Runs Test Definition Types Uses And Benefits
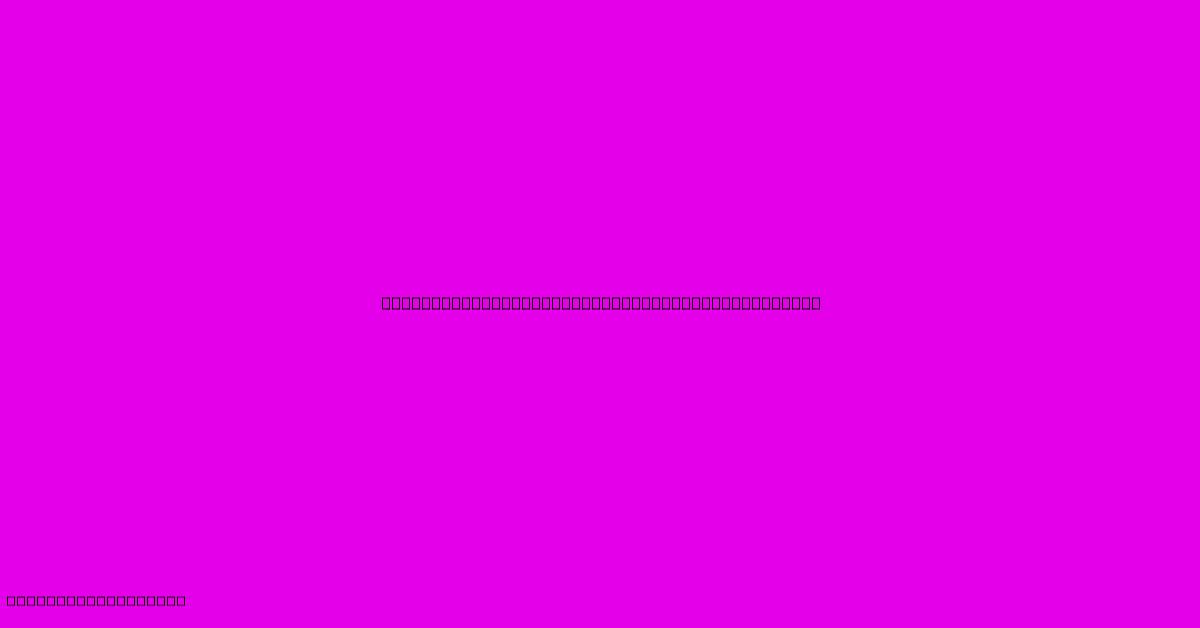
Discover more in-depth information on our site. Click the link below to dive deeper: Visit the Best Website meltwatermedia.ca. Make sure you don’t miss it!
Table of Contents
Unveiling the Runs Test: A Deep Dive into Definition, Types, Uses, and Benefits
What is a runs test, and why is it a powerful statistical tool? This comprehensive guide delves into the intricacies of the runs test, revealing its capabilities and applications across various fields.
Editor's Note: This detailed exploration of the runs test has been published today, providing a comprehensive understanding of its definition, types, uses, and benefits.
Importance & Summary: The runs test is a non-parametric statistical method used to determine whether a data sequence is random. It assesses the randomness of data by analyzing the number of "runs" – consecutive sequences of similar values – within a dataset. Understanding and applying this test is crucial in diverse fields, from quality control and finance to medicine and climatology, to identify patterns and anomalies indicative of non-randomness. This guide will cover the core definition, various types of runs tests, practical applications, and the significant benefits they offer in data analysis.
Analysis: This article synthesizes information from leading statistical textbooks, academic papers, and industry best practices. The analysis focuses on providing clear, concise explanations and real-world examples to illustrate the utility of runs tests, enhancing reader comprehension and practical application.
Key Takeaways:
- The runs test assesses the randomness of data sequences.
- Several types of runs tests exist, tailored to different data characteristics.
- Runs tests are valuable in detecting patterns and anomalies.
- Applications span diverse fields, from quality control to finance.
- Interpreting results requires understanding p-values and significance levels.
Runs Test: Definition and Significance
A runs test, also known as a run test for randomness, is a statistical technique used to examine the randomness of a sequence of data. It accomplishes this by analyzing the number of "runs" present in the sequence. A run is defined as a consecutive series of identical observations or values. The underlying principle is that a truly random sequence should exhibit a relatively even distribution of runs, neither too few nor too many. A significant deviation from the expected number of runs indicates a potential lack of randomness, suggesting the presence of trends, cycles, or other systematic patterns.
Types of Runs Tests
Several variations of the runs test exist, each designed to accommodate different types of data:
1. Runs Test for Attributes (Dichotomous Data):
This test is applied to binary data, sequences consisting of only two distinct categories (e.g., heads/tails, pass/fail, male/female). The test focuses on the number of runs formed by the alternation between these two categories. For example, in a sequence of coin tosses (H = Heads, T = Tails), HTHHTHT has 4 runs, whereas HHHHHHTTTTT has only 2 runs. A significantly low or high number of runs suggests non-randomness.
