What Is Credit Ai
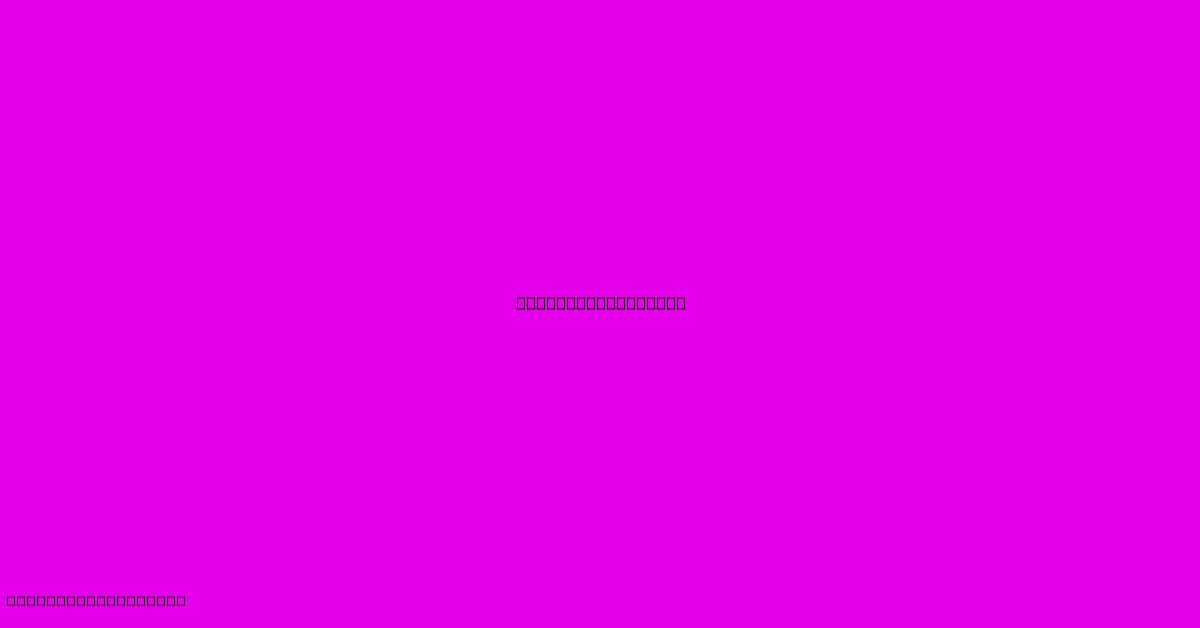
Discover more in-depth information on our site. Click the link below to dive deeper: Visit the Best Website meltwatermedia.ca. Make sure you don’t miss it!
Table of Contents
Unveiling the Power of Credit AI: Revolutionizing Financial Access and Risk Assessment
Hook: Is your business struggling to accurately assess credit risk? Credit AI is transforming how lenders evaluate borrowers, opening doors to previously underserved markets and streamlining the lending process.
Editor's Note: This comprehensive guide to Credit AI has been published today. It explores the technology's capabilities, applications, benefits, and challenges.
Importance & Summary: Credit AI represents a significant advancement in financial technology (FinTech). By leveraging machine learning and artificial intelligence, it enhances credit scoring models, improves fraud detection, and automates lending processes. This summary will explore the technology's core components, its impact on lending practices, and its implications for both lenders and borrowers. The analysis will cover various AI techniques employed, the data used, ethical considerations, and the future potential of Credit AI.
Analysis: The information compiled in this guide draws upon research from leading financial technology publications, academic studies on AI in finance, and reports from industry experts. Analysis focuses on providing a balanced perspective, considering both the advantages and limitations of credit AI.
Key Takeaways:
- Credit AI automates and improves credit scoring.
- It expands access to credit for underserved populations.
- It enhances fraud detection and risk management.
- It streamlines the lending process for efficiency.
- Ethical considerations and data privacy are paramount.
Credit AI: A Deep Dive
Introduction: Credit AI is reshaping the financial landscape by revolutionizing how creditworthiness is assessed and lending decisions are made. Its impact extends far beyond simple automation; it fosters greater financial inclusion, enhances risk management, and optimizes operational efficiency within the lending industry. The core of Credit AI lies in its ability to analyze vast datasets, identify patterns imperceptible to traditional methods, and predict future borrower behavior with remarkable accuracy.
Key Aspects:
- Advanced Algorithms: Credit AI utilizes sophisticated machine learning algorithms, such as neural networks and decision trees, to analyze diverse data points and create predictive models.
- Alternative Data Sources: Beyond traditional credit reports, Credit AI incorporates alternative data sources, including transactional data, social media activity, and online behavior, to create a holistic view of a borrower's creditworthiness.
