Social Sentiment Indicator Definition
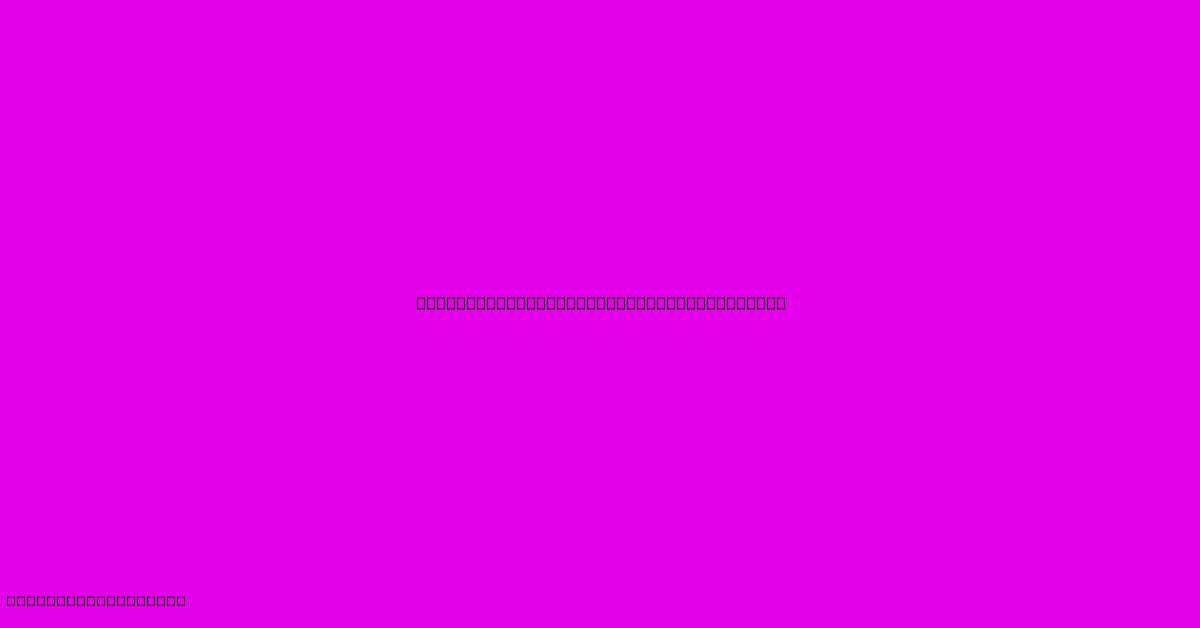
Discover more in-depth information on our site. Click the link below to dive deeper: Visit the Best Website meltwatermedia.ca. Make sure you don’t miss it!
Table of Contents
Unlocking the Power of Social Sentiment Indicators: A Comprehensive Guide
Editor's Note: This comprehensive guide to social sentiment indicators has been published today, providing invaluable insights into this crucial aspect of data analysis.
Importance & Summary: Understanding social sentiment is vital for businesses, researchers, and policymakers alike. This guide explores the definition, measurement, and application of social sentiment indicators, highlighting their role in gauging public opinion, predicting market trends, and informing strategic decision-making. It delves into various methodologies, challenges, and future directions in the field of social sentiment analysis.
Analysis: This guide compiles information from academic research papers, industry reports, and practical applications of social sentiment indicators. Data from various sources, including social media platforms, news articles, and online forums, were analyzed to provide a holistic understanding of the subject. A focus on clear explanations and illustrative examples ensures practical applicability for a diverse readership.
Key Takeaways:
- Social sentiment indicators reflect public opinion on a particular topic.
- Accurate measurement requires robust methodologies and data analysis techniques.
- Applications span various fields, including marketing, finance, and political science.
- Challenges include data biases and the complexities of natural language processing.
- Future developments focus on improved accuracy and real-time analysis.
Social Sentiment Indicators: A Deep Dive
Introduction: Social sentiment indicators represent a powerful tool for understanding and interpreting public opinion. These indicators quantify the prevailing sentiment—positive, negative, or neutral—expressed towards a specific entity, event, or topic across various digital platforms. Their accurate measurement and interpretation are critical for informed decision-making across diverse sectors.
Key Aspects:
- Data Sources: Social media platforms (Twitter, Facebook, Instagram), news articles, blogs, online forums, and review websites provide rich sources of data for analyzing social sentiment.
- Sentiment Classification: Determining whether a piece of text expresses positive, negative, or neutral sentiment forms the core of social sentiment analysis. This involves natural language processing (NLP) techniques.
- Indicator Development: Aggregating individual sentiment scores across numerous data points produces a comprehensive social sentiment indicator, offering a macro-level perspective on public opinion.
