What Is Variance In Statistics Definition Formula And Example
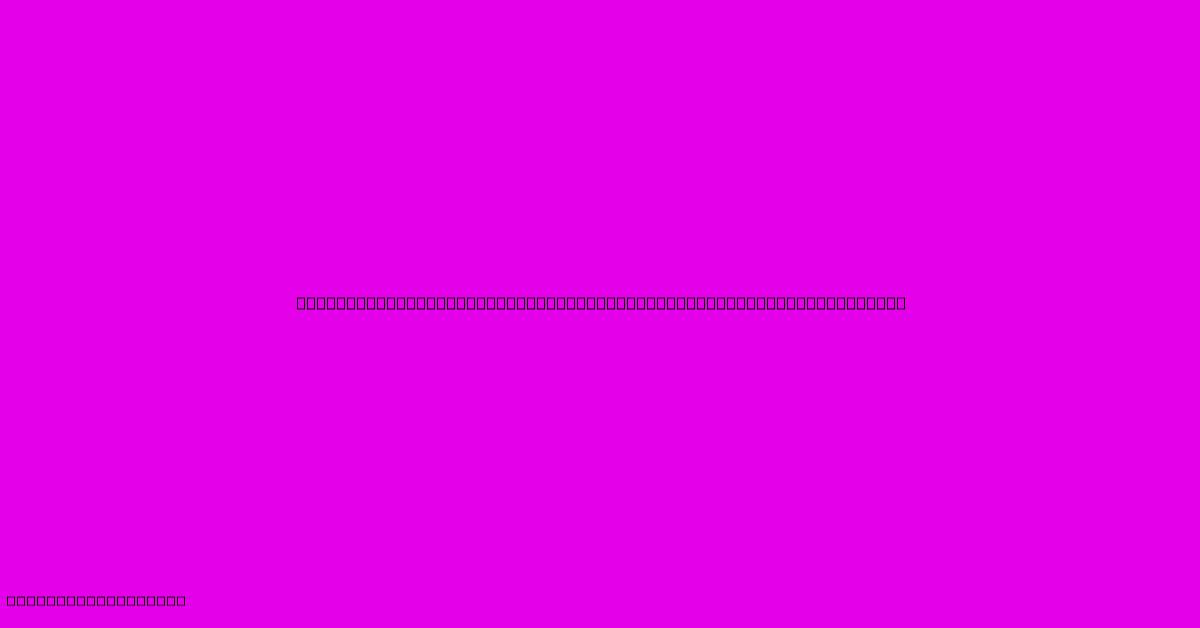
Discover more in-depth information on our site. Click the link below to dive deeper: Visit the Best Website meltwatermedia.ca. Make sure you don’t miss it!
Table of Contents
Unlocking the Power of Variance: Definition, Formula, and Real-World Applications
Hook: Ever wondered how spread out a dataset is? Variance reveals the extent to which data points deviate from the average, offering crucial insights into data variability.
Editor's Note: This comprehensive guide to variance in statistics has been published today, providing a clear understanding of its definition, calculation, and practical applications.
Importance & Summary: Understanding variance is fundamental in statistics. It quantifies the dispersion or spread of a dataset around its mean, providing valuable information for data analysis, forecasting, and decision-making across diverse fields. This guide will cover the definition, formula (both population and sample variance), step-by-step calculations, and real-world examples to illustrate its significance. Key concepts like standard deviation (the square root of variance) and its relationship to variance will also be explored.
Analysis: The information presented in this guide is compiled from established statistical principles and methodologies. Numerous examples across various fields demonstrate the practical application of variance calculations. This approach ensures clarity and practical understanding for the reader.
Key Takeaways:
- Variance measures data dispersion.
- Two formulas exist: one for populations, one for samples.
- Variance is always non-negative.
- Standard deviation is the square root of variance.
- Variance is crucial for various statistical analyses.
Variance: A Measure of Data Dispersion
Variance, in the context of statistics, quantifies the spread or dispersion of a dataset around its mean (average). A higher variance indicates greater variability, meaning data points are more spread out; conversely, a lower variance suggests data points cluster more closely around the mean. This measure provides invaluable insights into the data's characteristics and helps in making informed decisions.
Understanding the Formulas
Two distinct formulas exist for calculating variance: one for the entire population and another for a sample drawn from a larger population. The distinction arises because sample variance is an estimate of the population variance, requiring a slight adjustment to avoid underestimation.
