Skewness Positively And Negatively Skewed Defined With Formula
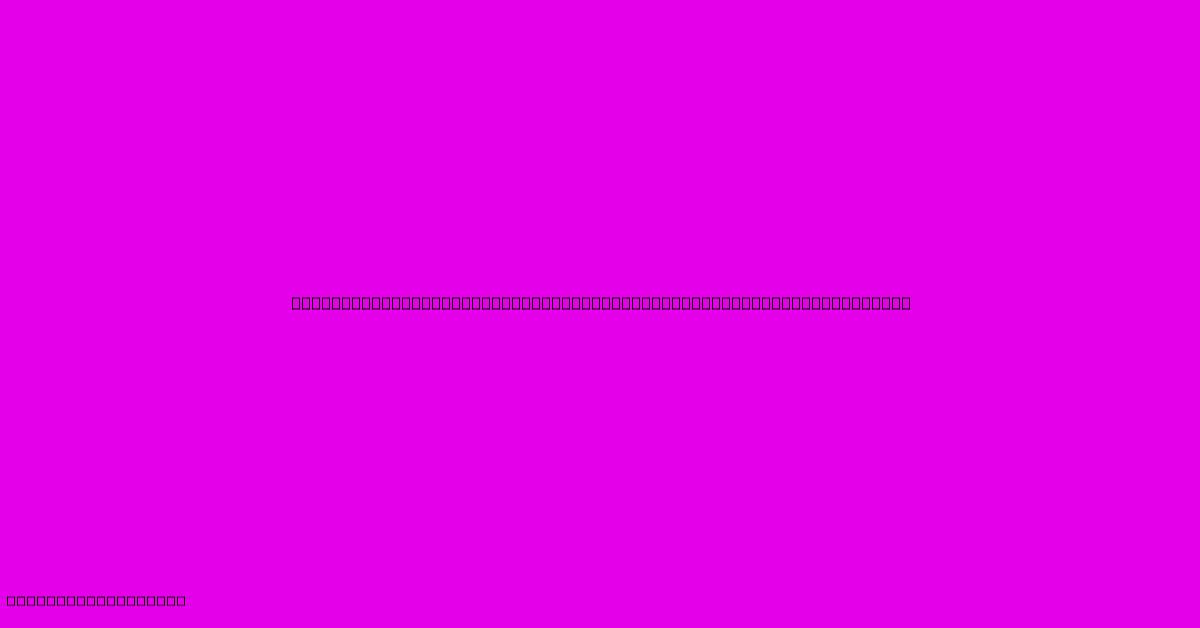
Discover more in-depth information on our site. Click the link below to dive deeper: Visit the Best Website meltwatermedia.ca. Make sure you don’t miss it!
Table of Contents
Unveiling Skewness: A Deep Dive into Positively and Negatively Skewed Distributions
Hook: Have you ever wondered why some data distributions are lopsided, with data points bunching up on one side more than the other? Understanding skewness is crucial for interpreting data accurately and making informed decisions.
Editor's Note: This comprehensive guide to positively and negatively skewed distributions has been published today. It provides a thorough explanation of skewness, including its definition, formulas, and practical applications.
Importance & Summary: Skewness, a measure of the asymmetry of a probability distribution, is a fundamental concept in statistics. Understanding skewness allows for a more nuanced interpretation of data sets, impacting areas like finance (risk assessment), healthcare (patient outcome analysis), and social sciences (income distribution). This guide will explore both positively and negatively skewed distributions, providing clear definitions, formulas, and illustrative examples. We will also delve into the practical implications of identifying and interpreting skewed data.
Analysis: This guide compiles information from various statistical resources, including textbooks, research papers, and online repositories. The analysis focuses on providing a clear and accessible explanation of skewness, incorporating illustrative examples and visuals to enhance understanding. The goal is to empower readers with the knowledge and tools to identify and interpret skewed data effectively.
Key Takeaways:
- Clear definitions of positive and negative skewness.
- Step-by-step calculation of skewness using different formulas.
- Real-world examples of positively and negatively skewed data.
- Practical applications of understanding skewness in various fields.
- Interpretation of skewness in the context of data analysis.
Subheading: Skewness: Defining Asymmetry in Data
Introduction: Skewness describes the asymmetry of a probability distribution. Unlike symmetrical distributions (like the normal distribution) where data is evenly distributed around the mean, skewed distributions show a concentration of data on one side of the mean. This imbalance reveals valuable insights into the nature of the data and can significantly impact statistical analyses.
