead to biased estimates of the price elasticity of demand.
- Risks & Mitigations: The risk is inaccurate inference and misleading conclusions. Mitigations include instrumental variable (IV) techniques, two-stage least squares (2SLS), and generalized method of moments (GMM).
- Impacts & Implications: Biased estimates can lead to incorrect policy recommendations or flawed predictions.
Subheading: Omitted Variable Bias
Introduction: Omitted variable bias occurs when a relevant variable that affects both the dependent and independent variables is excluded from the model. This omitted variable becomes part of the error term, causing correlation with the included endogenous variables.
Further Analysis: Suppose we are examining the relationship between advertising expenditure and sales. If the quality of the product is omitted, a higher quality product (unobserved) might lead to both higher advertising expenditure and higher sales. The correlation between advertising and sales will be influenced by the omitted quality variable, creating an endogenous relationship.
Closing: Omitted variable bias is a serious problem leading to inaccurate inferences. Carefully considering all relevant variables and addressing potential omitted variables through techniques like including proxies or more sophisticated modeling is crucial.
Subheading: Measurement Error
Introduction: Measurement error in an independent variable can cause endogeneity. If the measured value of an independent variable differs from its true value, the error in measurement becomes part of the error term, correlating it with the independent variable.
Further Analysis: Imagine studying the effect of a company's R&D spending on its market share. If R&D spending is mismeasured (e.g., due to accounting practices or reporting biases), the error in measurement introduces endogeneity.
Closing: Minimizing measurement error through careful data collection and using more reliable measures is essential in mitigating endogeneity.
FAQ: Endogenous Variables
Introduction
This section addresses frequently asked questions about endogenous variables.
Questions:
-
Q: What is the difference between endogenous and exogenous variables? A: Endogenous variables are determined within the model, influenced by other variables within the system. Exogenous variables are determined outside the model and are independent of other variables within it.
-
Q: How can I identify endogenous variables in my model? A: Look for simultaneity, feedback loops, omitted variables, or measurement error. Statistical tests like the Durbin-Wu-Hausman test can help detect endogeneity.
-
Q: What are the consequences of ignoring endogeneity? A: Ignoring endogeneity leads to biased and inconsistent parameter estimates, inaccurate inferences, and unreliable conclusions.
-
Q: What techniques can be used to address endogeneity? A: Instrumental variable (IV) estimation, two-stage least squares (2SLS), and generalized method of moments (GMM) are common techniques.
-
Q: Are all correlated variables endogenous? A: No. Correlation does not imply causation, and not all correlated variables are endogenous. Endogeneity arises from specific causal relationships within the model.
-
Q: Can I use OLS regression if I have endogenous variables? A: OLS is inappropriate when dealing with endogenous variables as it leads to biased and inconsistent results. Alternative techniques are required.
Summary: Recognizing and addressing endogeneity is vital for the reliability of your analysis.
Tips for Handling Endogenous Variables
Introduction
This section offers practical advice for managing endogenous variables in your research.
Tips:
-
Careful Model Specification: Thoroughly consider all potential variables and their relationships. Avoid omitting relevant variables.
-
Data Quality: Ensure high-quality data to minimize measurement error.
-
Instrumental Variable Selection: If using IV techniques, carefully select instruments that are strongly correlated with the endogenous variable but uncorrelated with the error term.
-
Diagnostic Tests: Employ diagnostic tests to detect endogeneity before applying more advanced techniques.
-
Robustness Checks: Perform sensitivity analyses and robustness checks to assess the impact of different model specifications and estimation methods.
-
Consider Alternative Methods: Explore alternative modeling approaches that might be less susceptible to endogeneity problems.
-
Consult Experts: If uncertain about how to handle endogeneity, seek guidance from a statistician or econometrician.
Summary: A proactive and thorough approach can significantly reduce the impact of endogenous variables.
Summary: Endogenous Variables and Their Significance
This guide explored endogenous variables, emphasizing their definition, characteristics, and implications for various analyses. Understanding their role is paramount for accurate modeling and robust causal inference. Ignoring endogeneity can lead to misleading conclusions and erroneous policy decisions.
Closing Message: The careful consideration of endogeneity is not just a technical detail but a foundational element of rigorous research and reliable conclusions. Proactive identification and effective handling of endogenous variables are essential for accurate insights and informed decision-making across diverse fields.
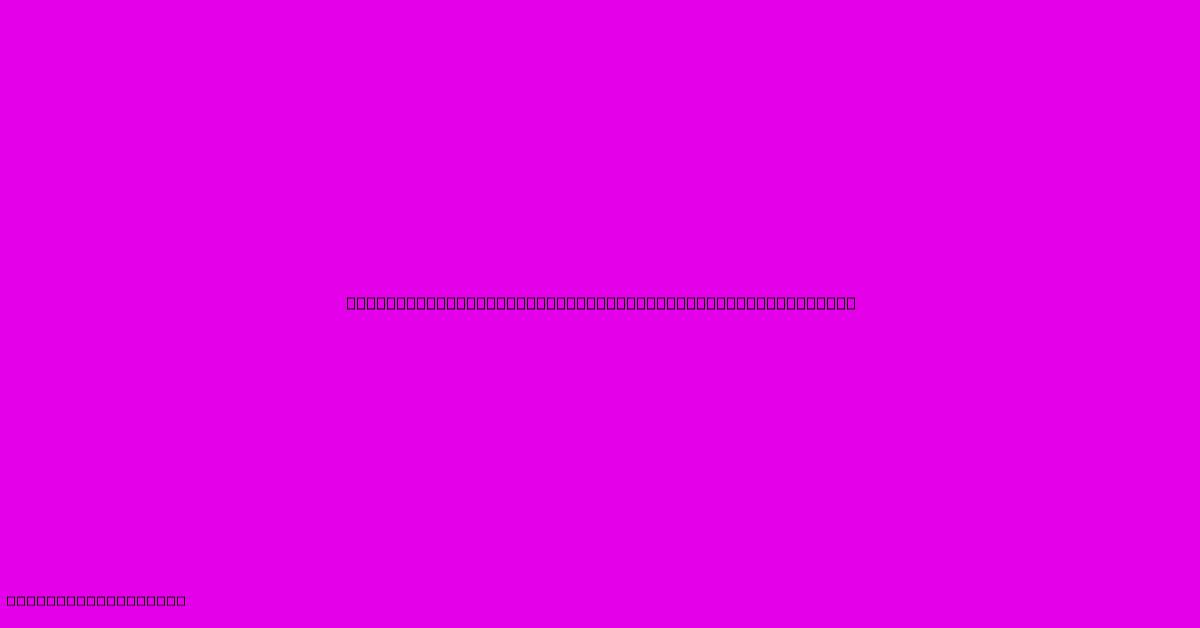
Thank you for taking the time to explore our website Endogenous Variable Definition Meaning And Examples. We hope you find the information useful. Feel free to contact us for any questions, and don’t forget to bookmark us for future visits!
We truly appreciate your visit to explore more about Endogenous Variable Definition Meaning And Examples. Let us know if you need further assistance. Be sure to bookmark this site and visit us again soon!
Featured Posts
-
How To Get My Credit Card Number
Jan 07, 2025
-
Flat Tax Definition How It Works Benefits Critics And Example
Jan 07, 2025
-
Fixed Rate Capital Securities Frcs Definition
Jan 07, 2025
-
How Long To Keep Credit Card Receipts
Jan 07, 2025
-
What Are Dividends In Insurance
Jan 07, 2025