1. Population Variance:
The population variance (σ²) is calculated using the following formula:
σ² = Σ(xi - μ)² / N
Where:
- σ² represents the population variance.
- Σ denotes the sum of.
- xi represents each individual data point in the population.
- μ represents the population mean (average).
- N represents the total number of data points in the population.
2. Sample Variance:
The sample variance (s²) is calculated using the following formula:
s² = Σ(xi - x̄)² / (n - 1)
Where:
- s² represents the sample variance.
- Σ denotes the sum of.
- xi represents each individual data point in the sample.
- x̄ represents the sample mean (average).
- n represents the total number of data points in the sample.
- (n - 1) is the degrees of freedom, used to correct for the bias in sample variance estimation.
The key difference is the denominator. Using (n-1) in the sample variance formula provides a more accurate and unbiased estimate of the population variance, especially when the sample size is small.
Step-by-Step Calculation Example (Sample Variance)
Let's consider a sample of exam scores: {70, 80, 90, 100, 75}. Let's calculate the sample variance:
-
Calculate the mean (x̄): (70 + 80 + 90 + 100 + 75) / 5 = 83
-
Calculate the deviations from the mean (xi - x̄):
- 70 - 83 = -13
- 80 - 83 = -3
- 90 - 83 = 7
- 100 - 83 = 17
- 75 - 83 = -8
-
Square the deviations:
- (-13)² = 169
- (-3)² = 9
- (7)² = 49
- (17)² = 289
- (-8)² = 64
-
Sum the squared deviations: 169 + 9 + 49 + 289 + 64 = 580
-
Divide by (n - 1): 580 / (5 - 1) = 145
Therefore, the sample variance (s²) is 145. This indicates a relatively high degree of variability in the exam scores.
Standard Deviation: The Square Root of Variance
The standard deviation (σ for population, s for sample) is simply the square root of the variance. It's often preferred because it's expressed in the same units as the original data, making it easier to interpret. For the example above, the sample standard deviation is √145 ≈ 12.04. This means the scores deviate from the mean by approximately 12.04 points on average.
Real-World Applications of Variance
Variance finds widespread application across various fields:
- Finance: Assessing the risk of investments. A higher variance in stock returns implies higher risk.
- Quality Control: Monitoring the consistency of production processes. Lower variance indicates higher quality and fewer defects.
- Healthcare: Measuring the variability of patient outcomes. Understanding variance in treatment responses helps optimize medical practices.
- Meteorology: Analyzing weather patterns. Variance can reveal the predictability and consistency of weather phenomena.
- Engineering: Evaluating the reliability of components. Lower variance in component performance signifies greater robustness.
Beyond the Basics: Interpreting Variance
The magnitude of variance itself is not always directly interpretable without context. Comparing the variances of different datasets, or relating variance to the mean (coefficient of variation), provides a more comprehensive understanding of data variability.
FAQ: Variance in Statistics
Introduction: This section addresses frequently asked questions about variance in statistics.
Questions:
-
Q: What does a variance of zero mean? A: A variance of zero indicates that all data points are identical; there is no variability.
-
Q: Can variance be negative? A: No, variance is always non-negative because it involves squaring the deviations from the mean.
-
Q: What is the difference between population and sample variance? A: Population variance uses the entire population data, while sample variance estimates population variance using a subset of data and adjusts for bias.
-
Q: How is variance related to standard deviation? A: Standard deviation is the square root of variance and is easier to interpret as it's in the same units as the original data.
-
Q: Why use (n-1) in the sample variance formula? A: Using (n-1) (degrees of freedom) corrects for the bias inherent in using a sample to estimate the population variance.
-
Q: How can I interpret variance in a real-world context? A: The interpretation depends on the context. In finance, high variance might indicate high risk; in manufacturing, low variance indicates high quality.
Summary: Understanding the nuances of variance calculation and interpretation is crucial for effectively analyzing data across various fields.
Transition: Let's move on to practical tips for working with variance.
Tips for Working with Variance
Introduction: These tips streamline the process of calculating and interpreting variance.
Tips:
-
Use software: Statistical software packages (R, SPSS, Excel) automate variance calculations.
-
Organize your data: A well-organized dataset simplifies calculations.
-
Check your calculations: Double-check your work to avoid errors.
