Standard Error Se Definition Standard Deviation In Statistics Explained
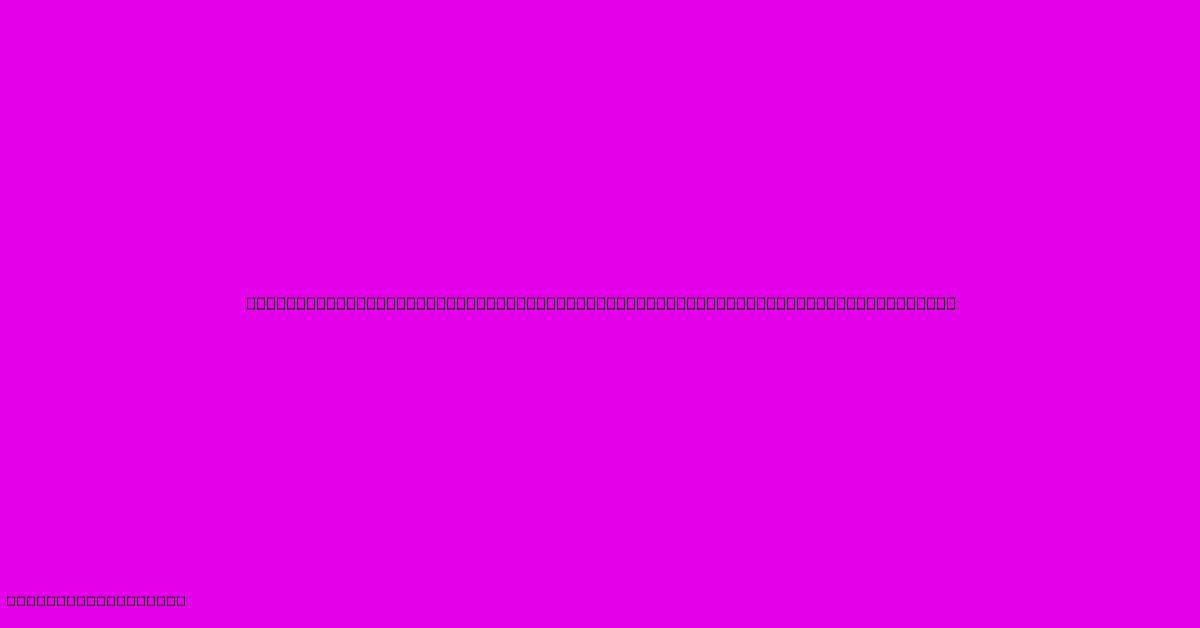
Discover more in-depth information on our site. Click the link below to dive deeper: Visit the Best Website meltwatermedia.ca. Make sure you don’t miss it!
Table of Contents
Unveiling the Mysteries of Standard Error: A Deep Dive into Statistical Significance
Does the standard error (SE) leave you scratching your head? Understanding standard error is crucial for interpreting statistical analyses and drawing meaningful conclusions from data. This comprehensive guide will demystify the concept of standard error, its relationship to standard deviation, and its vital role in statistical inference.
Editor's Note: This in-depth analysis of standard error and its connection to standard deviation in statistics has been published today. It aims to provide a clear and accessible explanation for both students and professionals.
Importance & Summary: The standard error is a fundamental concept in statistics, allowing researchers to quantify the uncertainty associated with sample estimates. This guide will explore the standard error definition, its calculation, its relationship with standard deviation, and its applications in hypothesis testing and confidence intervals. We'll utilize semantic keywords and LSI (Latent Semantic Indexing) terms such as sampling distribution, confidence level, margin of error, statistical significance, and inferential statistics to provide a holistic understanding of this crucial statistical measure.
Analysis: This guide synthesizes information from various reputable statistical textbooks and research papers. The explanations are grounded in established statistical principles, with illustrative examples to ensure clarity and practical application. A focus is placed on providing actionable insights, enabling readers to apply the concepts to their own analyses.
Key Takeaways:
- Standard error measures the variability of a sample statistic (e.g., mean).
- It quantifies the uncertainty associated with estimating a population parameter.
- Standard error is closely related to the standard deviation but focuses on sample estimates.
- Smaller standard error indicates higher precision in estimation.
- Standard error plays a critical role in hypothesis testing and confidence interval construction.
Standard Error (SE): A Deeper Look
Introduction
The standard error is a measure of the statistical accuracy of an estimate, especially of the mean. It quantifies the variability we'd expect to see if we were to repeatedly sample from the same population and calculate the mean for each sample. Understanding its key aspects is paramount for making reliable inferences about populations based on sample data.
Key Aspects of Standard Error
- Sample Statistic: Standard error applies to sample statistics, not individual data points. The most common application is with the sample mean.
