What Is A Two Tailed Test Definition And Example
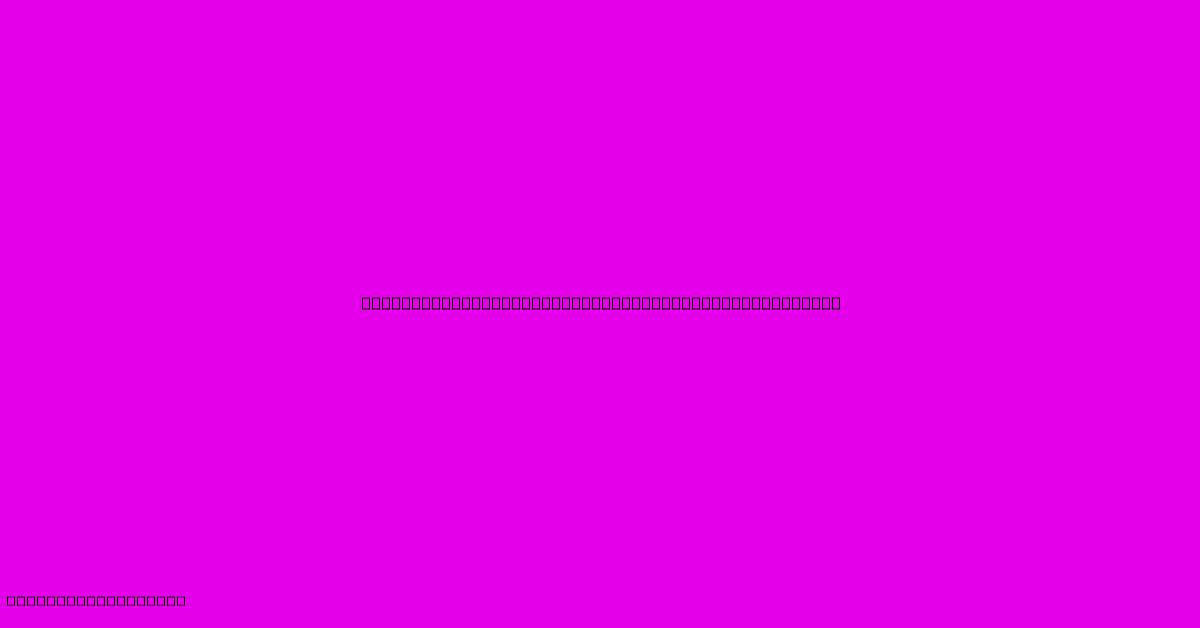
Discover more in-depth information on our site. Click the link below to dive deeper: Visit the Best Website meltwatermedia.ca. Make sure you don’t miss it!
Table of Contents
Unveiling the Two-Tailed Test: A Deep Dive with Examples
What's the difference between a situation where you suspect an effect might increase or decrease, versus one where you only suspect an increase? This subtle distinction leads us to the powerful statistical tool known as the two-tailed test. This article will explore its definition, applications, and provide illustrative examples.
Editor's Note: This comprehensive guide to two-tailed tests has been published today, offering valuable insights for researchers and data analysts.
Importance & Summary
Understanding hypothesis testing is crucial for drawing reliable conclusions from data. The two-tailed test plays a vital role by allowing researchers to investigate whether a parameter differs significantly from a hypothesized value in either direction (positive or negative). This contrasts with a one-tailed test, which only examines deviation in a single direction. This guide will summarize the key concepts, calculations, and interpretations of two-tailed tests, using illustrative examples to solidify understanding.
Analysis
This guide utilizes a combination of theoretical explanations, practical examples, and statistical software demonstrations (though code won't be directly presented here) to explain the two-tailed test. The information is drawn from reputable statistical textbooks, research articles, and online resources. The examples are designed to represent real-world scenarios where the two-tailed test is commonly applied.
Key Takeaways
- A two-tailed test examines whether a sample mean differs significantly from a population mean in either direction (greater than or less than).
- It uses a two-tailed p-value to assess the significance of the results.
- The critical region is split into two tails of the distribution.
- It's appropriate when the direction of the difference is unknown.
- The two-tailed test is more conservative than a one-tailed test.
What is a Two-Tailed Test?
A two-tailed test, also known as a two-sided test, is a statistical test used to determine if there is a significant difference between two groups or if a sample mean differs significantly from a population mean, without specifying the direction of the difference. This is a crucial distinction: the researcher doesn't have a pre-conceived notion of whether the difference will be positive or negative.
Key Aspects of Two-Tailed Tests
- Null Hypothesis (H₀): This hypothesis typically states that there is no significant difference between the groups or between the sample mean and the population mean (e.g., H₀: μ₁ = μ₂ or H₀: μ = μ₀).
