Sample Distribution Definition How Its Used With An Example
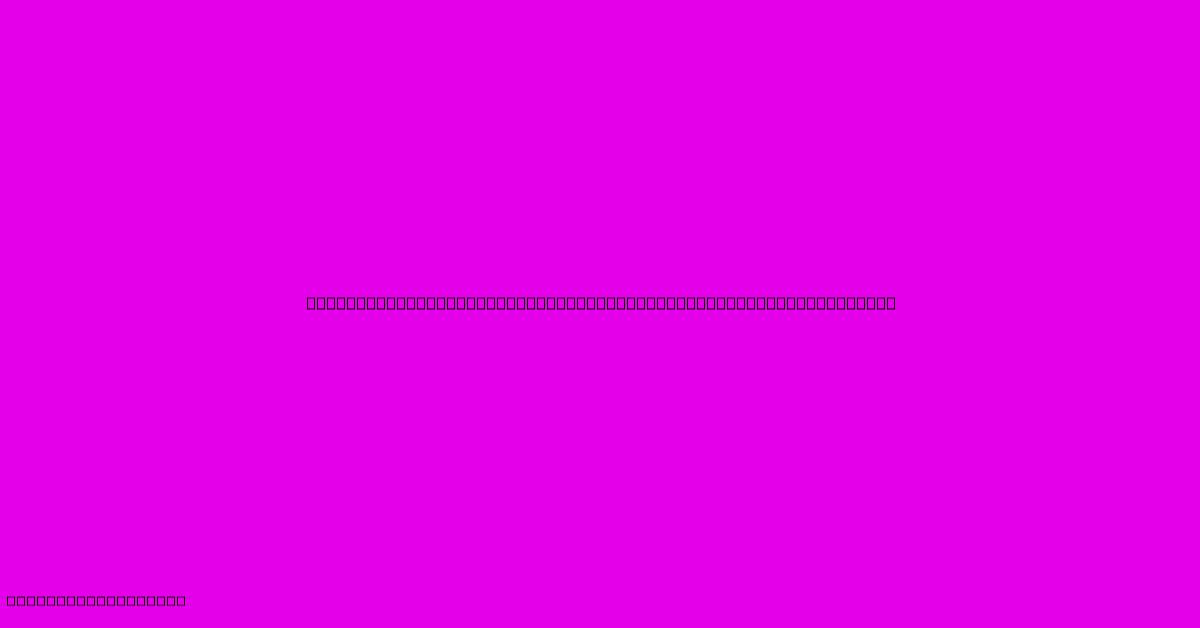
Discover more in-depth information on our site. Click the link below to dive deeper: Visit the Best Website meltwatermedia.ca. Make sure you don’t miss it!
Table of Contents
Unveiling the Power of Sample Distribution: A Comprehensive Guide
Hook: Ever wondered how researchers draw conclusions about vast populations based on limited data? The answer lies in understanding sample distribution—a fundamental concept in statistics that unlocks powerful insights.
Editor's Note: This comprehensive guide to sample distribution was published today. It explores the definition, applications, and practical implications of this crucial statistical tool.
Importance & Summary: Sample distribution is critical in inferential statistics, allowing researchers to make inferences about a population based on a representative sample. Understanding its properties, particularly the central limit theorem, is essential for accurate data analysis and informed decision-making across numerous fields, from market research to healthcare. This guide will delve into the definition, practical applications, and illustrative examples of sample distribution.
Analysis: This guide synthesizes information from various statistical textbooks, research papers, and online resources to provide a clear and concise explanation of sample distribution. Emphasis is placed on providing practical applications and examples to enhance reader comprehension and retention.
Key Takeaways:
- Sample distribution describes the probability distribution of a statistic (e.g., sample mean) obtained from multiple samples drawn from a population.
- Understanding sample distribution is crucial for making inferences about population parameters.
- The central limit theorem plays a pivotal role in simplifying the analysis of sample distributions.
- Sample distribution helps in hypothesis testing and constructing confidence intervals.
- Proper sampling techniques are crucial for obtaining a representative sample distribution.
Sample Distribution: A Deep Dive
What is Sample Distribution?
Sample distribution refers to the probability distribution of a statistic obtained from a large number of samples drawn from a population. Unlike the population distribution which encompasses all data points within a population, the sample distribution focuses on the distribution of a particular statistic (like the mean, median, or standard deviation) calculated from numerous random samples of a fixed size. This provides a framework for understanding how this statistic behaves across multiple samples, which, in turn, enables inferences about the population parameter it estimates.
