Probable Maximum Loss Definition And How To Calculate It
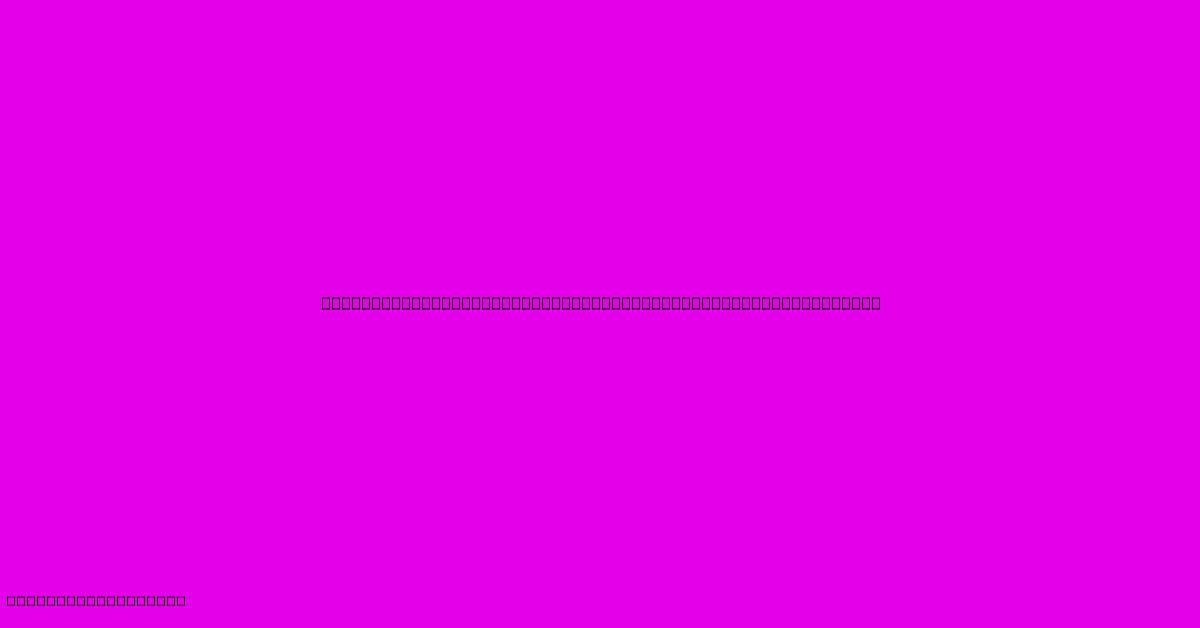
Discover more in-depth information on our site. Click the link below to dive deeper: Visit the Best Website meltwatermedia.ca. Make sure you don’t miss it!
Table of Contents
Unveiling Probable Maximum Loss (PML): Definition and Calculation Methods
Hook: What if a single catastrophic event wiped out your entire investment? Understanding Probable Maximum Loss (PML) is crucial for mitigating such devastating scenarios.
Editor's Note: This comprehensive guide to Probable Maximum Loss (PML) has been published today. It provides a detailed explanation of PML definition and calculation methods, equipping readers with the knowledge to effectively manage risk.
Importance & Summary: Probable Maximum Loss (PML) is a critical risk assessment metric used across various industries, particularly in insurance and finance. This guide will explore PML's definition, its practical applications, and different calculation methods, emphasizing its importance in strategic risk management and financial stability. Understanding PML allows businesses to adequately plan for worst-case scenarios, securing financial resilience and safeguarding against potential ruin. Key concepts covered include scenario analysis, data aggregation, statistical modeling, and loss estimation techniques.
Analysis: This guide compiles information from industry best practices, academic research papers on catastrophe modeling, and regulatory guidelines on risk assessment. The analysis focuses on providing a practical understanding of PML calculations, applicable across diverse contexts and risk profiles. The different methods presented are compared to highlight their respective strengths and weaknesses, ensuring readers select the most appropriate technique based on available data and risk characteristics.
Key Takeaways:
- PML defines the worst-case loss a company could face.
- Calculating PML involves scenario analysis and data modeling.
- Several methods exist for PML calculation, each with its advantages.
- Accurate PML estimation requires comprehensive data and expert judgment.
- Understanding PML strengthens financial resilience and planning.
Probable Maximum Loss: A Deep Dive
Introduction
Probable Maximum Loss (PML) represents the largest potential loss a company or portfolio could experience under a specified set of circumstances. It's a critical concept in risk management, helping organizations anticipate and prepare for catastrophic events. The accurate estimation of PML is vital for informed decision-making, particularly in insurance, reinsurance, and investment management. Its significance stems from its ability to quantify the potential for extreme financial losses, facilitating the development of effective risk mitigation strategies.
Key Aspects of PML
- Scenario Analysis: Identifying potential events (e.g., natural disasters, market crashes) that could lead to significant losses.