- Application and Interpretation: The derived indicators are utilized across sectors for market research, risk management, crisis communication, and strategic planning. Careful interpretation considering contextual factors is crucial.
Data Sources and Collection Methods
Introduction: The foundation of effective social sentiment analysis rests on the selection and aggregation of data from diverse online sources. The choice of data sources directly impacts the accuracy and scope of the generated indicators.
Facets:
- Social Media Platforms: These offer vast quantities of unstructured data expressing real-time opinions. However, challenges include data biases, noise, and the need for sophisticated NLP techniques.
- News Articles and Blogs: These sources offer more structured and curated content, potentially leading to higher reliability but reduced volume compared to social media.
- Online Forums and Review Websites: These provide focused feedback on specific products, services, or issues, allowing for targeted sentiment analysis.
- Data Collection Techniques: Methods range from simple keyword searches to sophisticated web scraping techniques, API access, and specialized data providers. Ethical considerations and compliance with platform policies are paramount.
Summary: The selection of data sources must align with the research objectives. A multi-source approach, combining data from various platforms, typically produces a more robust and representative social sentiment indicator.
Sentiment Classification Techniques
Introduction: Converting textual data into quantifiable sentiment scores requires sophisticated techniques. Various approaches exist, each with its strengths and limitations.
Facets:
- Lexicon-Based Approach: This relies on pre-defined dictionaries containing words and their associated sentiment scores. While straightforward to implement, it may struggle with nuanced language and sarcasm.
- Machine Learning Approach: This utilizes algorithms trained on large datasets of labelled text to classify sentiment. This offers higher accuracy but requires significant computational resources and data preprocessing.
- Hybrid Approaches: Combining lexicon-based and machine learning techniques often yields improved accuracy by leveraging the strengths of each.
- Challenges: Handling negation, sarcasm, irony, and the evolving nature of language pose significant challenges to accurate sentiment classification.
Summary: The choice of sentiment classification technique hinges on the available resources, data characteristics, and desired accuracy level. Ongoing research constantly refines these techniques, aiming for improved robustness and precision.
Indicator Development and Aggregation
Introduction: Raw sentiment scores obtained from individual data points need to be aggregated to create meaningful social sentiment indicators. This process requires careful consideration of data normalization, weighting, and temporal dynamics.
Further Analysis: Different aggregation strategies, such as simple averaging, weighted averaging based on source reliability or user influence, and time-series analysis to account for trends, can be employed. Normalization techniques ensure comparable sentiment scores across different data sources.
Closing: The development of reliable social sentiment indicators necessitates a systematic and rigorous approach, acknowledging inherent limitations and potential biases within the data.
Applications and Interpretations of Social Sentiment Indicators
Introduction: Social sentiment indicators find widespread applications across numerous domains, offering valuable insights for strategic decision-making. However, proper interpretation within context is crucial to avoid misinterpretations.
Further Analysis:
- Marketing and Brand Management: Tracking consumer sentiment towards brands and products enables targeted marketing campaigns and improved product development.
- Financial Markets: Analyzing public sentiment towards specific companies or industries can help predict stock price movements and assess investment risk.
- Political Science and Public Policy: Monitoring public opinion on political issues and candidates informs policy decisions and election forecasting.
- Crisis Communication and Risk Management: Early detection of negative sentiment can help organizations mitigate reputational damage and effectively respond to crises.
Closing: Social sentiment indicators provide a powerful tool for understanding the pulse of public opinion. However, their successful application hinges on a thorough understanding of their limitations and careful consideration of contextual factors.
FAQ
Introduction: This section addresses common queries regarding social sentiment indicators.
Questions:
- Q: What are the limitations of social sentiment indicators? A: They can be susceptible to biases in data sources, limitations in natural language processing techniques, and difficulties in interpreting nuanced language.
- Q: How can accuracy be improved? A: Utilizing hybrid sentiment classification methods, incorporating contextual information, and employing rigorous data cleaning and preprocessing techniques can enhance accuracy.
- Q: What ethical considerations are involved? A: Privacy concerns, potential for manipulation, and responsible data usage are critical ethical considerations.
- Q: Are social sentiment indicators always reliable? A: No, they should be interpreted carefully considering limitations and potential biases. They are valuable tools, not infallible predictors.
- Q: How can businesses use these indicators? A: For market research, brand monitoring, crisis management, and improving customer service.
- Q: What is the future of social sentiment analysis? A: Advancements in AI and NLP will likely improve accuracy and real-time analysis capabilities.
Summary: Understanding the strengths and limitations of social sentiment indicators is key to their effective application.
Transition: The following section provides practical tips for leveraging the power of social sentiment indicators.
Tips for Effective Social Sentiment Analysis
Introduction: This section offers practical guidance for maximizing the value derived from social sentiment indicators.