- Automated Decision-Making: The technology automates significant portions of the lending process, from initial application screening to loan approval and monitoring.
- Enhanced Risk Assessment: Credit AI identifies subtle risk indicators that human analysts might miss, reducing defaults and improving portfolio performance.
Discussion:
Advanced Algorithms: The algorithms powering Credit AI go beyond simple statistical models. Neural networks, capable of identifying complex relationships in data, are particularly effective in assessing credit risk. Decision trees provide a transparent and easily interpretable approach, useful for explaining lending decisions to both borrowers and regulators.
Alternative Data Sources: The integration of alternative data is a game-changer. Transactional data from bank accounts and mobile payment platforms provides insights into spending habits and financial responsibility. Social media analysis, while ethically sensitive, can reveal patterns of behavior indicative of creditworthiness. However, the use of such data must adhere to strict privacy regulations.
Automated Decision-Making: Automation drastically reduces processing time for loan applications. AI-powered systems can quickly assess applications, flag potentially fraudulent activity, and provide immediate pre-approval decisions, enhancing the customer experience.
Enhanced Risk Assessment: Traditional credit scoring models often overlook factors that contribute significantly to credit risk. Credit AI can identify these factors, such as subtle changes in spending patterns or unusual online activity, leading to more accurate risk assessments and reduced loan defaults. This allows lenders to offer more tailored products and pricing, while managing risk more effectively.
Alternative Data Sources in Credit AI
Introduction: The incorporation of alternative data is a defining characteristic of Credit AI, significantly expanding the scope of credit assessment beyond traditional credit reports.
Facets:
- Role: Alternative data provides a more comprehensive view of a borrower's financial behavior and creditworthiness.
- Examples: Bank transaction data, mobile payment data, social media activity, online purchase history, utility payment records.
- Risks: Data privacy concerns, potential for bias in data sources, regulatory compliance challenges.
- Mitigations: Strict adherence to data privacy regulations, rigorous data validation processes, algorithmic fairness audits.
- Impacts: Enhanced accuracy of credit scoring, increased financial inclusion, improved risk management.
- Implications: Changes in lending practices, the need for new regulatory frameworks, potential for increased competition in the lending market.
Summary: The strategic use of alternative data in Credit AI offers the potential to revolutionize the lending industry, providing access to credit for individuals previously excluded from traditional financial systems. However, addressing ethical considerations and regulatory requirements is crucial to ensuring responsible and equitable use of this technology.
Ethical Considerations and Data Privacy in Credit AI
Introduction: The use of AI in credit assessment raises important ethical considerations related to data privacy, algorithmic bias, and fairness. These issues must be carefully addressed to ensure the responsible and equitable development and deployment of Credit AI.
Further Analysis: Algorithmic bias, stemming from biased training data, can lead to discriminatory lending practices. For example, if the training data overrepresents certain demographic groups, the algorithm might unfairly disadvantage others. This bias must be actively mitigated through careful data selection, algorithmic fairness audits, and ongoing monitoring. Data privacy is paramount. Strict adherence to regulations such as GDPR and CCPA is essential to protect borrowers' sensitive personal information. Transparency in how credit AI systems work is also vital, enabling borrowers to understand the factors influencing their credit scores.
Closing: Addressing ethical considerations and ensuring data privacy are crucial for building public trust in Credit AI and fostering its responsible adoption within the financial sector. This requires a collaborative effort from developers, lenders, regulators, and consumer advocates.
FAQ
Introduction: This section addresses frequently asked questions regarding Credit AI.
Questions:
-
Q: How does Credit AI differ from traditional credit scoring? A: Credit AI utilizes more comprehensive data sources and sophisticated algorithms than traditional methods, leading to more accurate risk assessment and improved loan decision-making.
-
Q: What are the benefits of Credit AI for lenders? A: Enhanced risk management, improved operational efficiency, increased profitability, and access to a wider pool of borrowers.
-
Q: What are the benefits for borrowers? A: Increased access to credit, faster application processing, potentially more favorable loan terms.
-
Q: What are the potential risks of Credit AI? A: Algorithmic bias, data privacy concerns, and the potential for misuse of sensitive personal information.
-
Q: How is data privacy protected in Credit AI systems? A: Strict adherence to data privacy regulations, robust security measures, and data anonymization techniques.
-
Q: What is the future of Credit AI? A: Continued advancements in AI algorithms, integration with other FinTech solutions, and increased adoption across the lending industry.
Summary: Addressing common concerns and misconceptions surrounding Credit AI is vital for its successful adoption. Transparency and responsible development are essential for building trust.
Transition: Understanding the potential benefits and challenges of Credit AI requires a balanced perspective, recognizing both its transformative power and its inherent complexities.