Key Aspects of Sample Distribution:
- Sample Size (n): The number of observations in each sample significantly influences the shape and characteristics of the sample distribution. Larger sample sizes generally lead to a sample distribution that more closely resembles a normal distribution, regardless of the shape of the population distribution.
- Statistic of Interest: The specific statistic being analyzed (e.g., sample mean, sample variance) determines the form and properties of the sample distribution.
- Population Distribution: The underlying population distribution from which the samples are drawn also impacts the shape of the sample distribution, although its effect diminishes with larger sample sizes.
The Central Limit Theorem (CLT): A Cornerstone
The CLT is a fundamental concept in statistics that greatly simplifies the study of sample distributions. It states that the distribution of sample means (or other statistics, under certain conditions) will approach a normal distribution as the sample size increases, irrespective of the shape of the population distribution. This is crucial because the normal distribution possesses well-defined properties that allow for straightforward statistical analysis.
The CLT has several important implications:
- It allows for the use of normal distribution-based methods even when the population distribution is unknown or non-normal, provided that the sample size is sufficiently large (generally considered to be at least 30).
- It simplifies hypothesis testing and the construction of confidence intervals by providing a known distribution for the sample statistic.
- It underpins many statistical procedures commonly employed in various fields.
Applications of Sample Distribution
Sample distribution finds extensive applications in numerous fields, including:
- Market Research: Determining consumer preferences, product demand, and brand perception using sample data.
- Healthcare: Assessing the effectiveness of medical treatments, monitoring disease prevalence, and understanding patient outcomes.
- Quality Control: Monitoring manufacturing processes, ensuring product consistency, and detecting defects.
- Environmental Science: Studying pollution levels, assessing biodiversity, and predicting climate change impacts.
- Social Sciences: Conducting surveys, analyzing social behaviors, and evaluating policy effectiveness.
Example: Analyzing Average Income
Let's illustrate sample distribution with an example involving average income. Suppose a researcher wants to estimate the average annual income of residents in a large city. Due to the impracticality of surveying every resident, the researcher takes multiple random samples of 50 residents each. For each sample, they calculate the mean annual income. The distribution of these sample means constitutes the sample distribution of the mean income.
Sample Mean Analysis:
- Data Collection: The researcher gathers multiple samples of 50 residents.
- Mean Calculation: The mean income is calculated for each of these samples.
- Distribution Creation: The calculated mean incomes are plotted to create a frequency distribution. This visual representation shows the distribution of sample means.
- Inference: The CLT implies that if enough samples are taken, the distribution of these sample means will closely approximate a normal distribution. This normal distribution can then be used to estimate the population mean income with a specific level of confidence (e.g., 95% confidence interval). The standard error of the mean, which is the standard deviation of the sample means, quantifies the uncertainty surrounding the estimated population mean.
Exploring Facets of the Example:
- Role of Sample Size: If smaller samples (e.g., 10 residents) were used, the sample distribution might not closely resemble a normal distribution, even if the population distribution were normal.
- Impact of Population Distribution: The shape of the overall income distribution in the city will slightly influence the sample distribution, particularly with smaller sample sizes. However, as per the CLT, the effect becomes minimal with larger samples.
- Practical Implications: Understanding the sample distribution enables the researcher to create confidence intervals, making statements like "We are 95% confident that the average annual income in the city lies between X and Y dollars." This showcases the practical utility of sample distribution in making reliable inferences from limited data.
Frequently Asked Questions (FAQ)
Introduction: This section addresses common questions about sample distribution.
Questions:
-
Q: What is the difference between population distribution and sample distribution? A: Population distribution represents the entire population's data, while sample distribution depicts the distribution of a statistic from multiple samples drawn from that population.
-
Q: Why is the Central Limit Theorem important? A: It simplifies analysis by stating that sample means tend toward a normal distribution regardless of the population distribution, provided a sufficiently large sample size.
-
Q: How does sample size affect sample distribution? A: Larger samples produce sample distributions that more closely resemble the normal distribution.
-
Q: Can sample distribution be used with non-numerical data? A: While commonly used with numerical data, sample distribution principles can be adapted to categorical data using appropriate statistical methods.
-
Q: What are the limitations of using sample distribution? A: Bias in sampling techniques can significantly affect the accuracy of inferences.
-
Q: How can I ensure I have a representative sample? A: Utilize robust sampling methods like simple random sampling, stratified sampling, or cluster sampling to minimize bias.
Summary: Understanding the nuances of sample distribution is essential for accurate statistical analysis.
Tips for Effective Sample Distribution Analysis
Introduction: These tips provide practical guidance for working with sample distributions.
Tips:
- Choose Appropriate Sample Size: Ensure a sample size large enough to satisfy the CLT's requirements and achieve desired accuracy.