2. Runs Test for Numerical Data:
This version is used for continuous or ordinal data. The data is first divided into two groups based on a median value or a specified threshold. Runs are then counted based on the alternation between values above and below this threshold. This transformation allows the application of the run test's principle to numerical sequences.
3. Wald-Wolfowitz Runs Test:
This is a specific type of runs test often used for comparing two independent samples. It examines the runs formed by the intermingling of observations from the two samples when they are combined and ordered. A significant deviation from the expected number of runs can suggest a difference in the underlying distributions of the two samples.
Applications of Runs Tests
The versatility of the runs test makes it applicable across a broad range of disciplines:
1. Quality Control:
In manufacturing processes, the runs test helps assess the consistency of production. A significant deviation from randomness in a sequence of quality measurements (e.g., product defects) can indicate a problem in the production process.
2. Financial Markets:
Runs tests are used to analyze stock price movements, exchange rates, or other financial time series. A non-random pattern could suggest market manipulation, predictable trends, or the inefficiency of a particular market segment.
3. Meteorology and Climatology:
In climatological studies, runs tests can detect patterns in weather data (e.g., temperature, rainfall). A statistically significant deviation from randomness may reveal climate cycles or unusual weather events.
4. Medical Research:
In clinical trials or epidemiological studies, runs tests can help assess the randomness of treatment assignments or the temporal patterns of disease occurrence. Non-randomness might indicate biases in the study design or underlying trends in disease incidence.
5. Random Number Generation:
The runs test is a crucial tool for validating random number generators (RNGs). A reliable RNG should produce sequences that pass the runs test, indicating the absence of predictable patterns in the generated numbers. This is essential for various simulations and statistical modeling applications.
Benefits of Using Runs Tests
The advantages of employing runs tests include:
- Non-parametric nature: Runs tests don't require assumptions about the underlying data distribution, making them suitable for a wider range of data types.
- Simplicity and ease of interpretation: The test is relatively straightforward to understand and apply, even with limited statistical expertise.
- Sensitivity to different types of non-randomness: Runs tests can detect various patterns of non-randomness, including trends, cycles, and clustering.
- Wide applicability: Its use extends across various disciplines and data types, showcasing its versatility.
- Complementary to other tests: Runs tests can be used in conjunction with other statistical methods to provide a more comprehensive assessment of data randomness.
Subheading: Conducting a Runs Test (Example - Attributes)
Let's consider a hypothetical example illustrating a runs test for attributes. Suppose a coin is tossed 20 times, resulting in the following sequence: HTHTHTHHHTHTTTHHTHT.
-
Count the number of runs: There are 12 runs in this sequence.
-
Calculate the expected number of runs: For a sequence of n trials with n1 occurrences of one attribute and n2 occurrences of the other, the expected number of runs (E) is:
E = 2n1n2 / (n1 + n2) + 1
In our example, n1 (number of heads) = 11, n2 (number of tails) = 9, and n = 20. Therefore:
E = (2 * 11 * 9) / (11 + 9) + 1 = 10.9
-
Calculate the variance of the number of runs:
Variance (V) = 2n1n2(2n1n2 - n1 - n2) / [(n1 + n2)²(n1 + n2 -1)]
V = 2 * 11 * 9 (2 * 11 * 9 – 11 – 9) / [(11 + 9)² (11 + 9 – 1)] ≈ 4.7
-
Calculate the Z-score:
Z = (Observed runs - E) / √V = (12 - 10.9) / √4.7 ≈ 0.5
-
Determine significance: Compare the calculated Z-score to the critical Z-value for a chosen significance level (e.g., 0.05). If the absolute value of the Z-score exceeds the critical Z-value, the null hypothesis (that the data is random) is rejected.
In this case, the Z-score of 0.5 does not exceed the critical Z-value (approximately 1.96 for a two-tailed test at α = 0.05), so the data does not show significant evidence of non-randomness.
Subheading: Interpreting Runs Test Results
The outcome of a runs test is usually interpreted using a p-value. The p-value represents the probability of observing the obtained number of runs (or a more extreme value) if the data were truly random. A low p-value (typically below a significance level, such as 0.05) indicates that the observed number of runs is unlikely to have occurred by chance, suggesting a lack of randomness in the data.
FAQ
Q1: What are the assumptions of the runs test?
A1: The runs test's primary assumption is that the observations are independent. It doesn't require specific distributional assumptions about the data, making it a non-parametric test.
Q2: Can the runs test detect all types of non-randomness?
A2: While sensitive to many types, it may not detect all forms of non-randomness, especially complex or subtle patterns.