- Data Aggregation: Collecting relevant data on historical losses, asset values, and exposure to risks.
- Statistical Modeling: Utilizing statistical techniques to estimate the probability of different loss scenarios.
- Loss Estimation: Quantifying the financial impact of each scenario.
- Risk Mitigation: Developing strategies to reduce the probability or impact of potential losses.
Scenario Analysis: Identifying Potential Catastrophes
Introduction: Scenario analysis is the cornerstone of PML calculation. It involves systematically identifying and evaluating potential events that could trigger significant losses.
Facets:
- Role: Defines the potential threats and their potential impact.
- Examples: Natural catastrophes (earthquakes, hurricanes), market crashes, pandemics, cyberattacks, operational failures.
- Risks & Mitigations: Identifying inherent vulnerabilities and implementing preventative measures (e.g., diversification, insurance).
- Impacts & Implications: Analyzing the financial and operational consequences of each scenario.
Summary: The thoroughness of scenario analysis directly impacts the accuracy of PML estimations. Failing to consider all relevant scenarios can lead to significant underestimation of potential losses.
Data Aggregation: Gathering Essential Information
Introduction: Accurate data is essential for reliable PML calculation. This stage involves collecting information from various sources.
Facets:
- Historical Loss Data: Examining past loss events to establish frequency and severity.
- Asset Values: Determining the value of assets at risk.
- Exposure Analysis: Assessing the extent to which assets are exposed to specific risks.
- Market Data: Gathering data on market volatility and correlations to estimate potential losses.
- External Data: Utilizing external sources such as weather patterns, seismic activity data, or economic indicators to build more comprehensive risk models.
Summary: Comprehensive data aggregation ensures that the PML calculation considers all relevant factors and minimizes bias. The quality and quantity of data significantly affect the reliability of the final PML estimate.
Statistical Modeling: Estimating Probabilities
Introduction: Statistical modeling plays a crucial role in PML calculation. It allows us to quantify the likelihood of various loss scenarios.
Further Analysis: Various statistical models can be applied, including Monte Carlo simulations, which allow for the consideration of numerous variables and their interactions to generate a distribution of possible loss outcomes. Other methods include regression analysis, which can reveal relationships between different factors influencing loss severity.
Closing: The choice of statistical model depends on the available data and the complexity of the risks involved. The model must accurately reflect the inherent uncertainties in predicting future events.
Loss Estimation: Quantifying Financial Impacts
Introduction: This stage involves translating the probabilities from the statistical models into actual financial losses.
Further Analysis: Techniques range from simple multiplication of asset values and probabilities to complex algorithms that consider correlations between different loss events. Sensitivity analysis, where inputs are varied to observe their effects on the PML, further improves the model's robustness.
Closing: Accurate loss estimation relies on the quality of both the statistical model and the underlying assumptions about the value of assets and their exposure to risk.
PML Calculation Methods: A Comparative Overview
Several methods exist for calculating PML, each with its own strengths and weaknesses. The optimal method depends on the specific application and the availability of data.
- Loss Ratio Method: This method utilizes historical loss ratios to project potential losses under different scenarios. It is straightforward but relies on the stability of past loss patterns.
- Monte Carlo Simulation: This advanced statistical method generates thousands of potential scenarios, offering a broad range of potential outcomes and a better understanding of the uncertainty around the PML estimate. It requires significant computational power and extensive data.
- Expert Judgment: This method incorporates the knowledge and experience of industry experts to estimate potential losses. While valuable, it is subjective and prone to bias.
- Industry-Specific Models: Some industries have developed sophisticated models tailored to their specific risks (e.g., catastrophe models for insurance). These models often require specialized software and expertise.
FAQ
Introduction: This section addresses frequently asked questions about PML.
Questions:
-
Q: What is the difference between PML and Maximum Possible Loss (MPL)? A: PML represents the most likely worst-case scenario, while MPL represents the absolute worst-case scenario, regardless of probability.
-
Q: How often should PML be recalculated? A: PML should be updated regularly, reflecting changes in asset values, risk exposures, and the broader economic environment.
-
Q: What are the limitations of PML calculations? A: PML estimates are inherently probabilistic and subject to uncertainty. They are not guaranteed predictions of future events.
-
Q: How is PML used in insurance pricing? A: Insurers use PML estimates to determine appropriate premiums and set reinsurance limits.
-
Q: Can PML be used for risk mitigation planning? A: Yes, PML calculations highlight areas of vulnerability, allowing companies to develop targeted risk mitigation strategies.
-
Q: How does the quality of data affect PML accuracy? A: Inaccurate or incomplete data can significantly distort PML estimates, leading to unreliable results.
Summary: Understanding the nuances of PML calculation is vital for making informed risk management decisions. The use of sophisticated models and robust data increases the reliability of PML estimations.
