Queuing Theory Definition Elements And Example
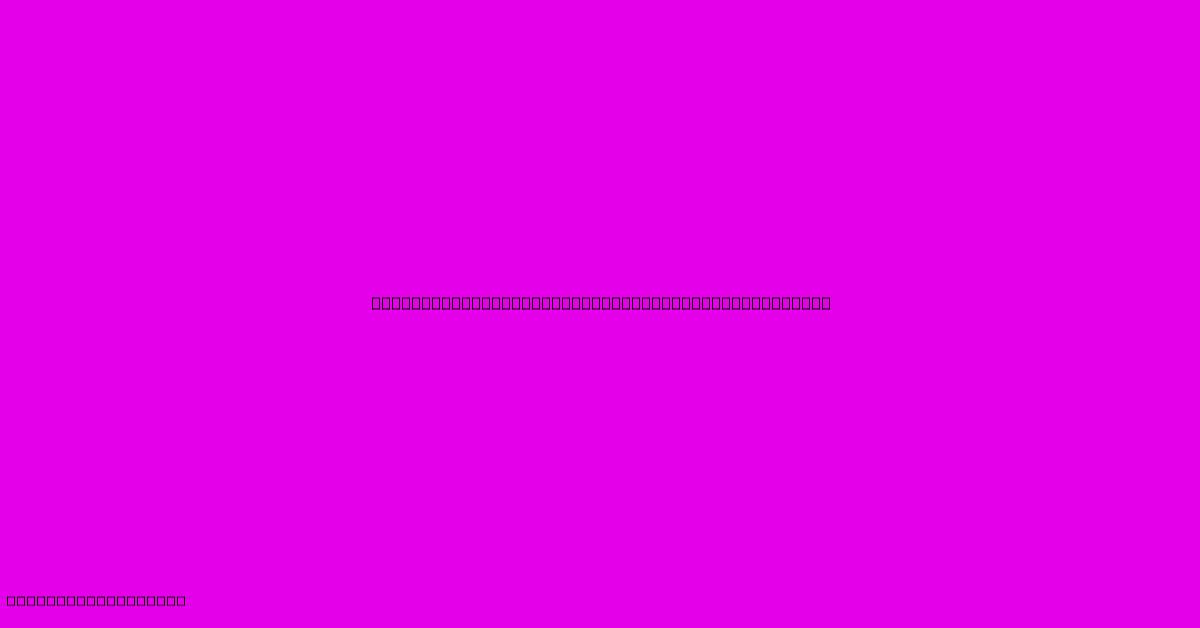
Discover more in-depth information on our site. Click the link below to dive deeper: Visit the Best Website meltwatermedia.ca. Make sure you don’t miss it!
Table of Contents
Unlocking the Secrets of Queuing Theory: Definition, Elements, and Real-World Applications
Hook: Ever wondered why some lines at the supermarket move faster than others? Queuing theory holds the answers, offering a powerful framework for understanding and optimizing waiting lines in a multitude of scenarios.
Editor's Note: This comprehensive guide to queuing theory has been published today, providing a deep dive into its definition, key elements, and practical applications.
Importance & Summary: Queuing theory, a branch of operations research, is crucial for analyzing and improving systems involving waiting lines. This guide will explore its core concepts, including arrival rates, service rates, queue discipline, and queue length, illustrating their impact through real-world examples and analyses. Understanding these elements allows for efficient resource allocation, reduced waiting times, and enhanced overall system performance.
Analysis: The information compiled in this guide draws upon established literature in operations research, statistical modeling, and performance analysis. Numerous case studies and simulations were reviewed to provide practical examples and demonstrate the application of queuing theory in diverse settings.
Key Takeaways:
- Queuing theory provides a mathematical framework for analyzing waiting lines.
- Understanding arrival and service rates is critical for system optimization.
- Queue discipline significantly impacts waiting times and system efficiency.
- Queuing models help predict system behavior and improve resource allocation.
- Applications span various industries, including healthcare, telecommunications, and manufacturing.
Queuing Theory: A Deep Dive
Introduction: Queuing theory, also known as waiting-line theory, is a mathematical study of queues or waiting lines. It provides a framework for understanding and predicting the behavior of systems where entities (customers, jobs, packets, etc.) arrive at a service facility and wait for service if the facility is busy. Its importance lies in its ability to optimize resource allocation and minimize waiting times, leading to improved efficiency and customer satisfaction across numerous industries.