- Employ Random Sampling: Utilize appropriate random sampling techniques to minimize bias.
- Visualize the Distribution: Use histograms or other graphical representations to understand the shape of the sample distribution.
- Calculate Descriptive Statistics: Compute measures like mean, standard deviation, and standard error to describe the sample distribution.
- Consider the Population Distribution: If known, the population distribution can provide valuable insights into the expected behavior of the sample distribution.
- Verify Assumptions: Before applying specific statistical tests, verify that the necessary assumptions (like normality) are met.
- Interpret Results Cautiously: Recognize the limitations of sample data and the potential for error in inferences.
Summary: Careful planning and execution are crucial for effective sample distribution analysis.
Summary: Unveiling Sample Distribution's Power
This guide explored the concept of sample distribution, highlighting its significance in statistical inference. The central limit theorem was emphasized for its crucial role in simplifying the analysis of sample distributions. Several applications across diverse fields were demonstrated, and practical tips were provided to ensure effective sample distribution analysis. Understanding sample distribution empowers researchers and analysts to make informed decisions based on data-driven insights.
Closing Message: Mastering sample distribution is a cornerstone of statistical literacy. By continuing to learn and apply these principles, individuals can enhance their analytical skills and contribute to informed decision-making across diverse fields. Further exploration into advanced statistical techniques will solidify this foundational understanding.
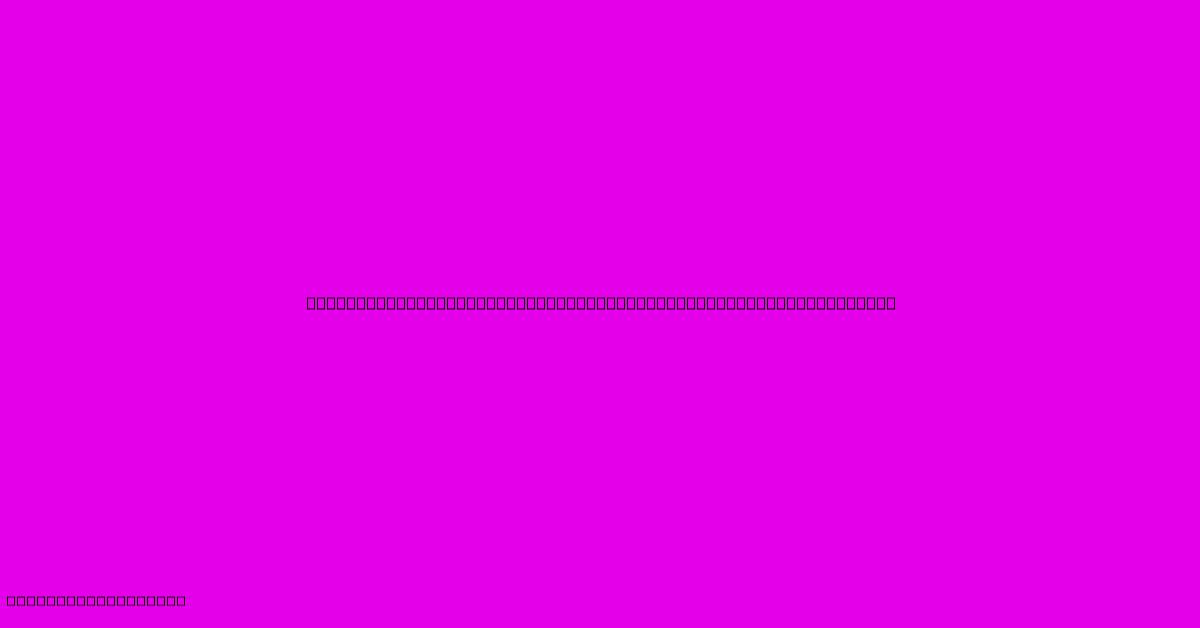
Thank you for taking the time to explore our website Sample Distribution Definition How Its Used With An Example. We hope you find the information useful. Feel free to contact us for any questions, and don’t forget to bookmark us for future visits!
We truly appreciate your visit to explore more about Sample Distribution Definition How Its Used With An Example. Let us know if you need further assistance. Be sure to bookmark this site and visit us again soon!
Featured Posts
-
Robber Barons Definition Significance Criticism And Examples
Jan 10, 2025
-
Roth Ira Conversion Definition Methods And Example
Jan 10, 2025
-
Reverse Morris Trust Rmt Definition Benefits And Tax Savings
Jan 10, 2025
-
What Rate Of Return To Use For Retirement Planning
Jan 10, 2025
-
What Does Atm Mean In Stocks
Jan 10, 2025