- Alternative Hypothesis (H₁): This hypothesis states that there is a significant difference, but doesn't specify the direction (e.g., H₁: μ₁ ≠ μ₂ or H₁: μ ≠ μ₀).
- Significance Level (α): This is the probability of rejecting the null hypothesis when it is actually true (Type I error). It is commonly set at 0.05 (5%).
- Critical Region: This is the area in the tails of the sampling distribution where the test statistic falls, leading to the rejection of the null hypothesis. In a two-tailed test, the critical region is split equally between the two tails.
- P-value: The probability of obtaining results as extreme as, or more extreme than, the observed results, assuming the null hypothesis is true. A small p-value (typically less than α) leads to the rejection of the null hypothesis.
Example: Comparing Mean Test Scores
Let's say a school implemented a new teaching method and wants to assess its impact on student test scores. They randomly select 30 students and administer a standardized test. The average score for this group is 78, while the population average (before the new method) is 75, with a known population standard deviation of 10. There is no prior reason to believe the new method would either improve or worsen scores. Therefore, a two-tailed test is appropriate.
H₀: μ = 75 (The new method has no effect on average scores) H₁: μ ≠ 75 (The new method has a significant effect on average scores, either increasing or decreasing them)
Using a t-test (since the population standard deviation is known), we would calculate the t-statistic and compare it to the critical t-value at the chosen significance level (e.g., 0.05) with the appropriate degrees of freedom. If the absolute value of the calculated t-statistic exceeds the critical t-value, we reject the null hypothesis. The p-value would also be calculated; if it's less than 0.05, we reject the null hypothesis.
Example: Comparing Sales Figures Between Two Marketing Campaigns
A company runs two different marketing campaigns (A and B) and wants to compare their effectiveness in terms of sales revenue. They collect sales data for both campaigns over a month. The average revenue for Campaign A is $10,000 and for Campaign B is $12,000. They don't have a pre-existing belief about which campaign will perform better. Again, a two-tailed test is suitable.
H₀: μA = μB (Both campaigns have equal average revenue) H₁: μA ≠ μB (The campaigns have significantly different average revenue)
Here, an independent samples t-test or a z-test (depending on sample size and whether population standard deviations are known) would be used to compare the means. A significant p-value (less than α) would indicate a significant difference in sales revenue between the two campaigns, without specifying which campaign performed better.
Two-Tailed Test vs. One-Tailed Test
The key difference lies in the alternative hypothesis and the resulting critical region. A one-tailed test specifies the direction of the difference (e.g., H₁: μ₁ > μ₂), while a two-tailed test doesn't (e.g., H₁: μ₁ ≠ μ₂). Consequently, the critical region in a one-tailed test is located in only one tail of the distribution, making it more likely to reject the null hypothesis (it's more powerful). However, a two-tailed test is more conservative because it accounts for deviations in both directions.
Choosing the Right Test
The choice between a one-tailed and a two-tailed test depends entirely on the research question and the prior knowledge available. If there's a strong theoretical reason or prior evidence to suggest the direction of the effect, a one-tailed test might be appropriate. However, in the absence of such prior knowledge, the two-tailed test is generally preferred due to its greater conservatism.
FAQ
Introduction: This section answers frequently asked questions about two-tailed tests.
Questions:
-
Q: What is the difference between a p-value and a significance level (α)? A: The significance level (α) is a pre-determined threshold for rejecting the null hypothesis. The p-value is the probability of observing the obtained results (or more extreme results) if the null hypothesis were true. If the p-value is less than α, we reject the null hypothesis.
-
Q: When should I use a two-tailed test over a one-tailed test? A: Use a two-tailed test when you don't have a prior expectation about the direction of the effect. If you have a strong reason to believe the effect will be in a specific direction, then a one-tailed test is appropriate.
-
Q: What happens if my p-value is greater than my significance level? A: If your p-value is greater than your significance level (α), you fail to reject the null hypothesis. This means that there is insufficient evidence to support the alternative hypothesis.
-
Q: Can I change my test from two-tailed to one-tailed after seeing the results? A: No, this is considered inappropriate statistical practice. The type of test should be decided before the data analysis begins.
-
Q: What are the assumptions of a two-tailed t-test? A: The assumptions include independence of observations, approximately normal distribution of the data, and homogeneity of variances (for independent samples t-tests).
-
Q: What software can I use to perform two-tailed tests? A: Many statistical software packages such as SPSS, R, SAS, and Python (with libraries like SciPy and Statsmodels) can be used.
Summary: This FAQ section clarified common misconceptions and provided practical guidance on applying two-tailed tests.