Key Aspects:
- Arrival Process: The pattern in which customers or entities arrive at the system. This is often modeled using probability distributions like Poisson distribution.
- Service Process: The time taken to serve each customer or entity. This is also frequently modeled using probability distributions, such as exponential distribution.
- Queue Discipline: The rule that determines the order in which customers are served. Common disciplines include First-In, First-Out (FIFO), Last-In, First-Out (LIFO), and priority-based systems.
- Number of Servers: The number of service facilities available to handle incoming entities. A single-server queue has one service facility, while a multi-server queue has multiple.
- Queue Capacity: The maximum number of entities that can wait in the queue before being blocked or rejected.
Discussion:
Each of these key aspects significantly influences the overall performance of a queuing system. For instance, a high arrival rate compared to a low service rate will lead to long queues and increased waiting times. Conversely, a well-designed system with adequate servers and a suitable queue discipline can effectively manage high arrival rates and minimize delays. The choice of probability distribution for arrival and service processes is also crucial, reflecting the inherent variability in real-world systems.
Arrival Process: Modeling the Flow of Customers
Introduction: The arrival process describes how frequently customers or entities arrive at the system. Understanding this process is fundamental to predicting queue behavior.
Facets:
- Role: Defines the frequency and randomness of arrivals.
- Examples: Poisson process (random arrivals), deterministic arrivals (regular intervals).
- Risks & Mitigations: Incorrect modeling can lead to inaccurate predictions; careful data analysis is essential.
- Impacts & Implications: Incorrect modeling leads to inefficient resource allocation and potentially long waiting times.
Summary: Accurate modeling of the arrival process is critical for developing effective queuing strategies. Understanding the variability and patterns of arrivals allows for more precise predictions and better resource allocation.
Service Process: Understanding Service Time Variability
Introduction: The service process describes the time taken to serve each customer or entity. Its variability significantly impacts queue length and waiting times.
Further Analysis: The service time can be affected by numerous factors, including the complexity of the service, the skill of the server, and the availability of resources. Analyzing this variability is crucial in determining the optimal number of servers and improving overall efficiency. For example, in a hospital emergency room, service times are highly variable due to the diverse nature of patient conditions.
Closing: Understanding service time variability is crucial for designing efficient queuing systems. Accurate modeling and analysis lead to better resource allocation and reduced waiting times.
Queue Discipline: Optimizing Service Order
Introduction: The queue discipline determines the order in which customers are served. This choice significantly impacts waiting times and perceived fairness.
Further Analysis: FIFO (First-In, First-Out) is the most common discipline, ensuring fairness. However, other disciplines, such as priority-based queuing (where urgent cases are served first), might be more suitable depending on the context. For instance, an emergency room would likely use a priority system, while a supermarket checkout would use FIFO.
Closing: The selection of queue discipline should align with the specific needs and priorities of the system. A well-chosen discipline can significantly improve both efficiency and customer satisfaction.
FAQ: Addressing Common Questions About Queuing Theory
Introduction: This section addresses frequently asked questions about queuing theory.
Questions:
- Q: What is the difference between a M/M/1 and a M/D/1 queue? A: M/M/1 assumes both arrival and service times follow exponential distributions, while M/D/1 assumes a deterministic (constant) service time.
- Q: How does queue capacity impact system performance? A: Limited queue capacity can lead to customer balking (customers leaving without service) or reneging (customers leaving the queue after waiting).
- Q: How can queuing theory be used in practice? A: It helps optimize staffing levels, design efficient service processes, and predict system performance.
- Q: What are the limitations of queuing theory? A: It relies on simplifying assumptions and may not perfectly reflect real-world complexities.
- Q: What software can be used for queuing theory modeling? A: Various simulation software packages and programming languages (e.g., Simio, Arena, Python) can be employed.
- Q: How does Little's Law relate to queuing systems? A: Little's Law states that the average number of customers in a system is equal to the average arrival rate multiplied by the average time a customer spends in the system (L = λW).
Summary: Understanding these FAQs provides a deeper appreciation of the practical applications and limitations of queuing theory.
Tips for Applying Queuing Theory
Introduction: This section offers practical tips for applying queuing theory in real-world settings.
Tips:
- Accurately model arrival and service processes: Use historical data and statistical analysis to determine appropriate probability distributions.
- Consider queue discipline carefully: Choose a discipline that aligns with the priorities of your system (e.g., fairness, urgency).