Tips for Effective PML Calculation
Introduction: This section provides practical tips for accurately calculating PML.
Tips:
- Comprehensive Data Collection: Gather as much relevant data as possible from multiple sources.
- Robust Scenario Analysis: Consider a wide range of potential events, including low-probability, high-impact scenarios.
- Appropriate Statistical Modeling: Choose the statistical model that best fits the available data and the complexity of the risks.
- Regular Updates: Reassess PML regularly to account for changes in risks and asset values.
- Expert Consultation: Seek advice from qualified experts to ensure the accuracy and reliability of your PML estimation.
- Transparency and Documentation: Clearly document the methodology, assumptions, and results of your PML calculations.
- Sensitivity Analysis: Perform sensitivity analysis to determine the impact of uncertainties in input data on the final PML estimate.
Summary: By following these tips, organizations can significantly improve the accuracy and effectiveness of their PML calculations.
Summary of Probable Maximum Loss
This guide has provided a comprehensive overview of Probable Maximum Loss (PML), a critical metric for risk assessment and management. It explores the definition, calculation methods, and practical applications of PML, emphasizing its importance in ensuring financial resilience. The importance of accurate data, robust statistical modeling, and thorough scenario analysis cannot be overstated.
Closing Message
Understanding and calculating PML is not just a technical exercise; it's a crucial step in building a more robust and sustainable business. By effectively managing the potential for extreme losses, organizations can safeguard their financial future and navigate uncertainties with greater confidence. Continuous monitoring, refinement, and adaptation of the PML calculation process are essential to maintain its relevance and accuracy in the ever-changing risk landscape.
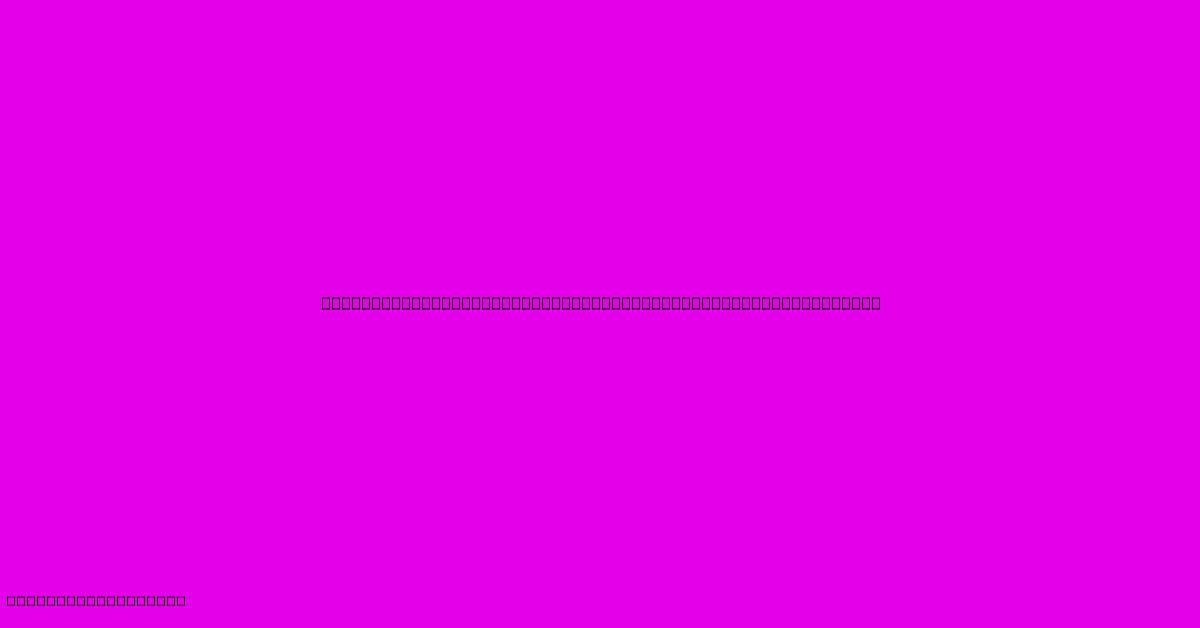
Thank you for taking the time to explore our website Probable Maximum Loss Definition And How To Calculate It. We hope you find the information useful. Feel free to contact us for any questions, and don’t forget to bookmark us for future visits!
We truly appreciate your visit to explore more about Probable Maximum Loss Definition And How To Calculate It. Let us know if you need further assistance. Be sure to bookmark this site and visit us again soon!
Featured Posts
-
Production Efficiency Defined With Ppf Curve Graph And Formula
Jan 11, 2025
-
Series 52 Definition
Jan 11, 2025
-
What Is Financial Budgeting
Jan 11, 2025
-
What Credit Do You Need To Rent A House
Jan 11, 2025
-
Property Management Definition Roles Types And Duties
Jan 11, 2025