Tips for Implementing Credit AI
Introduction: Successfully implementing Credit AI requires careful planning, selection of appropriate technology, and a commitment to ethical considerations.
Tips:
- Data Strategy: Develop a robust data strategy to ensure high-quality, unbiased, and ethically sourced data.
- Algorithm Selection: Choose algorithms appropriate for the specific lending needs and data characteristics.
- Model Validation: Rigorously validate and test models to ensure accuracy and fairness.
- Regulatory Compliance: Adhere to all relevant data privacy and regulatory requirements.
- Transparency and Explainability: Develop systems that provide transparent and easily understandable explanations of credit decisions.
- Ongoing Monitoring: Continuously monitor the performance of Credit AI systems and adapt them as needed.
- Human Oversight: Maintain appropriate human oversight to ensure responsible use of the technology.
Summary: The successful implementation of Credit AI depends on a thoughtful and strategic approach that prioritizes data quality, algorithmic fairness, and regulatory compliance.
Transition: The future of lending is inextricably linked to the continued development and responsible application of Credit AI.
Summary: The Transformative Power of Credit AI
Summary: This exploration of Credit AI has revealed its potential to revolutionize the lending industry, improving access to credit, enhancing risk management, and streamlining operations. However, responsible development and deployment require careful consideration of ethical implications and data privacy concerns.
Closing Message: Credit AI stands poised to reshape the financial landscape. By embracing its capabilities while mitigating its risks, the lending industry can create a more inclusive, efficient, and equitable system that benefits both lenders and borrowers alike. The future of responsible financial innovation hinges on this careful balancing act.
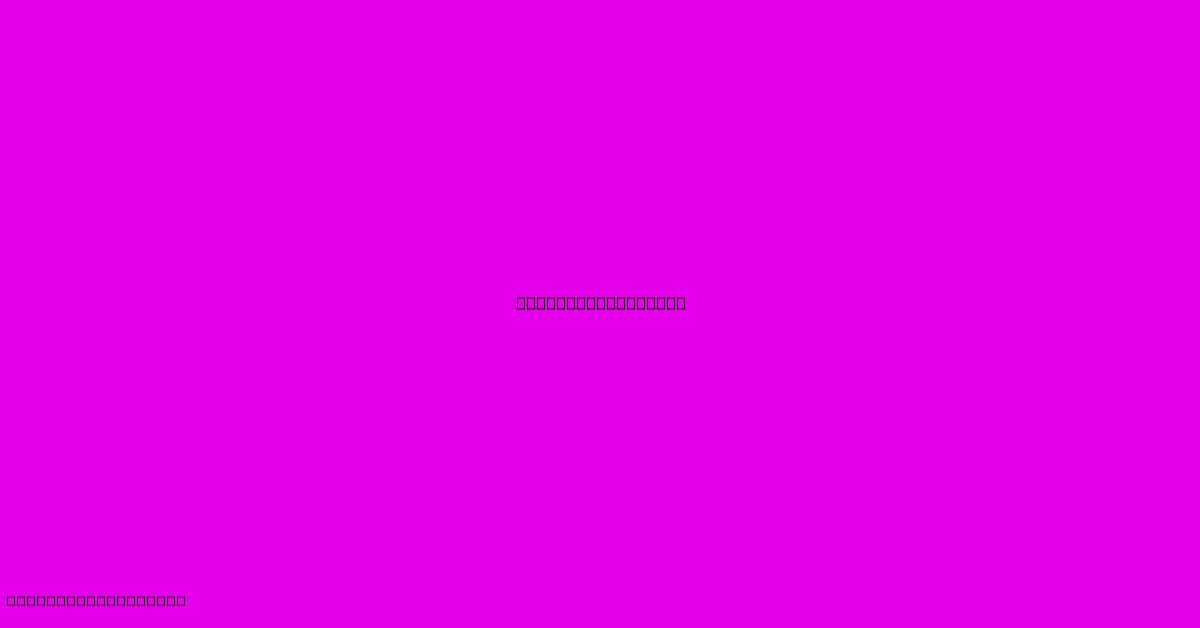
Thank you for taking the time to explore our website What Is Credit Ai. We hope you find the information useful. Feel free to contact us for any questions, and don’t forget to bookmark us for future visits!
We truly appreciate your visit to explore more about What Is Credit Ai. Let us know if you need further assistance. Be sure to bookmark this site and visit us again soon!
Featured Posts
-
Swap Spread Definition Use As An Indicator And Example
Jan 11, 2025
-
What Is A Value Added Reseller Var Definition And Example
Jan 11, 2025
-
Tax Liability Definition Calculation And Example
Jan 11, 2025
-
What Is The Cost Principle In Accounting
Jan 11, 2025
-
Unemployment Income Definition
Jan 11, 2025