Q3: How do I choose between different types of runs tests?
A3: The choice depends on the nature of the data. Use the runs test for attributes for binary data and the runs test for numerical data for continuous or ordinal data.
Q4: What if my p-value is close to the significance level?
A4: A p-value near the significance level suggests marginal evidence against randomness. Consider further analysis or additional data to reach a firm conclusion.
Q5: Are there any limitations to using the runs test?
A5: The test might be less powerful in detecting non-randomness in short sequences. It’s important to have a reasonably large sample size for reliable results.
Q6: Can I use the runs test with time series data?
A6: Yes, it’s frequently used to analyze time series data to identify trends or cyclical patterns. However, remember to consider the potential autocorrelation in time series data.
Tips for Effective Runs Test Application
- Ensure the data meets the assumptions of the test.
- Select the appropriate type of runs test based on data characteristics.
- Choose an appropriate significance level (α).
- Consider using other statistical tests alongside the runs test for comprehensive analysis.
- Interpret results cautiously, considering the context and limitations of the test.
Summary
The runs test offers a powerful and versatile approach to assessing the randomness of data sequences. Its simplicity, non-parametric nature, and broad applicability make it an invaluable tool across diverse fields. However, understanding its limitations and using it in conjunction with other statistical techniques is crucial for robust data analysis.
Closing Message
The runs test provides a crucial diagnostic tool for evaluating randomness, leading to more informed decision-making in various sectors. By understanding its nuances and benefits, researchers and practitioners can leverage this powerful statistical method effectively. Continued exploration of advanced variations and applications promises to enhance its role in modern data analysis.
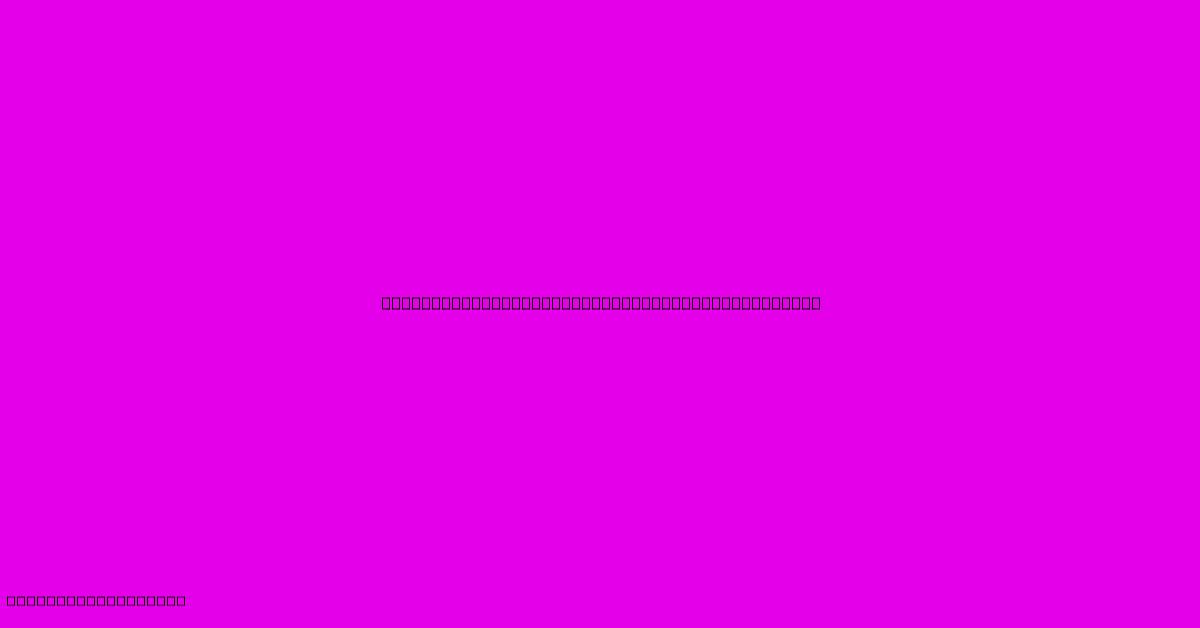
Thank you for taking the time to explore our website Runs Test Definition Types Uses And Benefits. We hope you find the information useful. Feel free to contact us for any questions, and don’t forget to bookmark us for future visits!
We truly appreciate your visit to explore more about Runs Test Definition Types Uses And Benefits. Let us know if you need further assistance. Be sure to bookmark this site and visit us again soon!
Featured Posts
-
Where Did The Money Come From For Oxbow Nd Flood Buyouts
Jan 10, 2025
-
Who Is The Best Retirement Planning Company
Jan 10, 2025
-
Section 16 Definition And Sec Filing Requirements
Jan 10, 2025
-
Regulatory Capture Definition With Examples
Jan 10, 2025
-
Roll In Definition
Jan 10, 2025