- Population Parameter: The ultimate goal is usually to estimate a population parameter (e.g., the population mean) using the sample statistic.
- Sampling Distribution: The standard error is intimately tied to the concept of the sampling distribution. This is the theoretical distribution of all possible sample statistics (like the mean) that could be obtained from repeatedly sampling from the same population.
- Precision of Estimation: A smaller standard error implies a more precise estimate of the population parameter.
Discussion
Imagine repeatedly taking random samples from a population and calculating the mean of each sample. These sample means will vary somewhat, creating a distribution of sample means. The standard error of the mean (SEM) is the standard deviation of this distribution of sample means. The formula for the SEM is:
SEM = σ / √n
where:
- σ (sigma) represents the population standard deviation.
- n represents the sample size.
Note that in practice, the population standard deviation (σ) is usually unknown. Therefore, it is typically estimated using the sample standard deviation (s):
SEM ≈ s / √n
This approximation becomes more accurate as the sample size increases. The key takeaway here is that the standard error decreases as the sample size (n) increases. Larger samples provide more precise estimates of the population parameter. This connection between sample size and standard error is fundamental in statistical power analysis.
Standard Deviation vs. Standard Error
While both standard deviation and standard error involve the square root of variance, they differ fundamentally:
- Standard Deviation (SD): Measures the spread or dispersion of individual data points within a single sample. It describes the variability within the data itself.
- Standard Error (SE): Measures the variability of a sample statistic (e.g., the mean) across multiple samples. It describes the variability of the estimate.
It's crucial to understand this distinction to avoid misinterpretations. The standard deviation helps describe the data itself, while the standard error helps assess the accuracy of our estimate of a population parameter based on that data.
The Role of Standard Error in Hypothesis Testing and Confidence Intervals
Hypothesis Testing
In hypothesis testing, the standard error is essential for calculating the test statistic. For example, in a t-test comparing two group means, the test statistic is calculated using the standard errors of the means of the two groups. The smaller the standard errors, the more likely it is that a statistically significant difference between the group means will be detected.
Confidence Intervals
Standard error is also vital in constructing confidence intervals. A confidence interval provides a range of values within which the true population parameter is likely to fall. The formula for a confidence interval for a mean is:
Confidence Interval = Sample Mean ± (Critical Value) * (Standard Error)
The critical value depends on the chosen confidence level (e.g., 95%) and the degrees of freedom. The standard error determines the width of the confidence interval. A smaller standard error results in a narrower confidence interval, indicating a more precise estimate.
Practical Applications and Considerations
The standard error finds widespread applications across various disciplines including:
- Medicine: Evaluating the effectiveness of treatments.
- Engineering: Assessing the reliability of measurements.
- Social Sciences: Analyzing survey data and experimental results.
- Finance: Estimating investment returns and risks.
It is important to remember that the standard error is an estimate itself and is subject to uncertainty. Its accuracy improves with larger sample sizes. Additionally, the standard error assumes that the data meet certain assumptions (e.g., normality, independence). Violations of these assumptions can affect the accuracy of the standard error.
FAQ: Standard Error and Standard Deviation
Introduction
This section addresses frequently asked questions about standard error and standard deviation to clarify common misconceptions and deepen understanding.
Questions and Answers
Q1: What is the difference between standard deviation and standard error?
A1: Standard deviation describes the variability within a single dataset, while standard error describes the variability of a sample statistic (like the mean) across multiple samples. Standard error reflects how accurately the sample statistic estimates the population parameter.
Q2: Can I use the standard error to make inferences about individual data points?
A2: No. Standard error applies to sample statistics, not individual data points. Standard deviation is the appropriate measure for the variability of individual data points.
Q3: How does sample size affect the standard error?
A3: Larger sample sizes lead to smaller standard errors, resulting in more precise estimates of population parameters.
Q4: What is the relationship between standard error and confidence intervals?
A4: The standard error is a key component in calculating confidence intervals. A smaller standard error leads to a narrower confidence interval, indicating higher precision in estimating the population parameter.
Q5: Is it possible to have a standard error of zero?
A5: Theoretically, yes, if all sample means are identical, implying no variability in the sampling distribution. This is highly unlikely in real-world applications.
Q6: How does standard error relate to statistical significance?
A6: A smaller standard error increases the likelihood of finding statistically significant results in hypothesis testing as it reduces the uncertainty in the sample estimates.
Summary
Understanding the distinction between standard deviation and standard error, and their interplay with sample size and confidence intervals, is essential for accurate statistical inference.
Tips for Working with Standard Error
Introduction
This section provides practical tips for effectively using and interpreting standard error in statistical analyses.
Tips
- Always clearly define the population parameter being estimated. Understanding the context of the analysis is critical for proper interpretation.