Key Aspects:
- Measurement of Asymmetry: Skewness quantifies the degree to which a distribution deviates from symmetry.
- Impact on Mean, Median, and Mode: The relationship between the mean, median, and mode provides visual clues about skewness.
- Types of Skewness: Distributions can be positively skewed (right-skewed) or negatively skewed (left-skewed).
- Interpretation: Understanding skewness allows for more accurate interpretations of data and statistical inferences.
Discussion:
A symmetrical distribution has its mean, median, and mode all equal, located at the center. In a positively skewed distribution, the tail stretches to the right, meaning the mean is greater than the median, which is greater than the mode. Conversely, in a negatively skewed distribution, the tail extends to the left, with the mean being less than the median, which is less than the mode. Visualizing these relationships with histograms or box plots is beneficial. The degree of skewness isn't simply a matter of "slightly" or "very" skewed, it is quantified through specific formulas.
Subheading: Formulas for Calculating Skewness
Introduction: Several methods exist for calculating skewness, each with its own advantages and disadvantages. The choice of method depends on the data type and the desired level of detail.
Facets:
-
Pearson's Moment Coefficient of Skewness: This is a widely used method, particularly suitable for reasonably symmetrical distributions. The formula is:
Skewness = 3 * (Mean - Median) / Standard Deviation
This formula leverages the relationship between the mean, median, and standard deviation to quantify skewness. A value of 0 indicates symmetry, positive values indicate positive skewness, and negative values indicate negative skewness. The magnitude of the value reflects the degree of skewness.
-
Fisher-Pearson Standardized Moment Coefficient: This method is often preferred because it standardizes the skewness measure, providing a more consistent interpretation across different datasets. The formula is a bit more complex, involving higher-order moments:
Skewness = [n/(n-1)(n-2)] * Σ(xi - x̄)³ / s³
Where:
- n = the number of data points
- xi = the individual data points
- x̄ = the sample mean
- s = the sample standard deviation
-
Software Calculations: Statistical software packages (like R, SPSS, Python with libraries such as SciPy) readily calculate skewness, often providing both Pearson's and Fisher-Pearson's coefficients.
Summary: While different formulas exist, they all aim to capture the asymmetry of a distribution. Choosing the appropriate formula depends on the context and the specific requirements of the analysis. Interpreting the results in relation to the shape of the distribution (as visualized in a histogram or box plot) is essential for a complete understanding.
Subheading: Real-World Examples of Skewed Distributions
Introduction: Understanding skewness is not just a theoretical exercise; it has profound implications for interpreting real-world data.
Further Analysis:
-
Positive Skewness: Income distribution often shows positive skewness. A few high earners significantly increase the mean, while most individuals earn less, creating a long right tail. Similarly, home prices in a given area might be positively skewed due to the presence of a few very expensive properties.
-
Negative Skewness: Test scores on an easy exam could exhibit negative skewness. Most students score high, creating a concentration on the right side, with a few low scores creating a tail extending to the left. Another example is the age at which people first learn to ride a bike; a majority of children learn at a young age, creating a leftward skew.
Closing: Observing skewness in real-world data highlights the importance of considering not just the mean but also the median and mode, as well as the shape of the distribution, for a complete picture. Ignoring skewness can lead to flawed conclusions and inaccurate predictions.
Subheading: FAQ
Introduction: This section addresses frequently asked questions regarding skewness and its interpretation.
Questions:
-
Q: What is the difference between positive and negative skewness? A: Positive skewness implies a longer tail to the right, while negative skewness implies a longer tail to the left of the distribution.
-
Q: How does skewness affect the mean, median, and mode? A: In positive skewness, Mean > Median > Mode. In negative skewness, Mean < Median < Mode.
-
Q: Can a distribution have zero skewness? A: Yes, a perfectly symmetrical distribution has a skewness of zero.
-
Q: What is the significance of the magnitude of skewness? A: The magnitude indicates the degree of asymmetry; a larger absolute value means a more pronounced skew.
-
Q: How does skewness affect statistical inference? A: Skewness can impact the validity of certain statistical tests that assume normality.
-
Q: How can I visualize skewness? A: Histograms and box plots provide visual representations of skewness.
Summary: Understanding these FAQs is crucial for correctly interpreting and utilizing skewness as a statistical tool.
Transition: Next, let's explore practical tips for handling skewed data.
Subheading: Tips for Handling Skewed Data
Introduction: Dealing with skewed data often requires specific approaches to ensure accurate analysis and interpretation.
Tips:
- Data Transformation: Techniques like logarithmic or square root transformations can reduce skewness.