Tips for Using Two-Tailed Tests
Introduction: This section provides practical tips for successful implementation of two-tailed tests.
Tips:
- Clearly define your hypotheses: Before conducting any analysis, ensure you clearly articulate both your null and alternative hypotheses.
- Choose the appropriate statistical test: Select the test that aligns with your data type and research question (t-test, z-test, etc.).
- Check assumptions: Verify that your data meets the assumptions of the chosen statistical test.
- Interpret the results carefully: Don't just focus on whether the p-value is less than α. Consider the effect size and the practical significance of the findings.
- Report your results transparently: Clearly communicate your methodology, results, and conclusions in your reports.
- Consider power analysis: Before your study, perform a power analysis to determine the sample size needed to detect a meaningful effect with reasonable certainty.
Summary: Careful planning and execution are crucial for accurate interpretation of results from two-tailed tests.
Summary of Two-Tailed Tests
This article provided a detailed explanation of the two-tailed test, highlighting its importance in hypothesis testing. The key difference from a one-tailed test lies in its consideration of deviations in both directions from the null hypothesis. Examples illustrated its applications in comparing means and analyzing the impact of interventions. Understanding the assumptions, choosing the right test, and correctly interpreting the results are crucial for drawing valid conclusions.
Closing Message: The two-tailed test serves as a fundamental tool in statistical inference, empowering researchers to analyze data objectively and arrive at well-supported conclusions. Mastering its application enhances the rigor and reliability of research across various disciplines. Future research should continue to explore its applications and refine its methodological considerations to further improve its usefulness.
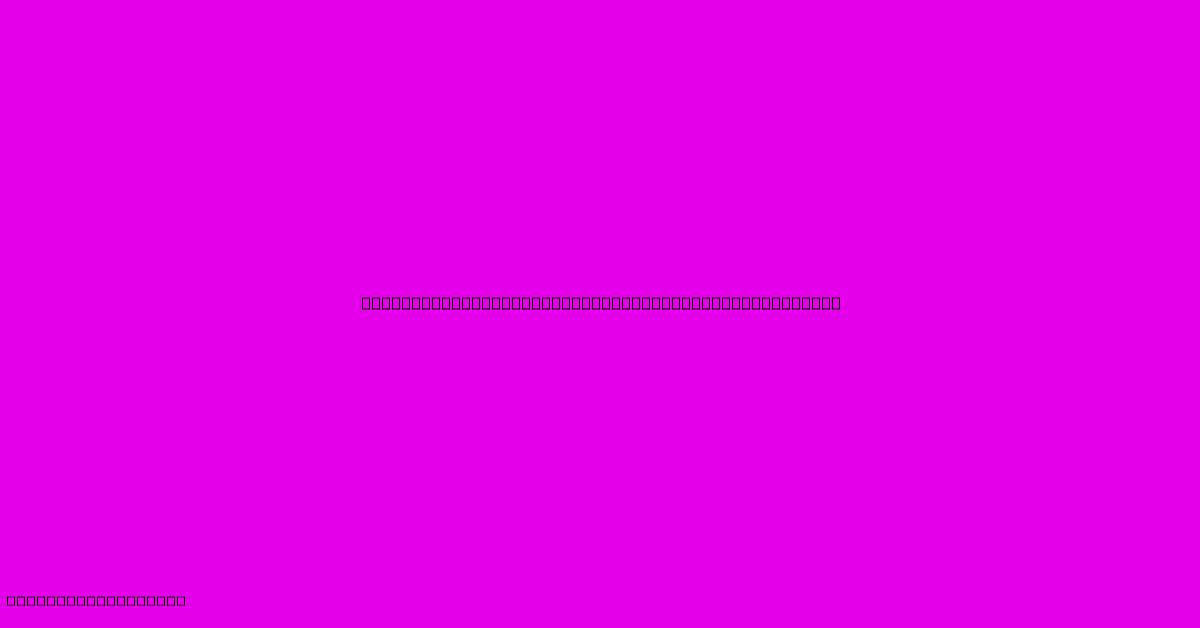
Thank you for taking the time to explore our website What Is A Two Tailed Test Definition And Example. We hope you find the information useful. Feel free to contact us for any questions, and don’t forget to bookmark us for future visits!
We truly appreciate your visit to explore more about What Is A Two Tailed Test Definition And Example. Let us know if you need further assistance. Be sure to bookmark this site and visit us again soon!
Featured Posts
-
Setup Price Definition
Jan 11, 2025
-
Automatic Reinvestment Plan Arp Definition
Jan 11, 2025
-
Toll Revenue Bond Definition Components And Examples
Jan 11, 2025
-
Tri Party Agreement Definition
Jan 11, 2025
-
Special Employer Definition
Jan 11, 2025