- Verify that the underlying assumptions are met. The accuracy of the standard error relies on the validity of assumptions like normality and independence.
- Consider the sample size. Larger samples generally lead to smaller standard errors and more precise estimates.
- Use appropriate statistical software. Statistical software packages provide tools for easily calculating standard errors and constructing confidence intervals.
- Properly interpret the results. Avoid over-interpreting small differences or ignoring the uncertainty inherent in estimations.
- Consider reporting both the point estimate and its standard error. This provides a complete picture of the results, showing both the estimate and its associated uncertainty.
- Consult with a statistician when necessary. For complex analyses or when dealing with unusual datasets, seeking expert guidance is advisable.
Summary
By following these tips, researchers and analysts can enhance the reliability and accuracy of their statistical analyses, particularly when using standard error.
Summary of Standard Error and Standard Deviation
This guide provided a comprehensive overview of standard error, its relationship with standard deviation, and its applications in statistical inference. Understanding these concepts is crucial for interpreting statistical analyses correctly and drawing meaningful conclusions from data. Standard error provides a critical measure of the uncertainty associated with sample estimates, allowing researchers to quantify the precision of their findings.
Closing Message
Mastering the concept of standard error is a cornerstone of sound statistical practice. By understanding its nuances and applications, researchers and analysts can make more informed decisions, and contribute to robust and reliable scientific advancements. Continued learning and critical analysis of statistical methods are essential for success in this field.
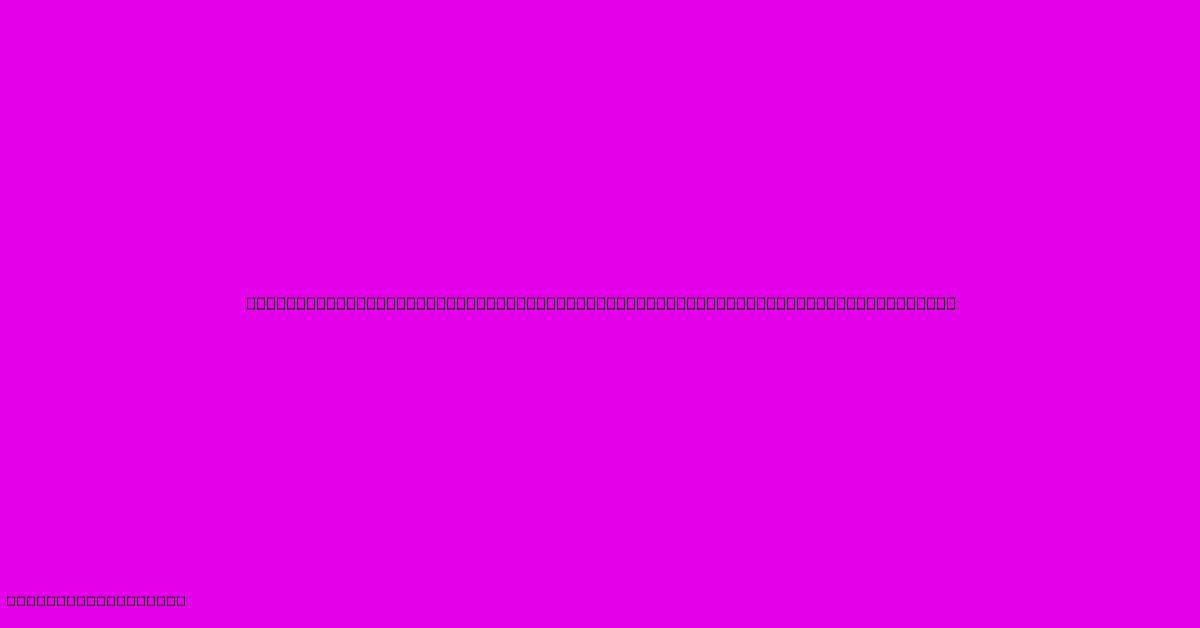
Thank you for taking the time to explore our website Standard Error Se Definition Standard Deviation In Statistics Explained. We hope you find the information useful. Feel free to contact us for any questions, and don’t forget to bookmark us for future visits!
We truly appreciate your visit to explore more about Standard Error Se Definition Standard Deviation In Statistics Explained. Let us know if you need further assistance. Be sure to bookmark this site and visit us again soon!
Featured Posts
-
12b 1 Fund Definition
Jan 11, 2025
-
Average Annual Return Aar Definition Calculation And Example
Jan 11, 2025
-
Swap Spread Definition Use As An Indicator And Example
Jan 11, 2025
-
What Are Soft Skills Definition Importance And Examples
Jan 11, 2025
-
Shadow Pricing Definition How It Works Uses And Example
Jan 11, 2025