- Non-parametric Tests: Consider non-parametric statistical tests which are less sensitive to deviations from normality.
- Robust Statistical Measures: Utilize robust statistics, such as the median, which are less influenced by outliers.
- Visual Inspection: Always inspect your data visually using histograms and box plots to assess skewness.
- Consider the Context: The interpretation of skewness should always consider the context of the data.
- Report Skewness: Clearly document the skewness of your data in any analysis.
- Use Appropriate Visualization: Choose visualizations that best represent skewed data, avoiding misleading presentations.
Summary: These tips help ensure that skewed data is handled appropriately and interpreted correctly, avoiding misleading conclusions.
Transition: Now let's summarize our exploration of skewness.
Summary: This guide has provided a comprehensive overview of skewness, covering its definition, calculation methods, interpretation, and practical applications. We have explored both positively and negatively skewed distributions, illustrating their characteristics and implications.
Closing Message: Understanding skewness is a fundamental step towards accurate and insightful data analysis. By mastering the concepts presented in this guide, data analysts can interpret data with greater precision and avoid misinterpretations that can arise from ignoring the asymmetry in their datasets. The ability to recognize and quantify skewness is a valuable skill for any data professional.
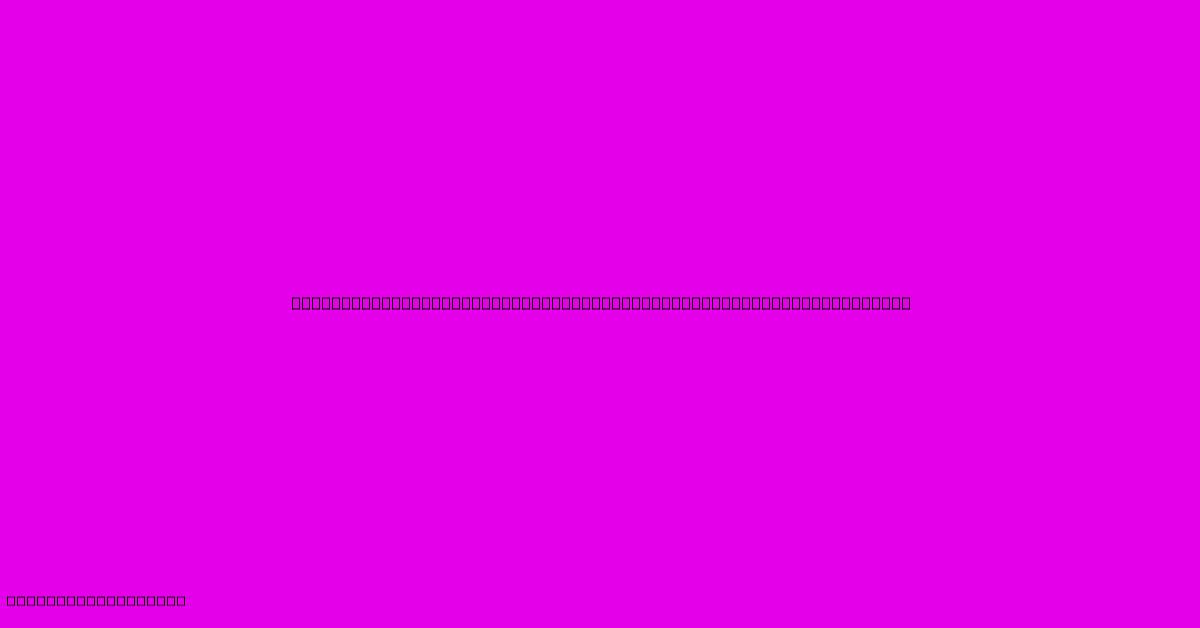
Thank you for taking the time to explore our website Skewness Positively And Negatively Skewed Defined With Formula. We hope you find the information useful. Feel free to contact us for any questions, and don’t forget to bookmark us for future visits!
We truly appreciate your visit to explore more about Skewness Positively And Negatively Skewed Defined With Formula. Let us know if you need further assistance. Be sure to bookmark this site and visit us again soon!
Featured Posts
-
Back End Ratio Definition Calculation Formula Vs Front End
Jan 11, 2025
-
Spot Next Definition
Jan 11, 2025
-
Shareholder Value Definition Calculation And How To Maximize It
Jan 11, 2025
-
Step Up In Basis Definition How It Works For Inherited Property
Jan 11, 2025
-
Accrued Interest Definition Example
Jan 11, 2025