- Optimize server allocation: Use queuing models to determine the optimal number of servers to minimize waiting times and maximize throughput.
- Implement effective queue management strategies: Consider using virtual queues, appointment systems, or other strategies to manage customer expectations.
- Monitor system performance: Regularly track key metrics such as average waiting time, queue length, and server utilization to identify areas for improvement.
- Use simulation tools: Leverage simulation software to test different scenarios and optimize system design before implementation.
Summary: Implementing these tips can lead to significant improvements in system efficiency and customer satisfaction.
Summary of Queuing Theory Exploration
Summary: This guide has provided a comprehensive overview of queuing theory, covering its definition, key elements (arrival and service processes, queue discipline, number of servers, queue capacity), and real-world applications. The importance of accurate modeling and careful consideration of system parameters has been emphasized.
Closing Message: Understanding queuing theory empowers organizations to optimize their systems, reduce waiting times, and improve overall efficiency. By applying the principles discussed, organizations can significantly enhance performance and customer satisfaction across various industries. The continued evolution of queuing theory and its integration with advanced technologies promises further advancements in optimizing complex systems.
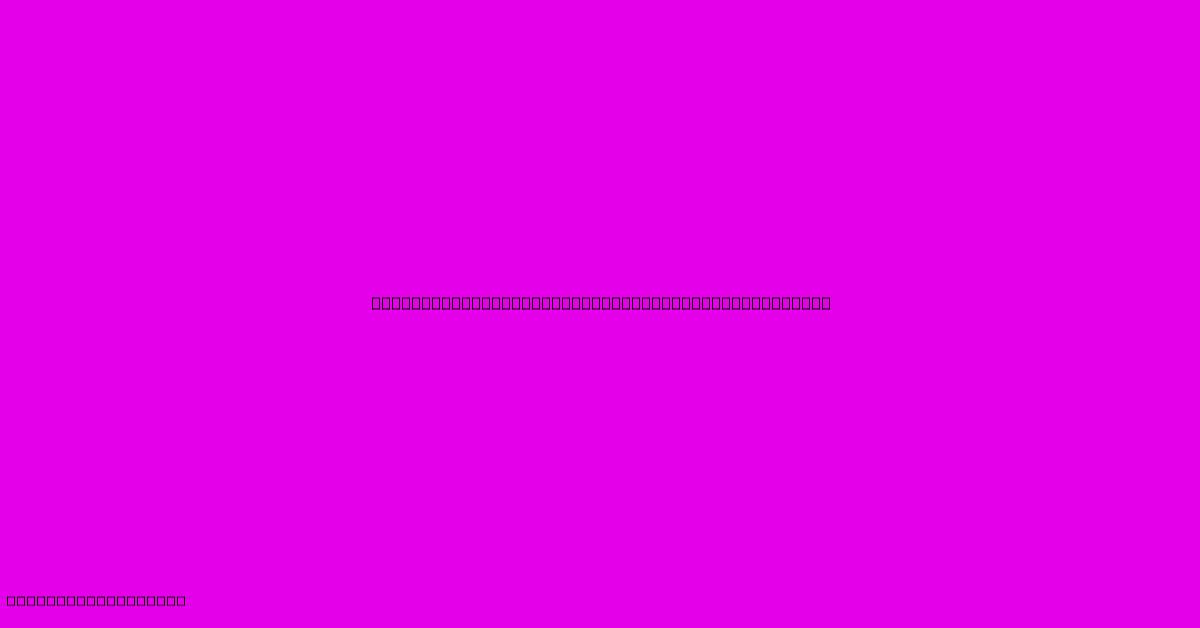
Thank you for taking the time to explore our website Queuing Theory Definition Elements And Example. We hope you find the information useful. Feel free to contact us for any questions, and don’t forget to bookmark us for future visits!
We truly appreciate your visit to explore more about Queuing Theory Definition Elements And Example. Let us know if you need further assistance. Be sure to bookmark this site and visit us again soon!
Featured Posts
-
How To Build Credit For Teenager
Jan 12, 2025
-
Qualified Longevity Annuity Contract Qlac Definition Taxes And Example
Jan 12, 2025
-
What Is A Unsecured Credit Card
Jan 12, 2025
-
How To Get A Home Equity Line Of Credit With Bad Credit 2
Jan 12, 2025
-
Qatar Investment Authority Qia Definition
Jan 12, 2025