-
Understand the context: The meaning of variance depends heavily on the application and dataset.
-
Consider the standard deviation: Standard deviation often provides a more intuitive understanding of variability.
-
Compare variances: Compare variances across datasets for meaningful insights.
-
Visualize your data: Histograms and box plots help visualize data distribution and variance.
-
Consult resources: Refer to statistical textbooks or online resources for deeper understanding.
Summary: Applying these tips leads to efficient and accurate variance analysis, enhancing data interpretation and decision-making.
Transition: Let's summarize the key findings of this exploration of variance.
Summary of Variance in Statistics
This guide comprehensively explored the concept of variance in statistics, covering its definition, calculation methods (for both populations and samples), practical applications across various fields, and related concepts such as standard deviation. The importance of understanding variance for accurate data analysis and informed decision-making has been emphasized throughout.
Closing Message: Mastering variance is a cornerstone of statistical literacy. By understanding its calculation and interpretation, one can gain valuable insights into data variability and make data-driven decisions with greater confidence. Further exploration of advanced statistical techniques building upon the foundation of variance is encouraged.
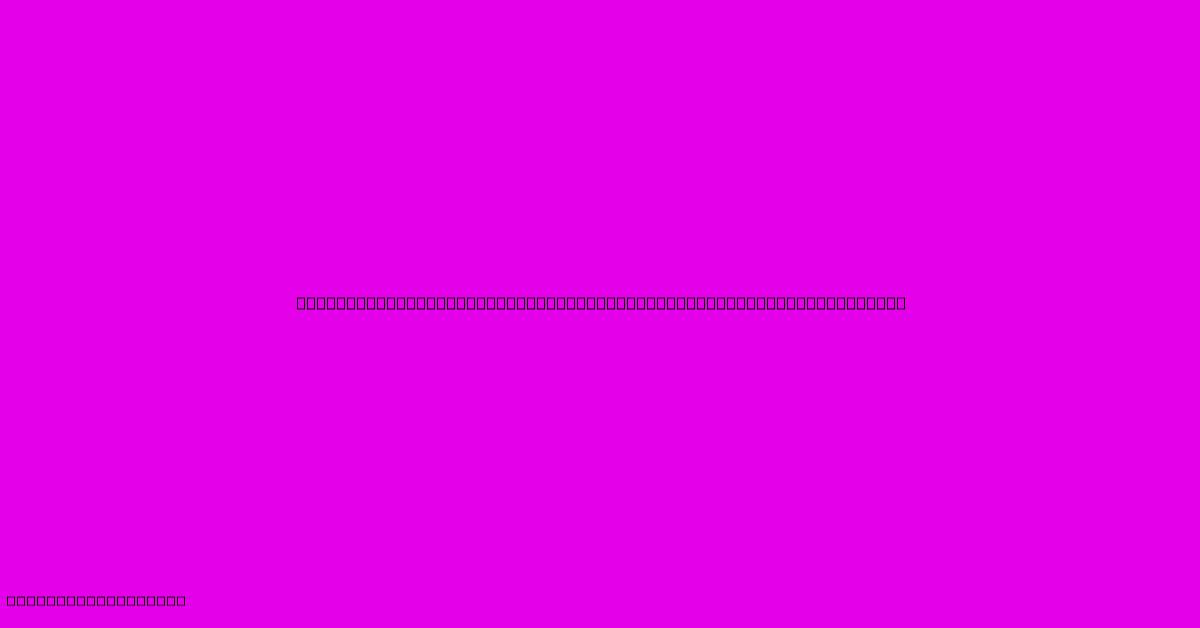
Thank you for taking the time to explore our website What Is Variance In Statistics Definition Formula And Example. We hope you find the information useful. Feel free to contact us for any questions, and don’t forget to bookmark us for future visits!
We truly appreciate your visit to explore more about What Is Variance In Statistics Definition Formula And Example. Let us know if you need further assistance. Be sure to bookmark this site and visit us again soon!
Featured Posts
-
What Is Ppv Accounting
Jan 11, 2025
-
Publicly Traded Company Definition How It Works And Examples
Jan 11, 2025
-
Trade Line Definition How It Works And Included Records
Jan 11, 2025
-
How To Get Cash From Paypal Credit
Jan 11, 2025
-
Swap Curve Definition Comparison To Yield Curve And How To Use
Jan 11, 2025