Tips:
- Define clear objectives: Clearly articulate the research question or business problem before starting analysis.
- Select appropriate data sources: Choose sources that align with the research objectives and consider multiple sources for robustness.
- Employ robust sentiment classification techniques: Choose techniques appropriate to the data and resources available.
- Consider contextual factors: Interpret results within the broader context, accounting for potential biases and evolving trends.
- Visualize and communicate findings: Present data clearly and concisely using appropriate visualizations.
- Monitor and adapt: Regularly monitor social sentiment and adjust strategies accordingly.
- Ensure ethical compliance: Adhere to data privacy regulations and responsible data usage practices.
- Utilize advanced analytics: Employ time-series analysis and other advanced techniques for deeper insights.
Summary: Following these guidelines will ensure a more effective and insightful analysis of social sentiment.
Transition: The following section summarizes the key insights explored in this guide.
Summary of Social Sentiment Indicators
Summary: This guide provided a comprehensive exploration of social sentiment indicators, encompassing their definition, measurement, and applications across various fields. The importance of accurate data collection, robust analysis techniques, and ethical considerations was highlighted. Challenges and future directions in the field were also addressed.
Closing Message: Social sentiment indicators offer a powerful lens through which to understand the dynamics of public opinion. By embracing best practices and continually adapting to technological advancements, researchers and organizations can leverage this crucial information to improve decision-making, anticipate future trends, and create more effective strategies.
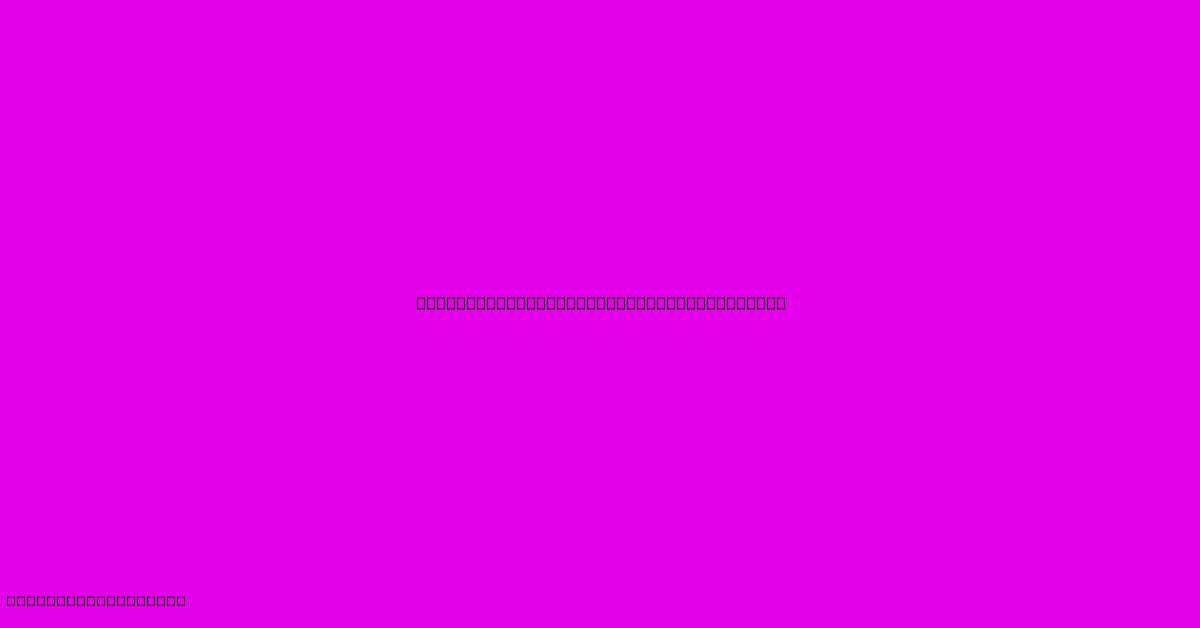
Thank you for taking the time to explore our website Social Sentiment Indicator Definition. We hope you find the information useful. Feel free to contact us for any questions, and don’t forget to bookmark us for future visits!
We truly appreciate your visit to explore more about Social Sentiment Indicator Definition. Let us know if you need further assistance. Be sure to bookmark this site and visit us again soon!
Featured Posts
-
How Do I Use My Jetblue Travel Credit
Jan 11, 2025
-
What Does Odp Mean In Banking
Jan 11, 2025
-
How To Set Up Zelle With Suncoast Credit Union
Jan 11, 2025
-
Vancouver Stock Exchange Van Definition
Jan 11, 2025
-
Singapore Dollar Sgd Definition Currency Symbol History
Jan 11, 2025